Fault Diagnosis Generalization Improvement Through Contrastive Learning for a Multistage Centrifugal Pump
IEEE Transactions on Reliability(2024)
摘要
Data scarcity in prognostic and health management research presents a significant challenge, often hindering the performance of supervised models due to the difficulty of acquiring diverse fault mode data during prolonged faultless operation. Conversely, nominal operating condition (NOC) data, including both healthy and varied faulty data, are more readily available due to predelivery inspection. Subsequently, we study this novel and unresolved NOC premise that leverages NOC data along with healthy data from other conditions to construct a fault diagnoser called Res-1D-bootstrap your own latent (BYOL) with the proposed probability distribution generalization strategy. The initial step involves a novel approach to the contrastive transformation optimization with the criteria based solely on similarity loss obtained in the training stage. We then pretrain the fault detector based on our NOC premise, followed by finetuning the network exclusively with NOC data. Given the novelty of our premise, there are few models for direct comparison. Thus, we contrast our approach with a supervised baseline, MoCo, an unoptimized equivalent algorithm, and an equivalent algorithm that solely employs NOC data for pretraining the feature extractor. Empirical results demonstrate our model's superior distribution generalization capabilities through the improved classification accuracy across different operating conditions.
更多查看译文
关键词
BYOL,centrifugal pump,contrastive learning,fault diagnosis,prognostic and health management (PHM)
AI 理解论文
溯源树
样例
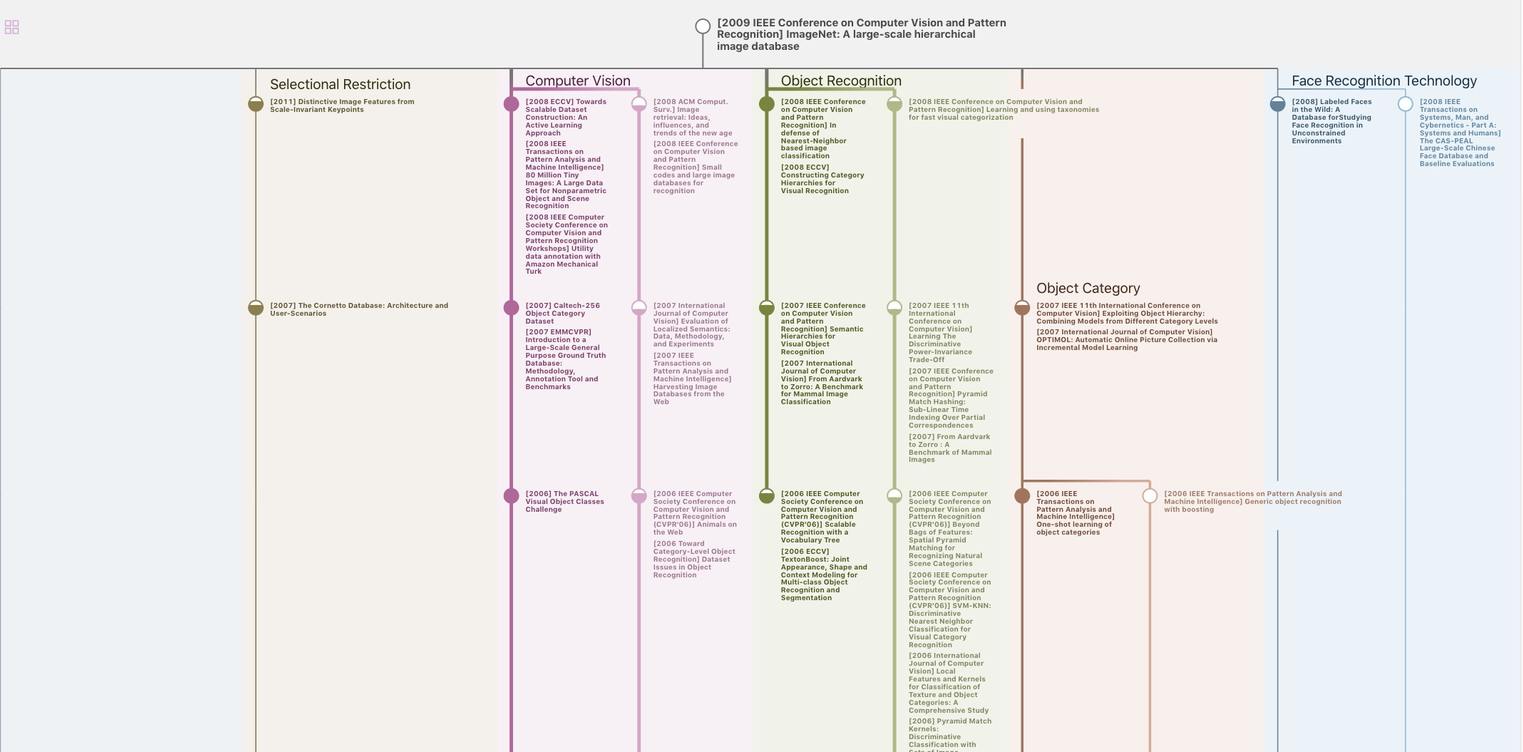
生成溯源树,研究论文发展脉络
Chat Paper
正在生成论文摘要