Contrast Agent Dynamics Determine Radiomics Profiles in Oncologic Imaging.
Cancers(2024)
摘要
BACKGROUND:The reproducibility of radiomics features extracted from CT and MRI examinations depends on several physiological and technical factors. The aim was to evaluate the impact of contrast agent timing on the stability of radiomics features using dynamic contrast-enhanced perfusion CT (dceCT) or MRI (dceMRI) in prostate and lung cancers.
METHODS:Radiomics features were extracted from dceCT or dceMRI images in patients with biopsy-proven peripheral prostate cancer (pzPC) or biopsy-proven non-small cell lung cancer (NSCLC), respectively. Features that showed significant differences between contrast phases were identified using linear mixed models. An L2-penalized logistic regression classifier was used to predict class labels for pzPC and unaffected prostate regions-of-interest (ROIs).
RESULTS:Nine pzPC and 28 NSCLC patients, who were imaged with dceCT and/or dceMRI, were included in this study. After normalizing for individual enhancement patterns by defining seven individual phases based on a reference vessel, 19, 467 and 128 out of 1204 CT features showed significant temporal dynamics in healthy prostate parenchyma, prostate tumors and lung tumors, respectively. CT radiomics-based classification accuracy of healthy and tumor ROIs was highly dependent on contrast agent phase. For dceMRI, 899 and 1027 out of 1118 features were significantly dependent on time after contrast agent injection for prostate and lung tumors.
CONCLUSIONS:CT and MRI radiomics features in both prostate and lung tumors are significantly affected by interindividual differences in contrast agent dynamics.
更多查看译文
关键词
radiomics,machine-learning,contrast agent,lung cancer,prostate cancer
AI 理解论文
溯源树
样例
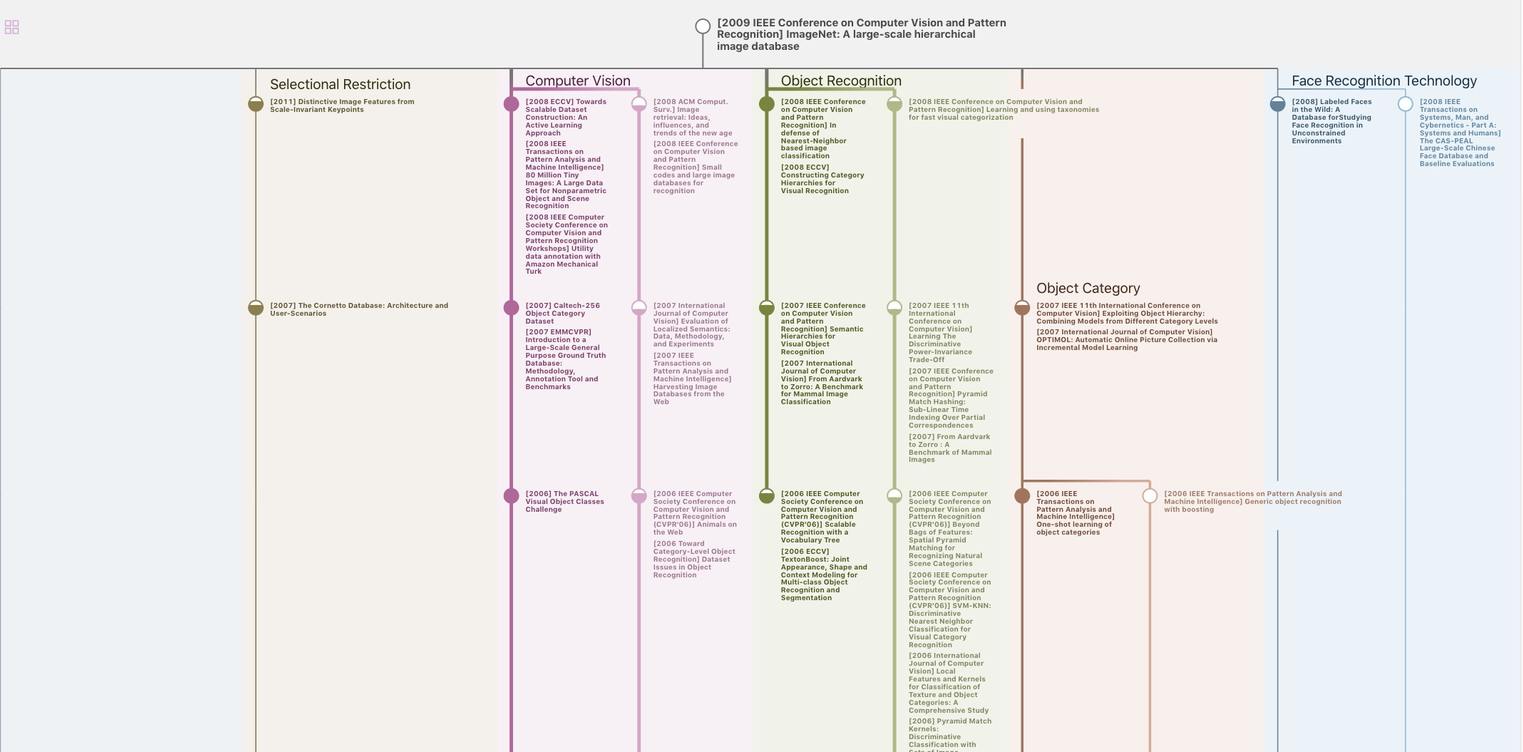
生成溯源树,研究论文发展脉络
Chat Paper
正在生成论文摘要