Mean-field Potts and random-cluster dynamics from high-entropy initializations
arxiv(2024)
摘要
A common obstruction to efficient sampling from high-dimensional
distributions is the multimodality of the target distribution because Markov
chains may get trapped far from stationarity. Still, one hopes that this is
only a barrier to the mixing of Markov chains from worst-case initializations
and can be overcome by choosing high-entropy initializations, e.g., a product
or weakly correlated distribution. Ideally, from such initializations, the
dynamics would escape from the saddle points separating modes quickly and
spread its mass between the dominant modes.
In this paper, we study convergence from high-entropy initializations for the
random-cluster and Potts models on the complete graph – two extensively
studied high-dimensional landscapes that pose many complexities like
discontinuous phase transitions and asymmetric metastable modes. We study the
Chayes–Machta and Swendsen–Wang dynamics for the mean-field random-cluster
model and the Glauber dynamics for the Potts model. We sharply characterize the
set of product measure initializations from which these Markov chains mix
rapidly, even though their mixing times from worst-case initializations are
exponentially slow. Our proofs require careful approximations of projections of
high-dimensional Markov chains (which are not themselves Markovian) by
tractable 1-dimensional random processes, followed by analysis of the latter's
escape from saddle points separating stable modes.
更多查看译文
AI 理解论文
溯源树
样例
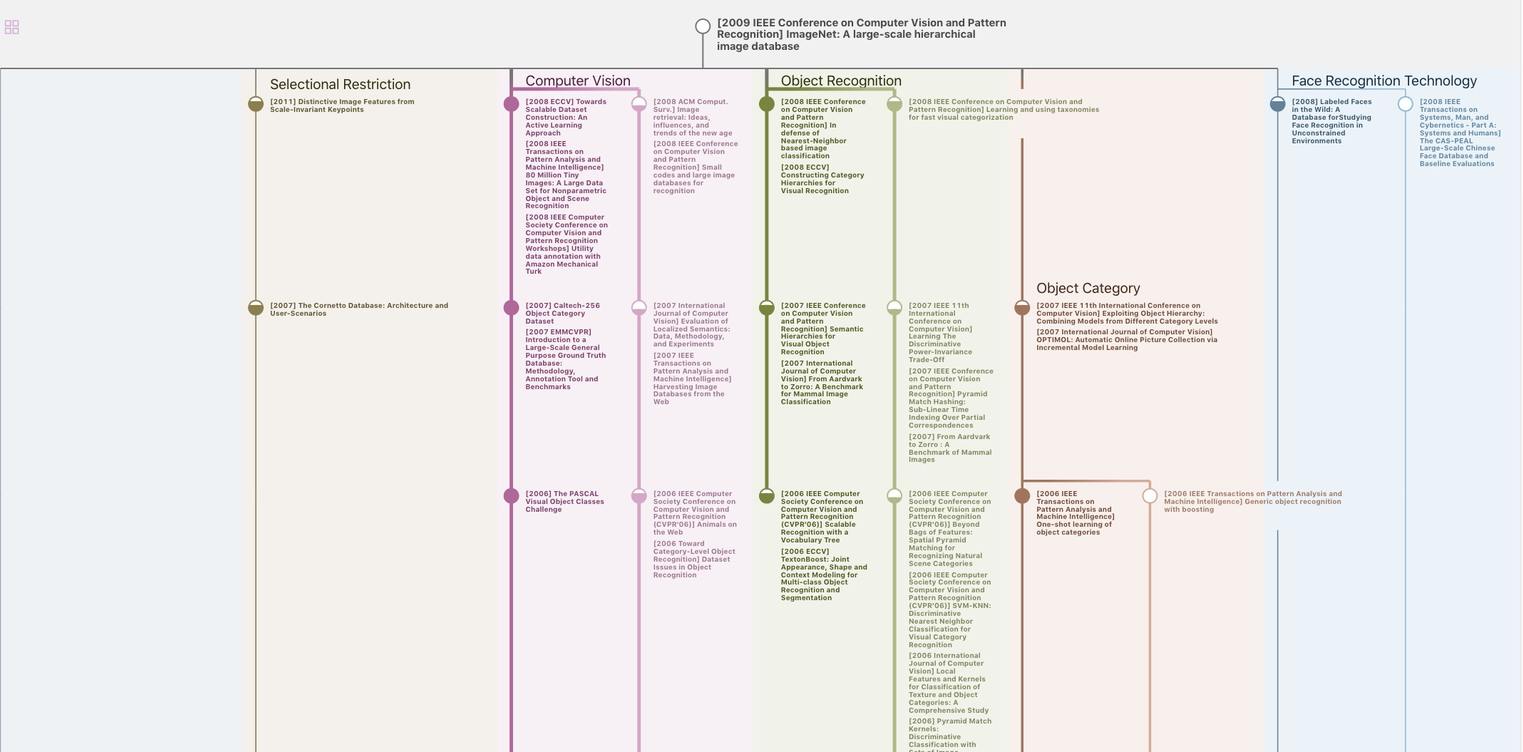
生成溯源树,研究论文发展脉络
Chat Paper
正在生成论文摘要