Exploiting Duality in Aspect Sentiment Triplet Extraction with Sequential Prompting
IEEE Transactions on Knowledge and Data Engineering(2024)
摘要
Aspect sentiment triplet extraction is an important task in natural language processing. Previous work tends to focus on the interaction between the aspect and opinion, while ignoring the positive impact of sentiment on interaction within the triplet. In this paper, we propose a novel aspect sentiment triplet extraction model based on dual learning with sequential prompting. This model is designed as a bidirectional extraction framework that fully takes sentiment polarity into account in the interaction process of aspect and opinion. Besides, we introduce a dual loss as a regularization term for the extraction model to promote better learning in both directions. We further design a sequential prompting strategy to determine aspect, opinion, and sentiment polarity more accurately, which utilizes the results extracted in the previous step as prior knowledge to guide the prediction of the next target. We conduct experiments on three public datasets and the results show the effectiveness of our method. More importantly, we deploy our method on Fliggy application and the 14-day online A/B testing indicates that Page View Click-Through Rate and Page View Conversion Rate increase by 1.17% and 1.08% when user short reviews are used for tagging items with the help of our method.
更多查看译文
关键词
Aspect sentiment triplet extraction,Dual learning,Sequential prompting
AI 理解论文
溯源树
样例
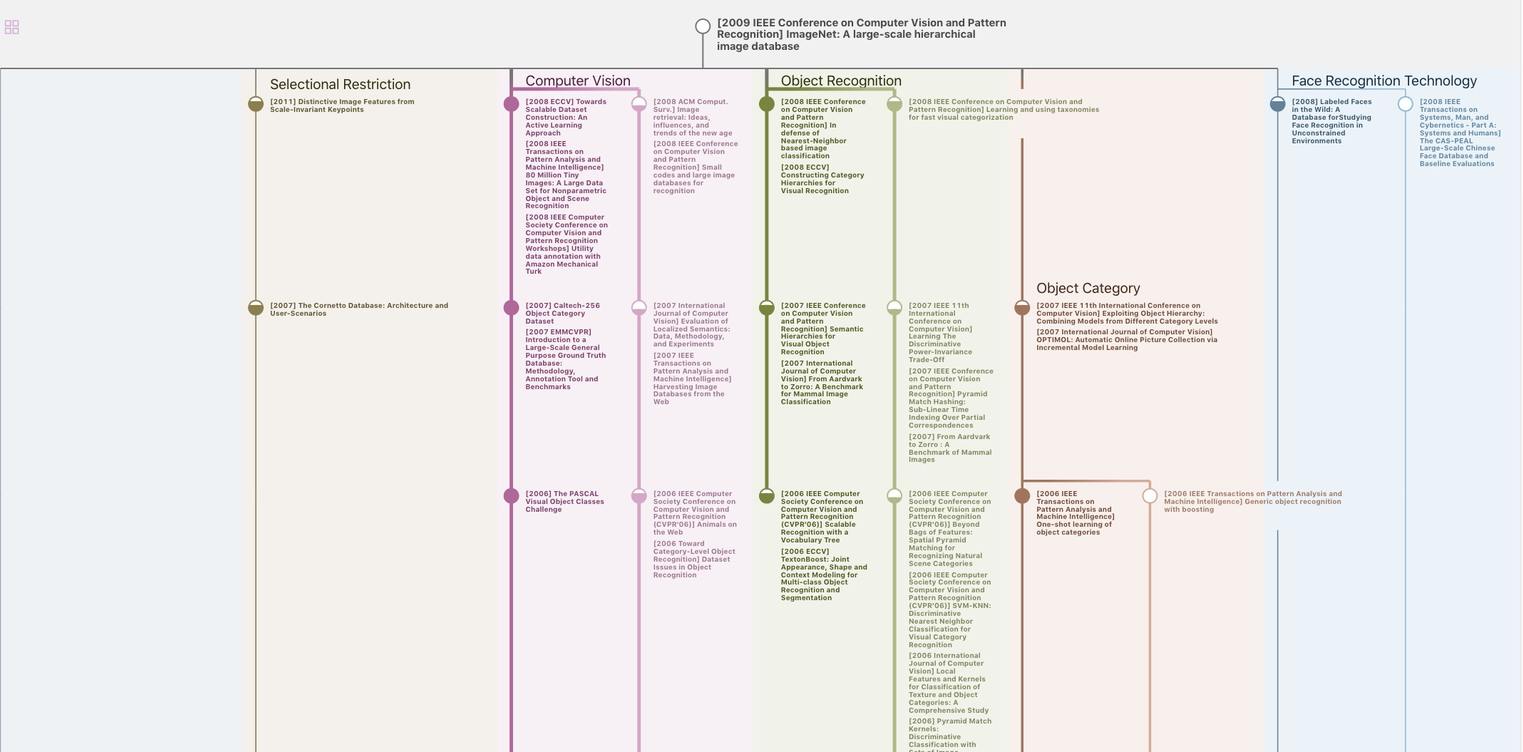
生成溯源树,研究论文发展脉络
Chat Paper
正在生成论文摘要