A deep-learning algorithm with two-stage training for solar forecast post-processing
Solar Energy(2024)
摘要
Post-processing is a common strategy to boost the quality of irradiance forecasts from numerical weather prediction (NWP). This work approaches this problem from a machine learning perspective and proposes a two-stage optimization training method based on an improved multi-layer extreme learning machine (ML-ELM). In the first stage, the standard ELM network is stacked into ML-ELM using the auto-encoder, and the parameters of the network are trained to calculate the weight matrix between the connection layers. In the second stage, the particle swarm optimization algorithm is employed to further improve the network by optimizing the parameters. In the empirical part of this study, the proposed post-processing method is applied to NWP forecasts issued by the European Centre for Medium-Range Weather Forecasts. The “apple-to-apple” comparison, which is exceedingly rare in the current research, shows that the two-stage post-processing method is able to outperform not just previously published results obtained through the kernel conditional density estimation, but also those from two other ELM-based methods.
更多查看译文
关键词
Extreme learning machine,Machine learning,Solar forecasting,Numerical weather prediction,Post-processing
AI 理解论文
溯源树
样例
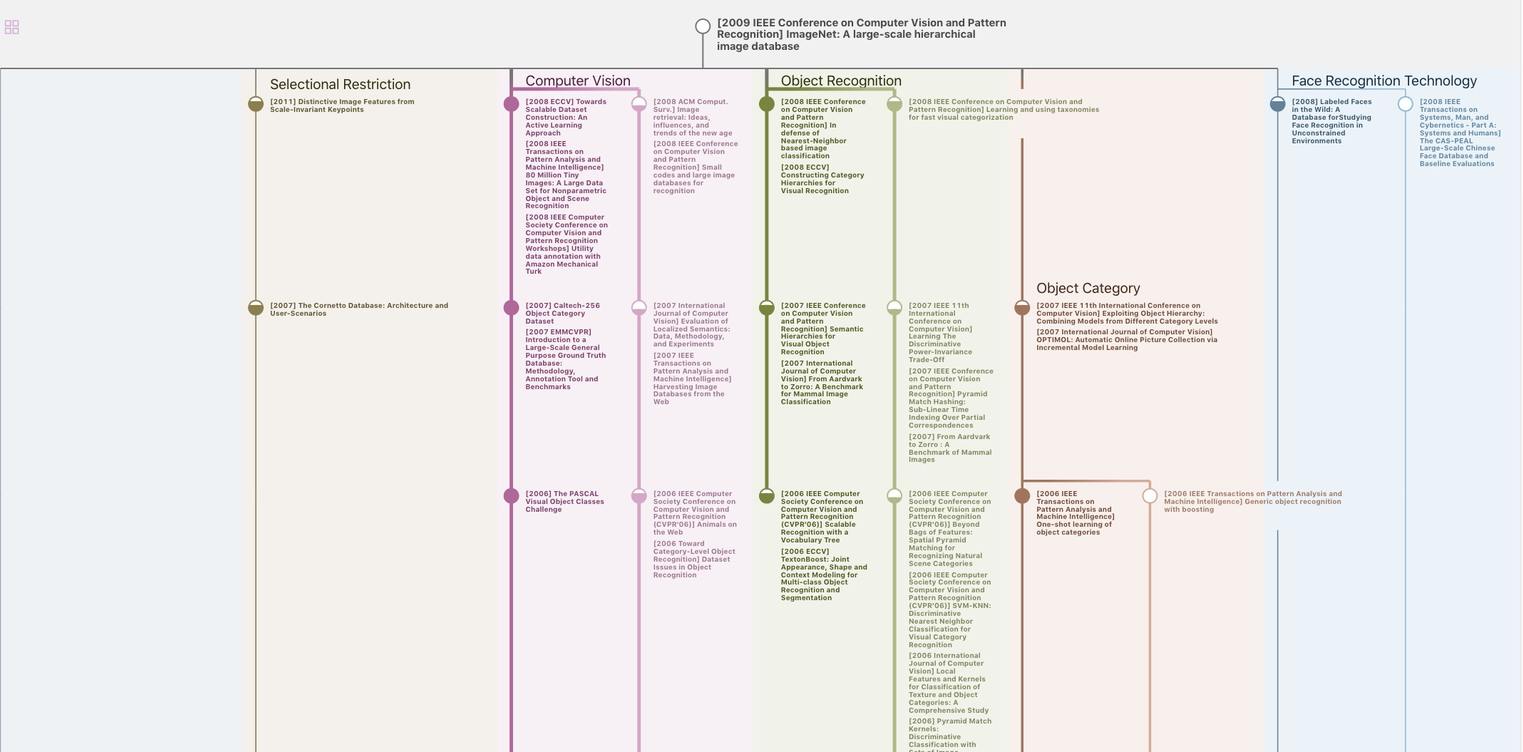
生成溯源树,研究论文发展脉络
Chat Paper
正在生成论文摘要