Memory-Adaptive Vision-and-Language Navigation
Pattern Recognition(2024)
摘要
Vision-and-Language Navigation (VLN) requests an agent to navigate in 3D environments following given instructions, where history is critical for decision-making in dynamic navigation process. Particularly, a memory bank storing histories is widely used in existing methods to incorporate with multimodel representations in current scenes for better decision-making. However, by weighting each history with a simple scalar, those methods cannot purely utilize the informative cues that co-exist with detrimental contents in each history, thereby inevitably introducing noises into decision-making. To that end, we propose a novel Memory-Adaptive Model (MAM) that can dynamically restrain the detrimental contents in histories for retaining contents that benefit navigation only. Specifically, two key modules, Visual and Textual Adaptive Modules, are designed to restrain history noises based on scene-related vision and text, respectively. A Reliability Estimator Module is further introduced to refine above adaptation operations. Our experiments on the widely used RxR and R2R datasets show that MAM outperforms its baseline method by 4.0%/2.5% and 2%/1% on the validation unseen/test split, respectively, wrt the SR metric.
更多查看译文
关键词
Vision-and-Language Navigation,Memory bank,History noises,Memory-Adaptive Model
AI 理解论文
溯源树
样例
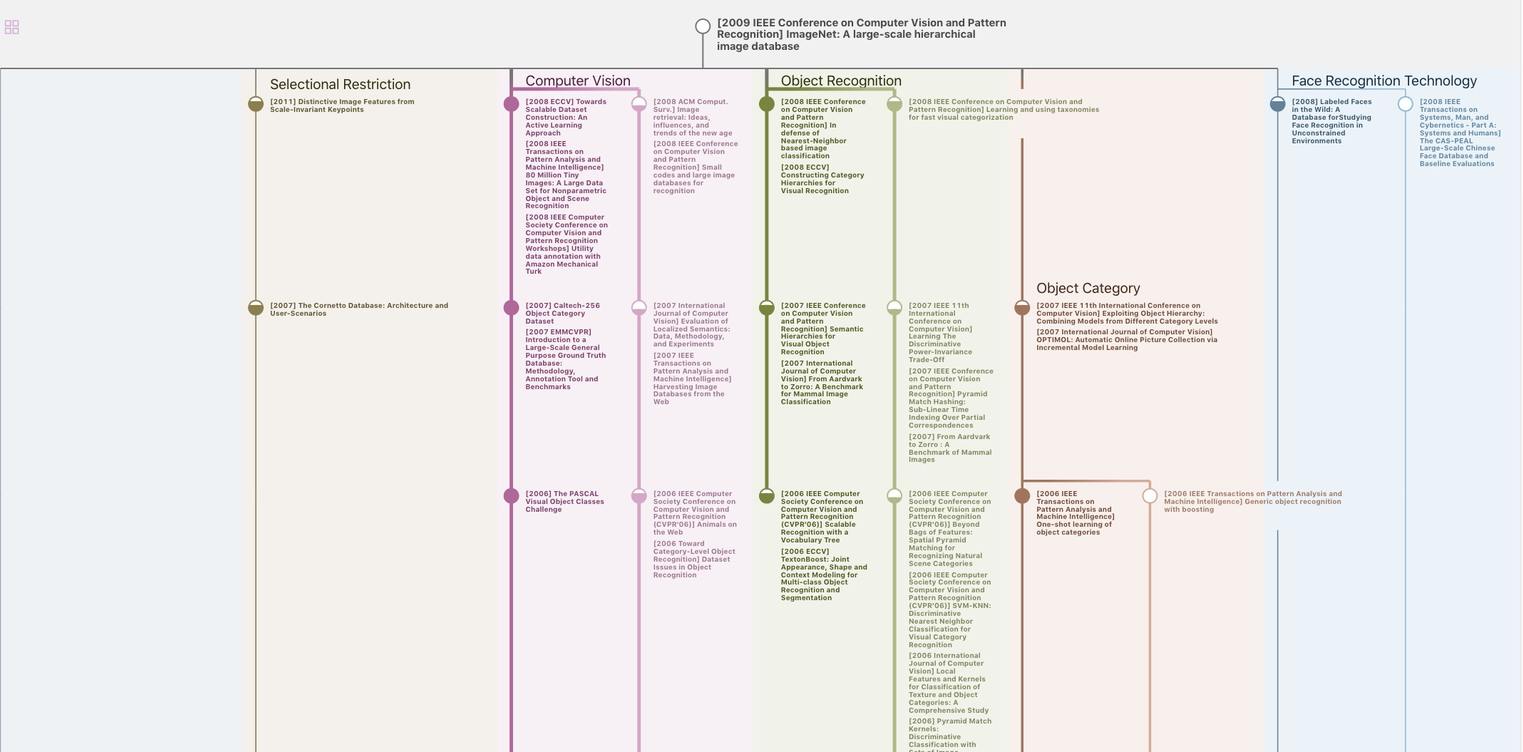
生成溯源树,研究论文发展脉络
Chat Paper
正在生成论文摘要