Learning enhancing modality-invariant features for visible-infrared person re-identification
International Journal of Machine Learning and Cybernetics(2024)
摘要
To solve the task of visible-infrared person re-identification, most existing methods embed all images into a unified feature space through shared parameters, and then use a metric learning loss function to learn modality-invariant features. However, they may encounter the following two problems: For one thing, they mostly focus on modality-invariant features. In reality, some unique features within each modality can enhance feature discriminability but are often overlooked; For another, current metric learning loss functions mainly focus on feature discriminability and only align modality distributions implicitly, which leads to that the feature distributions from different modalities are still inconsistent in this unified feature space. Taking the foregoing into consideration, in this paper, we propose a novel end-to-end framework composed of two modules: the intra-modality enhancing module and the modality-invariant module. The former fully leverages modality-specific characteristics by establishing independent branches for each modality. It improves feature discriminability by further enhancing the intra-class compactness and inter-class discrepancy within each modality. The latter is designed with a cross-modality feature distribution consistency loss based on the Gaussian distribution assumption. It significantly alleviates the modality discrepancies by effectively and directly aligning the feature distribution in the unified feature space. As a result, the proposed framework can learn modality-invariant features with enhancing discriminability in each modality. Extensive experimental results on SYSU-MM01 and RegDB demonstrate the effectiveness of our method.
更多查看译文
关键词
Visible-infrared person re-identification,Cross-modality,Feature learning,Feature distribution
AI 理解论文
溯源树
样例
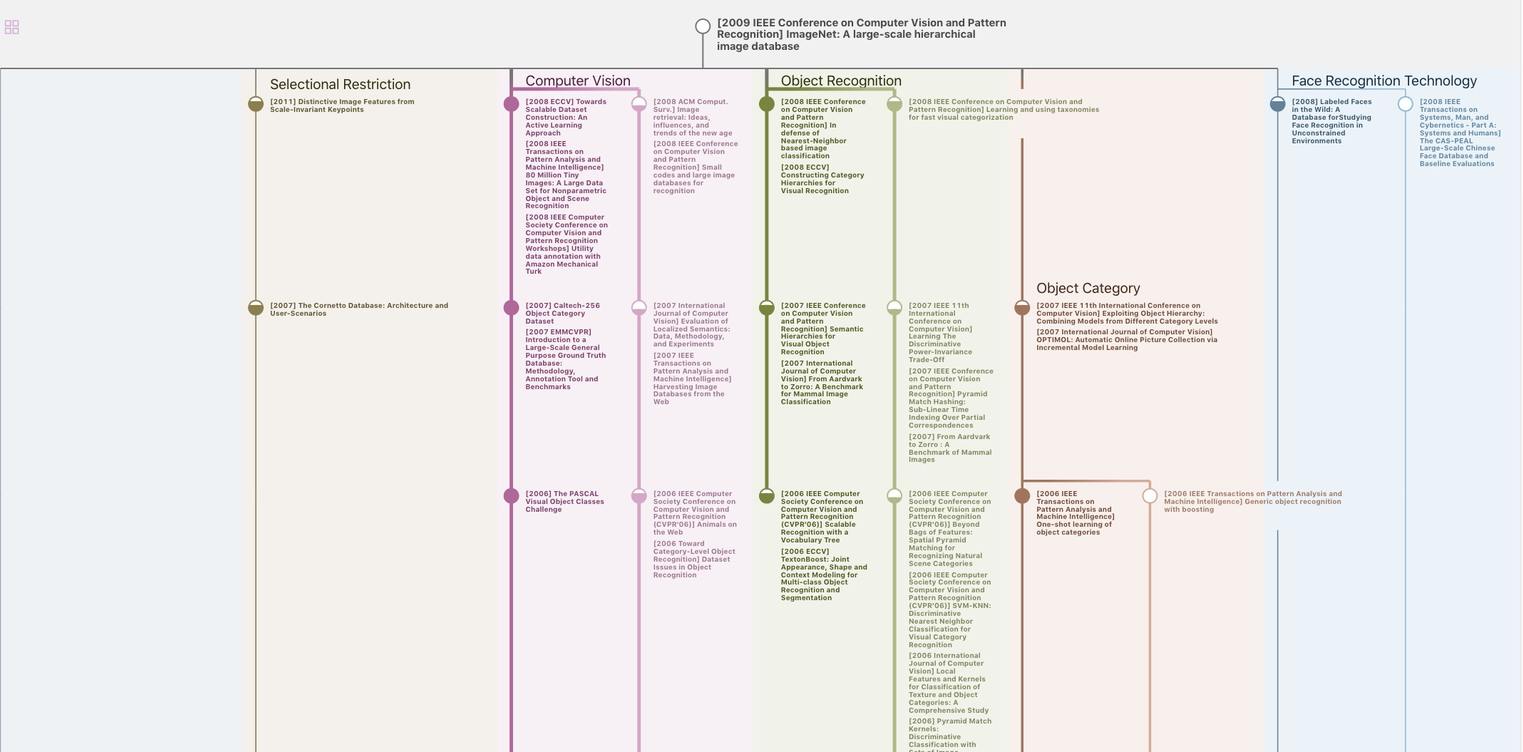
生成溯源树,研究论文发展脉络
Chat Paper
正在生成论文摘要