Dynamic image super-resolution via progressive contrastive self-distillation
Pattern Recognition(2024)
摘要
Convolutional neural networks (CNNs) are highly successful for image super-resolution (SR). However, they often require sophisticated architectures with high memory cost and computational overhead, significantly restricting their practical deployments on resource-limited devices. In this paper, we propose a novel dynamic contrastive self-distillation (Dynamic-CSD) framework to simultaneously compress and accelerate various off-the-shelf SR models, and explore using the trained model for dynamic inference. In particular, to build a compact student network, a channel-splitting super-resolution network (CSSR-Net) can first be constructed from a target teacher network. Then, we propose a novel contrastive loss to improve the quality of SR images via explicit knowledge transfer. Furthermore, progressive CSD (Pro-CSD) is developed to extend the two-branch CSSR-Net into multi-branch, leading to a switchable model at runtime. Finally, a difficulty-aware branch selection strategy for dynamic inference is given. Extensive experiments demonstrate that the proposed Dynamic-CSD scheme effectively compresses and accelerates several standard SR models such as EDSR, RCAN and CARN.
更多查看译文
关键词
Single image super-resolution,Model compression,Model acceleration,Dynamic neural networks
AI 理解论文
溯源树
样例
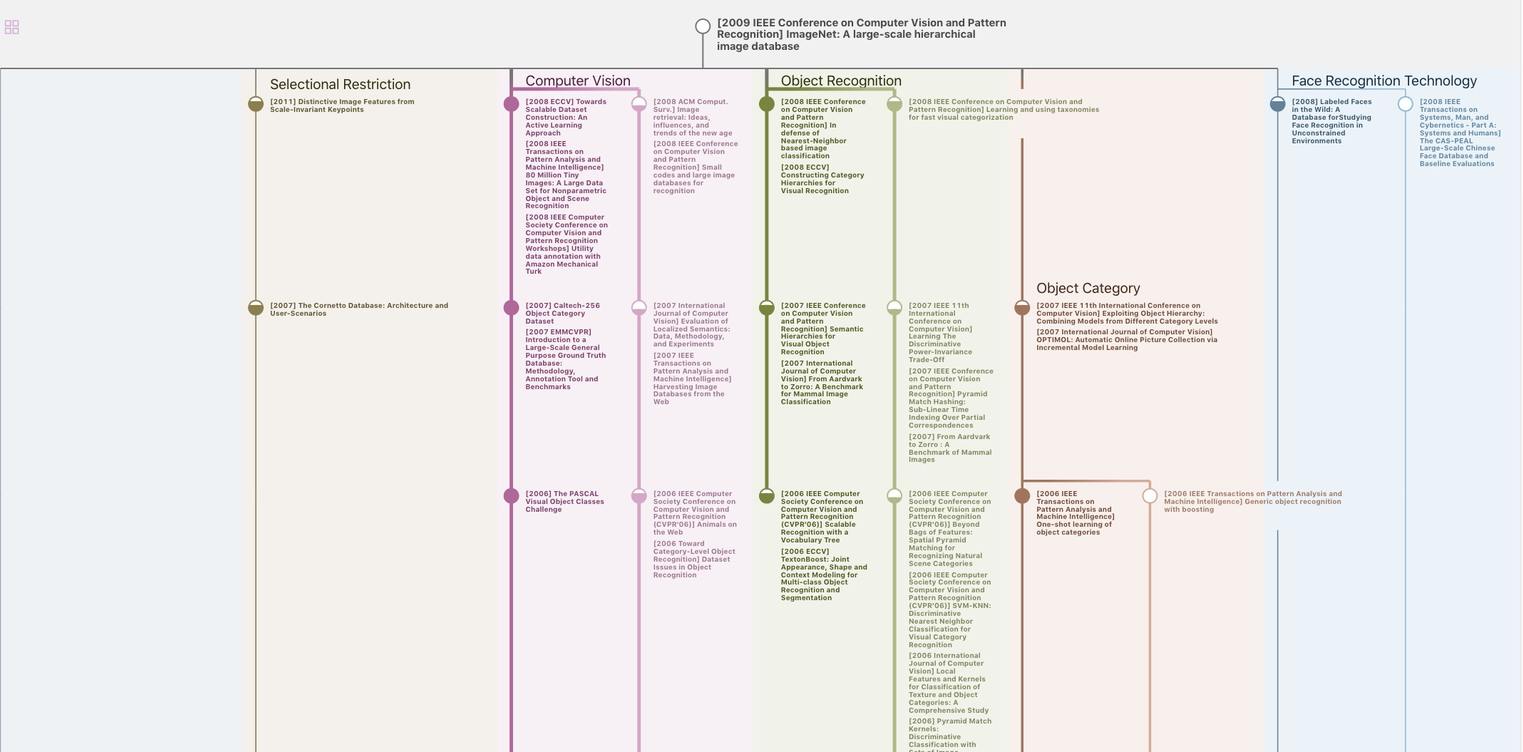
生成溯源树,研究论文发展脉络
Chat Paper
正在生成论文摘要