Efficiently Tackling Million-Dimensional Multiobjective Problems: A Direction Sampling and Fine-Tuning Approach
IEEE Transactions on Emerging Topics in Computational Intelligence(2024)
摘要
We define very large-scale multiobjective optimization problems as optimizing multiple objectives (VLSMOPs) with more than 100,000 decision variables. These problems hold substantial significance, given the ubiquity of real-world scenarios necessitating the optimization of hundreds of thousands, if not millions, of variables. However, the larger dimension in VLSMOPs intensifies the curse of dimensionality and poses significant challenges for existing large-scale evolutionary multiobjective algorithms, rendering them more difficult to solve within the constraints of practical computing resources. To overcome this issue, we propose a novel approach called the very large-scale multiobjective optimization framework (VMOF). The method efficiently samples general yet suitable evolutionary directions in the very large-scale space and subsequently fine-tunes these directions to locate the Pareto-optimal solutions. To sample the most suitable evolutionary directions for different solutions, Thompson sampling is adopted for its effectiveness in recommending from a very large number of items within limited historical evaluations. Furthermore, a technique is designed for fine-tuning directions specific to tracking Pareto-optimal solutions. To understand the designed framework, we present our analysis of the framework and then evaluate VMOF using widely recognized benchmarks and real-world problems spanning dimensions from 100 to 1,000,000. Experimental results demonstrate that our method exhibits superior performance not only on LSMOPs but also on VLSMOPs when compared to existing algorithms.
更多查看译文
关键词
Evolutionary algorithm,multiobjective optimization,large-scale optimization
AI 理解论文
溯源树
样例
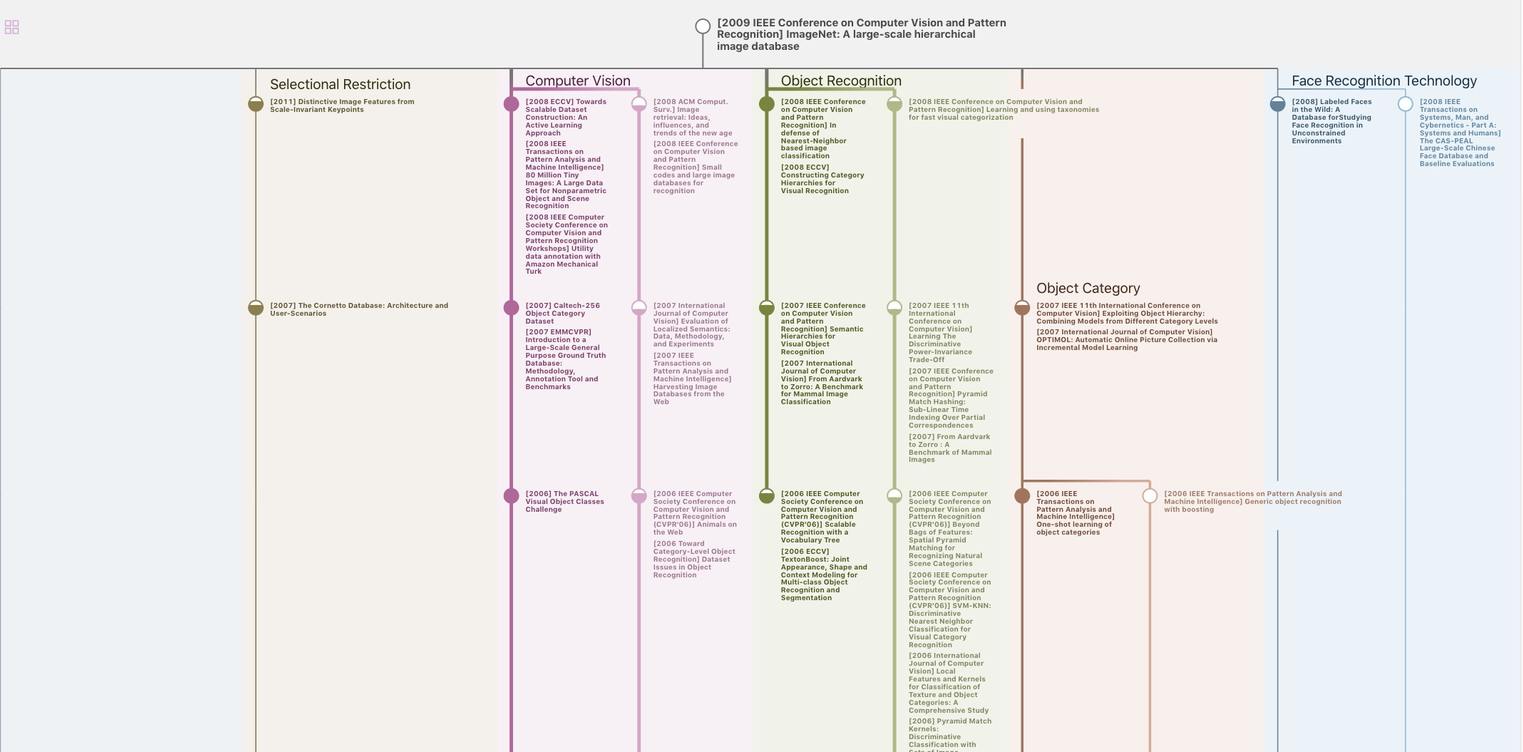
生成溯源树,研究论文发展脉络
Chat Paper
正在生成论文摘要