A novel graph oversampling framework for node classification in class-imbalanced graphs
Science China Information Sciences(2024)
摘要
Graph neural network (GNN) is a promising method to analyze graphs. Most existing GNNs adopt the class-balanced assumption, which cannot deal with class-imbalanced graphs well. The oversampling technique is effective in alleviating class-imbalanced problems. However, most graph oversampling methods generate synthetic minority nodes and their edges after applying GNNs. They ignore the problem that the representations of the original and synthetic minority nodes are dominated by majority nodes caused by aggregating neighbor information through GNN before oversampling. In this paper, we propose a novel graph oversampling framework, termed distribution alignment-based oversampling for node classification in class-imbalanced graphs (named Graph-DAO). Our framework generates synthetic minority nodes before GNN to avoid the dominance of majority nodes caused by message passing in GNNs. Additionally, we introduce a distribution alignment method based on the sum-product network to learn more information about minority nodes. To our best knowledge, it is the first to use the sum-product network to solve the class-imbalanced problem in node classification. A large number of experiments on four real datasets show that our method achieves the optimal results on the node classification task for class-imbalanced graphs.
更多查看译文
关键词
graph neural network,class-imbalanced graphs,sum-product network,oversampling,node classification
AI 理解论文
溯源树
样例
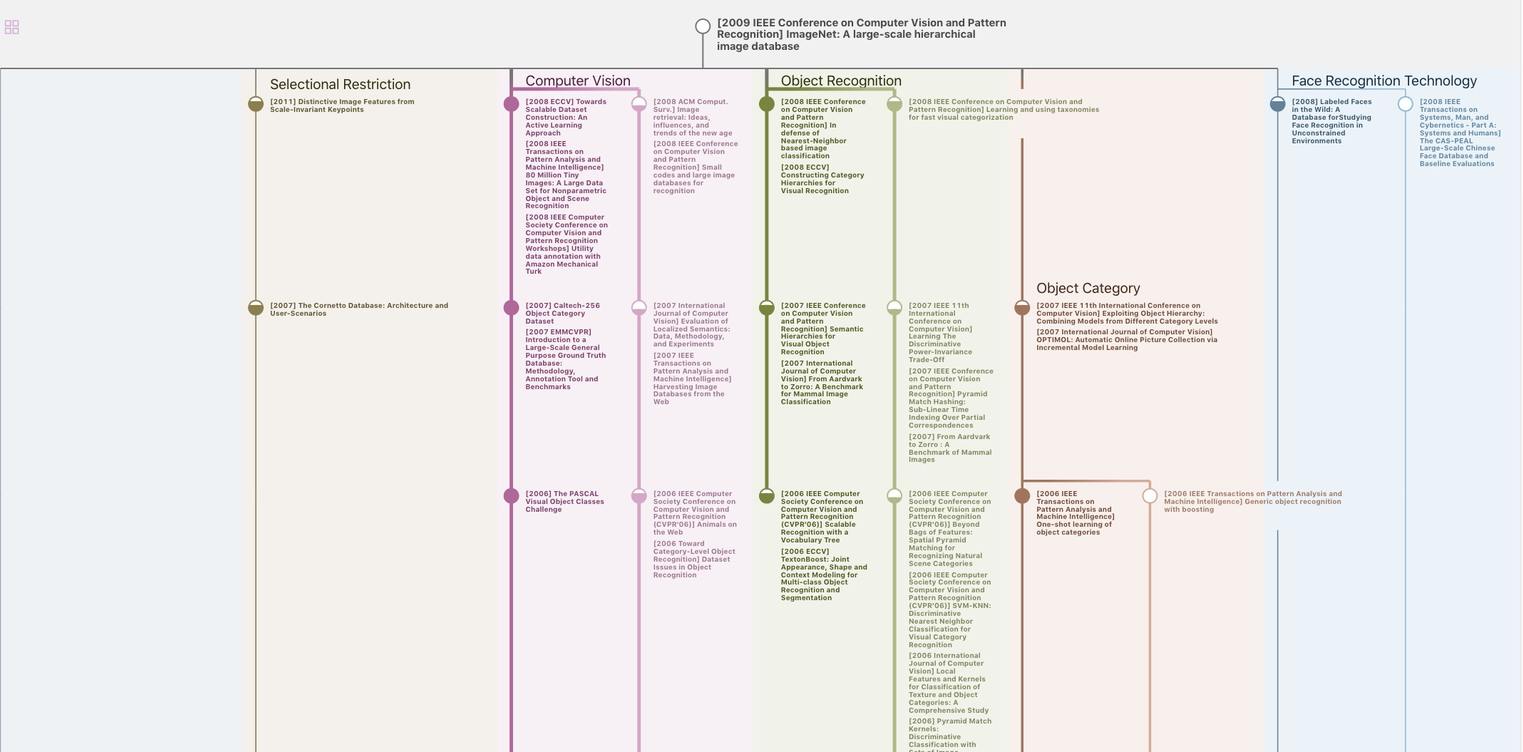
生成溯源树,研究论文发展脉络
Chat Paper
正在生成论文摘要