Towards Universal Performance Modeling for Machine Learning Training on Multi-GPU Platforms
arxiv(2024)
摘要
Characterizing and predicting the training performance of modern machine
learning (ML) workloads on compute systems with compute and communication
spread between CPUs, GPUs, and network devices is not only the key to
optimization and planning but also a complex goal to achieve. The primary
challenges include the complexity of synchronization and load balancing between
CPUs and GPUs, the variance in input data distribution, and the use of
different communication devices and topologies (e.g., NVLink, PCIe, network
cards) that connect multiple compute devices, coupled with the desire for
flexible training configurations. Built on top of our prior work for single-GPU
platforms, we address these challenges and enable multi-GPU performance
modeling by incorporating (1) data-distribution-aware performance models for
embedding table lookup, and (2) data movement prediction of communication
collectives, into our upgraded performance modeling pipeline equipped with
inter-and intra-rank synchronization for ML workloads trained on multi-GPU
platforms. Beyond accurately predicting the per-iteration training time of DLRM
models with random configurations with a geomean error of 5.21
multi-GPU platforms, our prediction pipeline generalizes well to other types of
ML workloads, such as Transformer-based NLP models with a geomean error of
3.00
hardware, it is capable of generating insights such as quickly selecting the
fastest embedding table sharding configuration (with a success rate of 85
更多查看译文
AI 理解论文
溯源树
样例
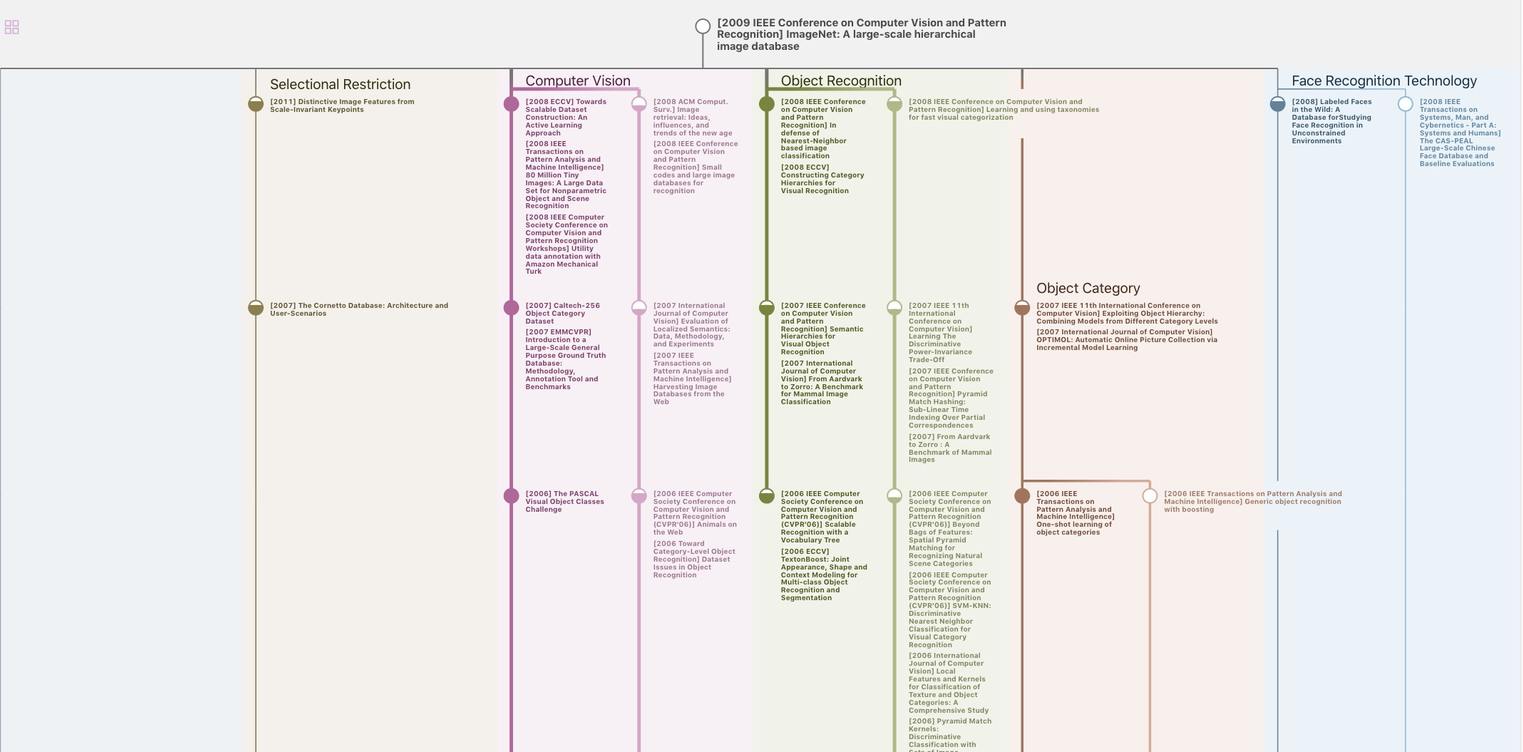
生成溯源树,研究论文发展脉络
Chat Paper
正在生成论文摘要