Spatial-Spectral Contrastive Self-Supervised Learning with Dual Path Networks for Hyperspectral Target Detection
IEEE Transactions on Geoscience and Remote Sensing(2024)
摘要
Deep learning has shown great success in hyperspectral target detection (HTD). Due to the limited targets of interest in hyperspectral image (HSI), many deep learning based methods focus on spectral feature extraction by expanding samples, exhibiting low model transferability as well as high time consumption. To address these issues, we propose a novel spatial-spectral contrastive self-supervised learning-based HTD method with dual path networks (DPN-CSSTD). This method constructs a contrastive task using a HSI augmentation method and a DPN encoder on a large amount of unlabeled data, enabling the pretrained model with spatial-spectral discrimination ability. The DPN encoder utilizes the convolution neural network and the skip connections to improve the spatial-spectral feature extraction. Additionally, the proposed method constructs a transfer learning model with the pretrained DPN encoder and a newly added detector to improve the pretrained model transferability and the time efficiency of target detection tasks. The transfer learning model is fine-tuned via a proposed fine-tuning strategy with few selected samples to adapt to different TD tasks. Extensive experiments conducted on four challenging hyperspectral datasets demonstrate that the proposed method outperforms other state-of-the-art approaches.
更多查看译文
关键词
Hyperspectral target detection,contrastive learning,self-supervised learning,CNN,SLIC segmentation
AI 理解论文
溯源树
样例
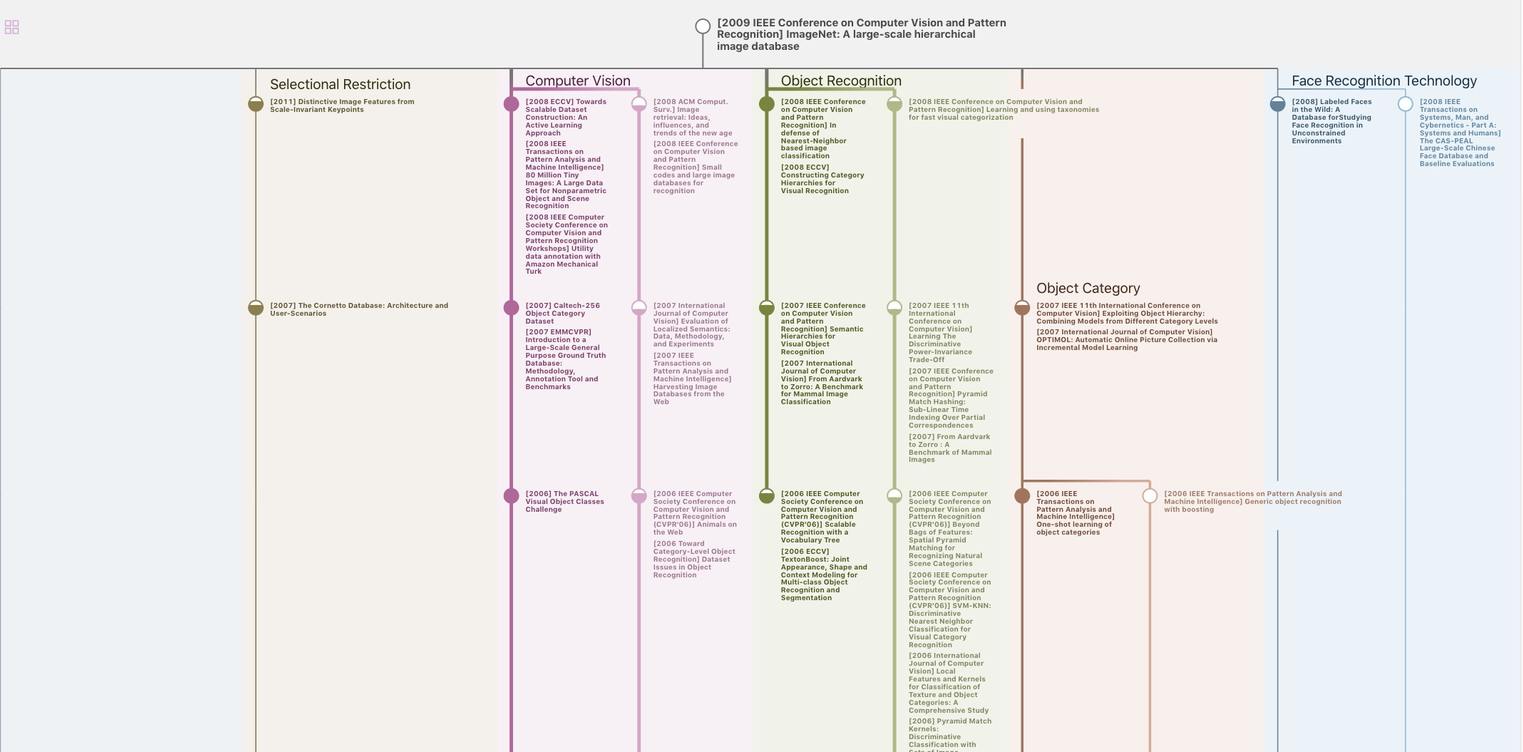
生成溯源树,研究论文发展脉络
Chat Paper
正在生成论文摘要