Automated Texture Feature Based Bone Tumor Segmentation and Image Analysis Using Supervised Machine Learning
2024 International Conference on Computer, Electrical & Communication Engineering (ICCECE)(2024)
摘要
Manual demarcation of tumors in MR Images is time consuming, hard to reproduce and prone to intra rater variability. Accurate tumor demarcation is required for diagnosis and prognosis of tumors and in quantitative image analysis techniques like Apparent Diffusion Coefficients (ADC) using diffusion weighted (DW) MRI. In this study, fully automated machine learning algorithms were developed using textural features extracted from multimodal MRI. A total of 20 patients (n=20; Male:Female=16:4; Age=15.5±2.6 years) with biopsy proven osteosarcoma were analyzed. Multimodal MRI dataset including T1, T2 and proton density weighted MRI and DW-MRI were acquired before treatment using 1.5 T Phillips Achieva MRI scanner. 15 textural features were extracted using Grey-level co-occurrence matrices and Grey-level run length matrices in tumor volume from multimodal MRI. Four fully automated supervised machine learning algorithms 1) Logistic Regression, 2) Linear Support Vector Machines (L-SVMs), 3) Random Forests, and 4) Gradient Boosted D-Trees (GBDTs) were implemented and tested using accuracy metrics as dice coefficient (DC), precision, recall and accuracy. The ADC values calculated in manually demarked and segmented tumor volume were compared for clinical reliability. Logistic Regression demonstrated the best performance (DC: 0.70±0.12; precision: 0.72±0.18; recall: 0.74±0.19; accuracy: 0.98±0.02); While Random forest and GBDTs showed comparable performance (DC: 0.68±0.14, 0.68±0.14; precision: 0.73±0.19, 0.71±0.20; recall: 0.73±0.22, 0.75±0.22; accuracy: 0.98±0.02, 0.98±0.02 respectively); and L-SVM showed comparatively inferior performance (DC: 0.61±0.16; precision: 0.63±0.20; recall: 0.74±0.24; accuracy: 0.97±0.03) than the other methods. The masks segmented by four methods have ADC values ((1.17–1.21)x10
−3
mm
2
/sec) similar to those of manually demarcated masks (1.27×10
−3
mm
2
/sec) and can be used for tumor assessment in clinical settings. Therefore, proposed segmentation methods showed clinically acceptable accuracies and considerable reduction of manual effort involved.
更多查看译文
关键词
Medical image segmentation,Osteosarcoma,Bone tumor segmentation,Machine learning segmentation method,Multimodal MRI
AI 理解论文
溯源树
样例
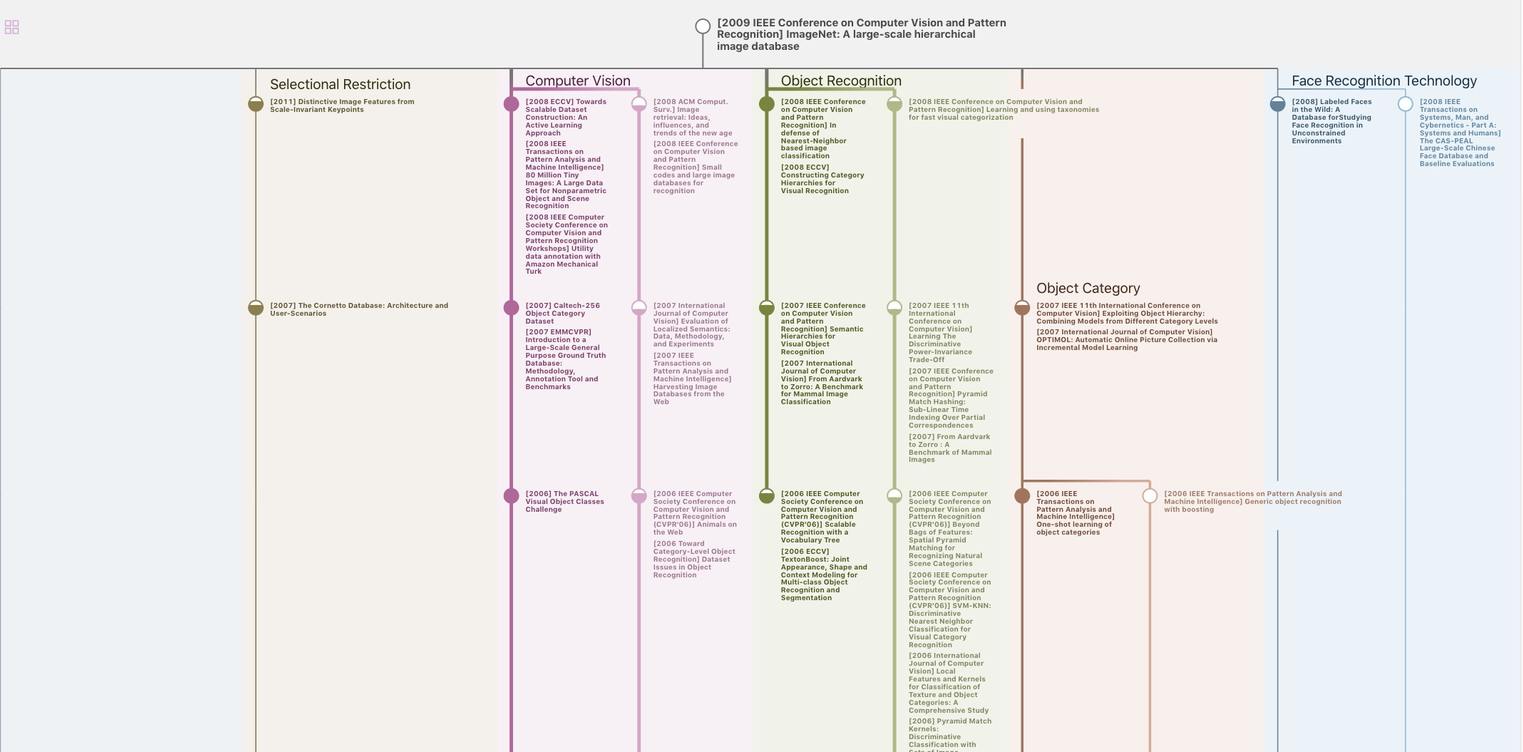
生成溯源树,研究论文发展脉络
Chat Paper
正在生成论文摘要