A Data-Driven Approach for EV Electricity Demand Modeling Using Spatial Regression: A UAE Case Study
IEEE Access(2024)
摘要
The growing global interest in developing environment-friendly and sustainable transportation solutions is motivating mass adoption of Electric Vehicles (EVs). This increasing EV penetration is anticipated to result in a growing electricity demand to address the EV charging requirements. Therefore, precise demand modeling is essential to enable optimal sizing of the electricity generation and distribution networks as well as optimal placement of the EV charging infrastructure. Furthermore, microscopic modeling of EV traffic patterns and trip-wise energy requirements is essential to enable effective charging coordination and demand distribution for on-the-move EVs. However, microscopic EV demand modeling is typically hindered by the scarcity of open-access data that integrates EV charging and driving patterns. Accordingly, this work proposes a methodology for microscopic modeling of the trip-wise electricity demand of mobile EVs in the spatial and temporal domains, using both multiple linear regression and spatial autoregressive models. Secondary open-access data is extracted, wrangled, and pre-processed from a number of data sources to test and validate the proposed methodology on a case study of Dubai – UAE, acknowledging the growing EV adoption rates in the city. The proposed models are benchmarked against baseline models to confirm their superior performance.
更多查看译文
关键词
Autoregressive models,demand modeling,electric vehicles,microscopic demand,multiple linear regression,spatial regression
AI 理解论文
溯源树
样例
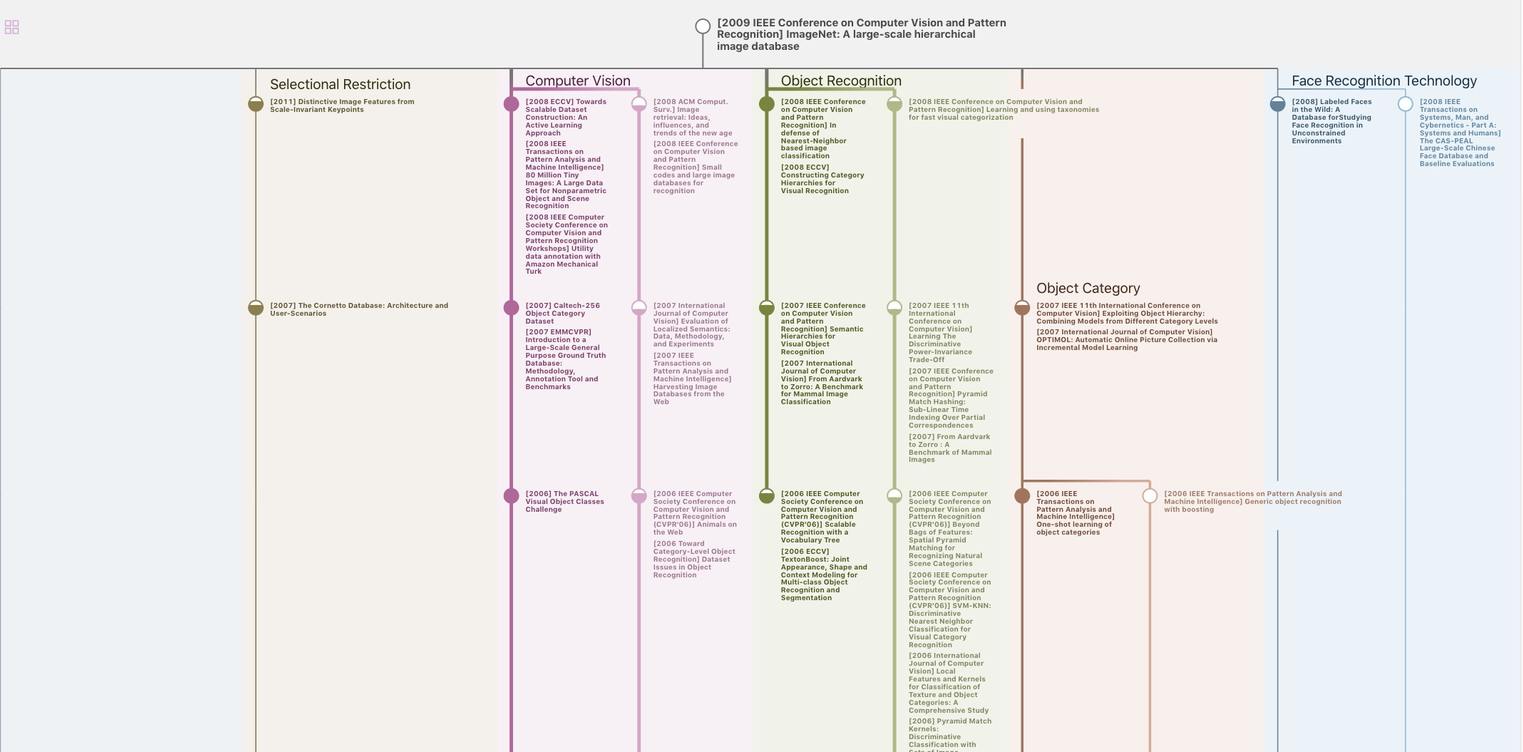
生成溯源树,研究论文发展脉络
Chat Paper
正在生成论文摘要