Knowledge and Data Dual-Driven Fault Diagnosis in Industrial Scenarios: A Survey
IEEE Internet of Things Journal(2024)
摘要
Knowledge and data dual-driven (KDDD) represents a novel paradigm that leverages the strengths of data-driven methods in feature representation and knowledge transfer, while also incorporating expertise accumulated by domain experts. This integration allows KDDD methods to enhance the interpretability, reliability, and robustness of fault diagnosis (FD) approaches, making them widely studied in the field of industrial equipment (IE) FD. Despite the existence of systematic and valuable reviews on IE FD, there remains a gap in the literature regarding the review of KDDD IE FD methods. Therefore, conducting a comprehensive investigation into KDDD IE FD methods is of utmost importance and necessity. Such an investigation will facilitate readers’ understanding of advanced technologies and enable the rapid design of effective solutions for real-world IE FD problems. In this survey, we first outline the limitations of data-driven and knowledge-based FD methods, highlighting the need for KDDD methods. Subsequently, we delve into the details of how domain knowledge can be effectively integrated with deep learning models. Additionally, we analyze challenges of KDDD methods in real-world IE FD applications, while also discussing novel solutions for prospective research directions. Finally, we conclude this survey, emphasizing the inspiration it offers to researchers interested in advancing IE FD, and its potential to stimulate practical IE FD research.
更多查看译文
关键词
Fault Diagnosis,Knowledge and Data Dual-driven,Explainable Artificial Intelligence,Industry 4.0
AI 理解论文
溯源树
样例
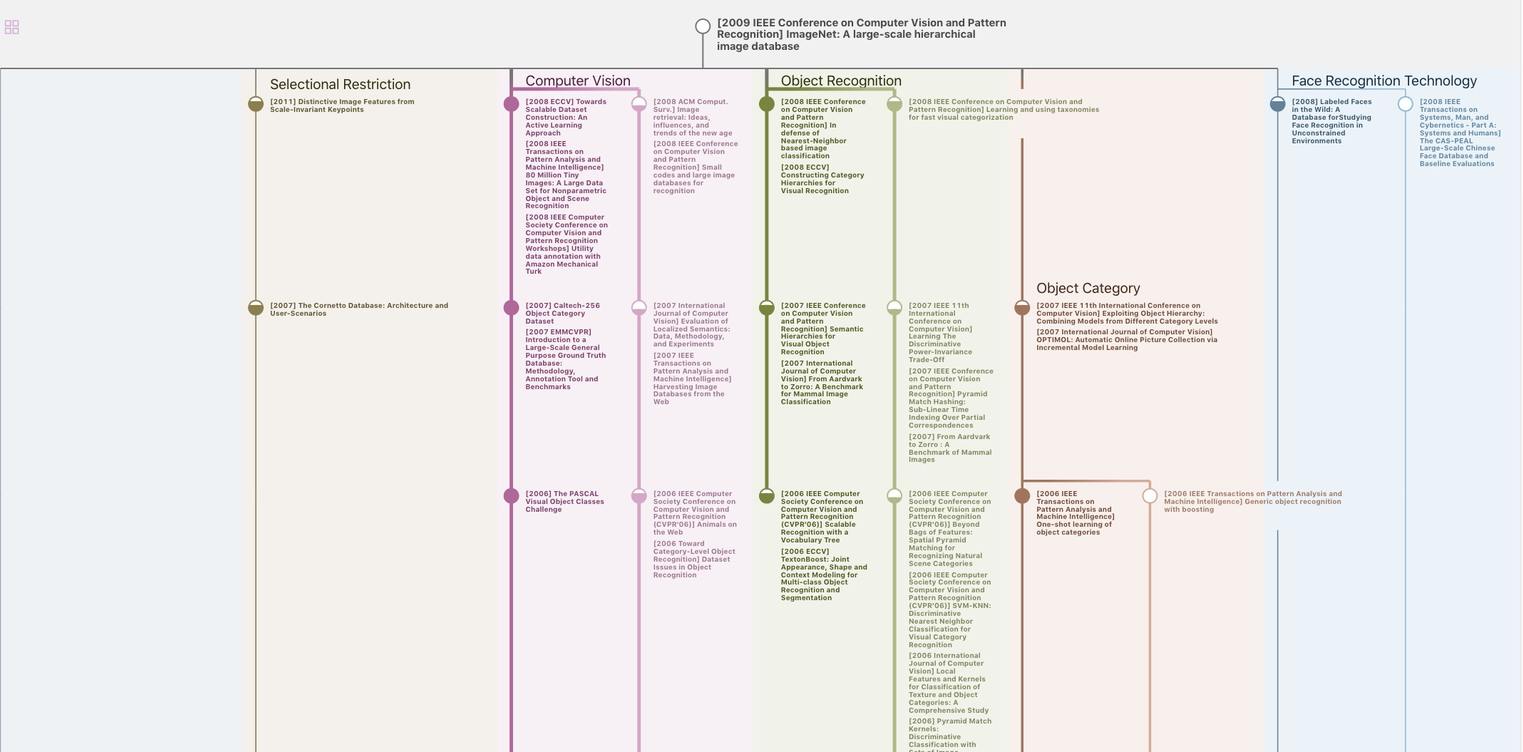
生成溯源树,研究论文发展脉络
Chat Paper
正在生成论文摘要