Segment all roads: Domain generalized freespace detection by robust surface normal information embedding and edge-aware learning
Displays(2024)
摘要
Freespace detection (FD), also known as drivable area detection, plays a crucial role in autonomous driving. However, existing FD methods, which are based on supervised learning, struggle to perform well when testing on out-of-distribution data. Domain generalization is a prospective solution to address this issue, aiming to improve the generalization ability on out-of-distribution data (unseen domains). In this paper, we explore a new task, domain generalized freespace detection, and design a novel approach, which can generalize to both structured urban on-road scenes and unstructured off-road scenes. First, considering the robustness of surface normal (SN) information in diverse domains, we present a robust information embedding module to embed SN information into RGB features. Then, a cross-scale feature robustness enhancement module is introduced to aggregate RGB-SN features across different scales to obtain more domain-invariant features. Moreover, an RGB-SN edge-aware learning strategy is devised to further improve the generalization ability. Extensive experiments demonstrate that the proposed approach achieves superior performance compared to current approaches.
更多查看译文
关键词
Freespace detection,Domain generalization,Robust information embedding,Cross-scale enhancement,Edge-aware learning
AI 理解论文
溯源树
样例
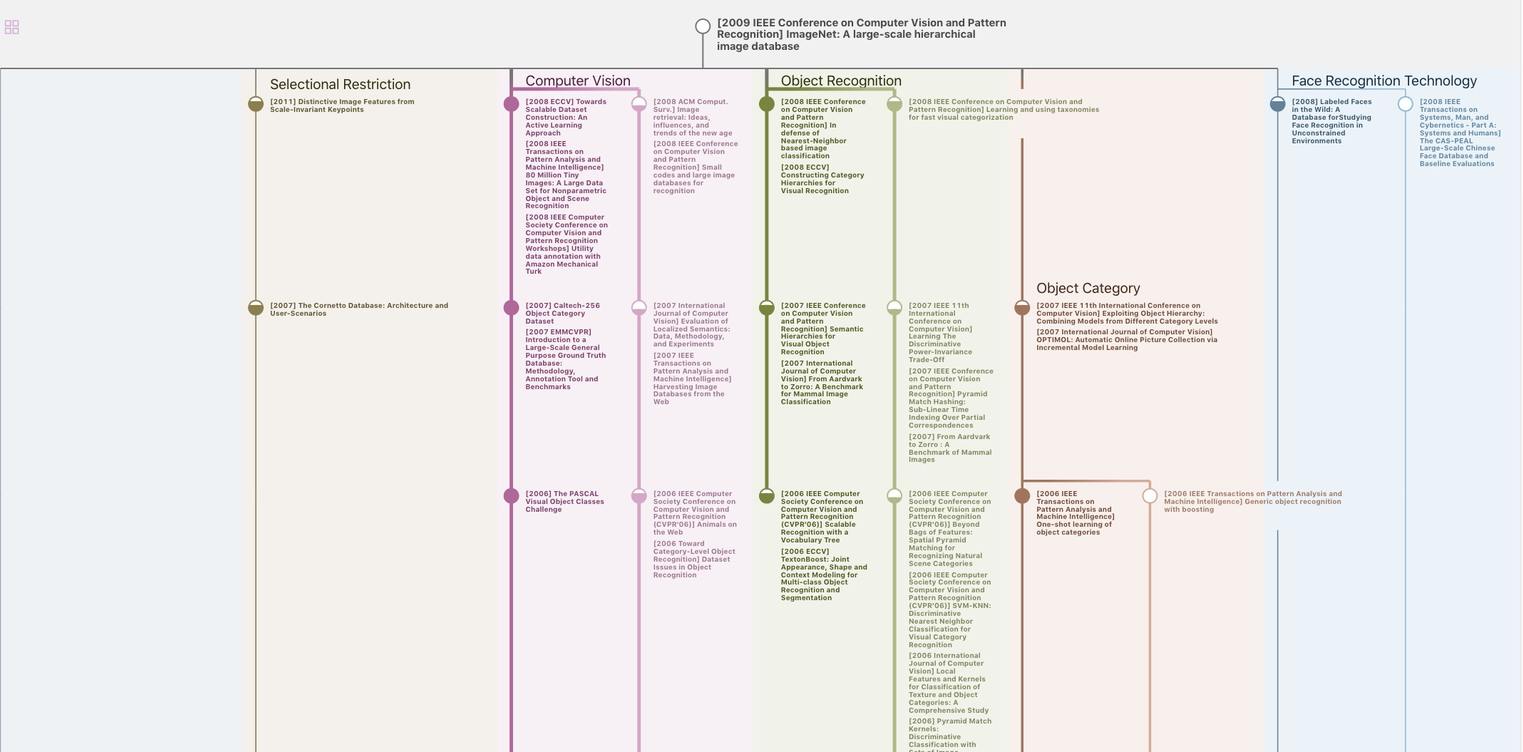
生成溯源树,研究论文发展脉络
Chat Paper
正在生成论文摘要