Deep reinforcement learning framework for end-to-end semiconductor process control
Neural Computing and Applications(2024)
摘要
This work focuses on bringing state-of-the-art artificial intelligence and deep reinforcement learning to the manufacturing of semiconductor devices. The main goal of this research is to lay down the foundation of an end-to-end system for manufacturing control. In contrast to most of the research published in this field, the goal of this algorithm is not to conceptualize the different processes as separate entities but rather to try to control the chain of semiconductor processes as a whole. Therefore, we treat the process chain as an ecosystem where we control all processes using agents that learn to cooperate to produce the best wafers and semiconductor devices. In order to achieve that, we developed an architecture called an ensemble of independent preprocessors (EIP). We applied it with the deep reinforcement learning technique called soft actor-critic (SAC) in various environments, including environments comporting simulated semiconductor devices. The introduced technique strongly outperformed standard statistical methods and allowed us to find new sets of optimal manufacturing parameters different from the true ones. We hope that this work and the presentation of our framework will encourage practitioners to experiment with such systems and make further advancements in the field of decision-making for semiconductor manufacturing.
更多查看译文
关键词
Artificial intelligence,Semiconductor manufacturing,Reinforcement learning,End-to-end system
AI 理解论文
溯源树
样例
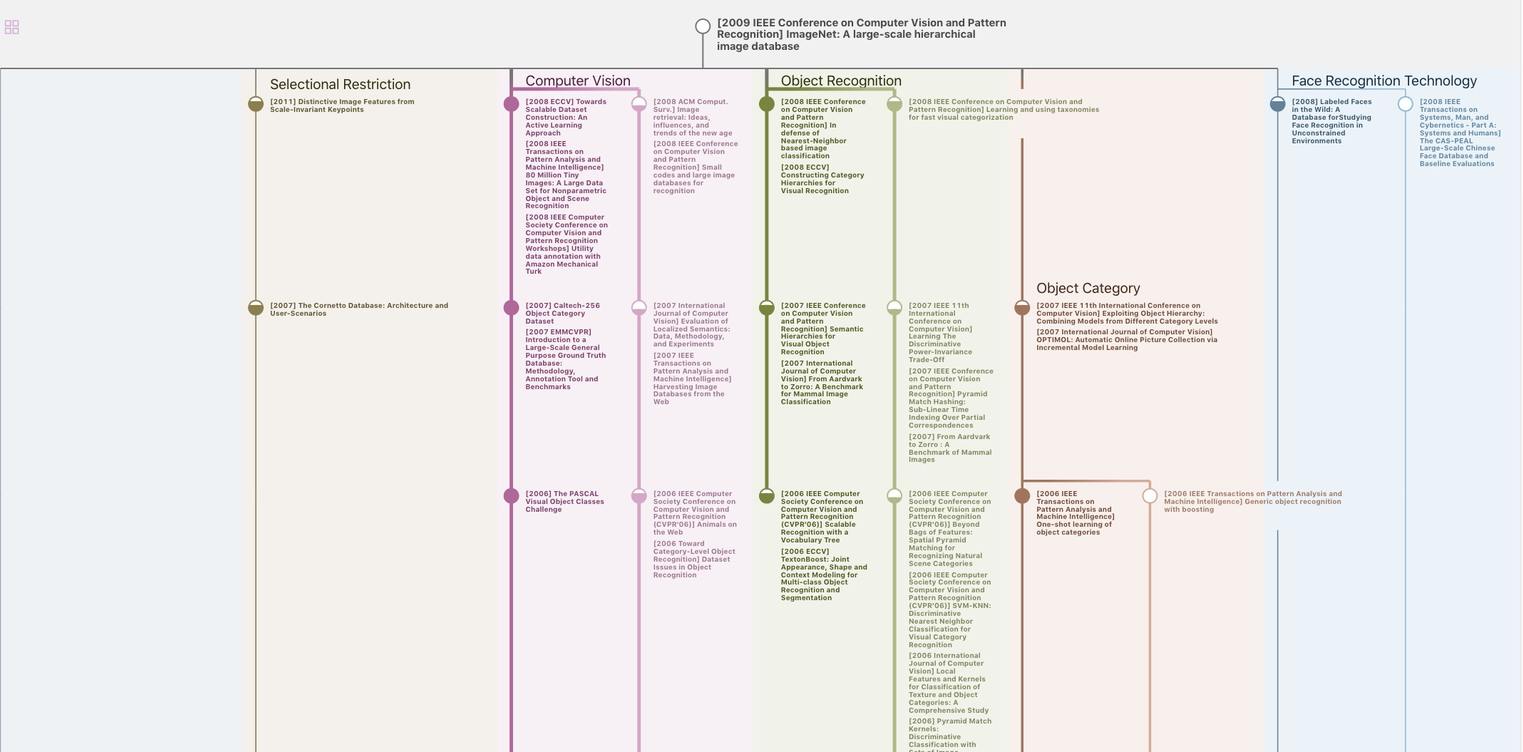
生成溯源树,研究论文发展脉络
Chat Paper
正在生成论文摘要