Explainability of CNN-based Alzheimer’s Disease Detection from Online Handwriting
crossref(2024)
摘要
Abstract With over 55 million people globally affected by dementia and nearly 10 million new cases reported annually, Alzheimer’s disease is a prevalent and challenging neurodegenerative disorder. Despite significant advancements in machine learning techniques for Alzheimer’s disease detection, the widespread adoption of deep learning models raises concerns about their explainability. The lack of explainability in deep learning models for online handwriting analysis is a critical gap in the literature in the context of Alzheimer’s disease detection. This paper addresses this challenge by interpreting predictions from a Convolutional Neural Network applied to multivariate time series data, generated by online handwriting data associated with continuous loop series handwritten on a graphical tablet. Our explainability methods reveal distinct motor behavior characteristics for healthy individuals and those diagnosed with Alzheimer’s. This emphasizes the critical role of explainability in translating complex models into clinically relevant insights. Our research contributes to the enhancement of early diagnosis, providing significant and reliable insights to stakeholders involved in patient care and intervention strategies. Our work bridges the gap between machine learning predictions and clinical insights, fostering a more effective and understandable application of advanced models for Alzheimer’s disease assessment.
更多查看译文
AI 理解论文
溯源树
样例
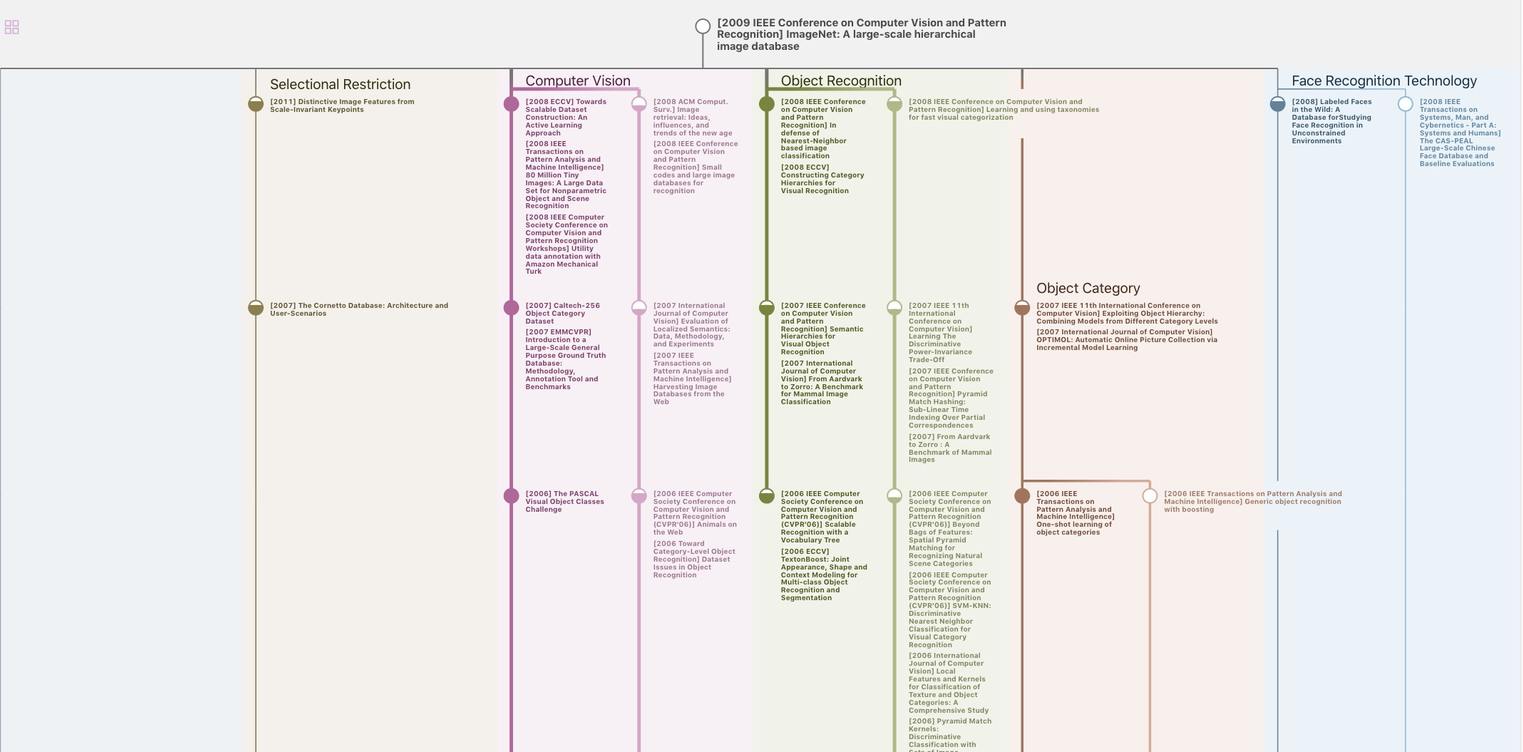
生成溯源树,研究论文发展脉络
Chat Paper
正在生成论文摘要