Defending Against Membership Inference Attacks: RM Learning is All You Need
Information Sciences(2024)
摘要
Large-capacity machine learning models are vulnerable to membership inference attacks that disclose the privacy of the training dataset. The privacy concerns posed by membership inference attacks have inspired many defense strategies.Unfortunately, they have various limitations: 1) failing to achieve a high-quality tradeoff between membership privacy and model utility. 2) Providing little flexibility to users.This paper proposes Repair-Membership Learning(RM Learning), a simple but effective defense mechanism. RM Learning mitigates membership inference attacks by giving each sample of the training dataset an appropriate soft label to reduce the loss gap between the training and testing datasets of the model. Meanwhile, RM Learning controls the distinguishability between the training and testing datasets by limiting the model's loss gap over them with an adjustable parameter λ to give users flexibility. We evaluated the RM Learning algorithm on four datasets with seven models and compared it with three state-of-the-art methods. For instance, we reduce the effectiveness of black-box and white-box membership inference attacks to about 50.0%, with a loss of less than 1.3% of testing accuracy, for the CIFAR10 dataset with the ResNet18 model.
更多查看译文
关键词
Membership inference attacks,white-box attacks,black-box attacks,defense,privacy-preserving machine learning
AI 理解论文
溯源树
样例
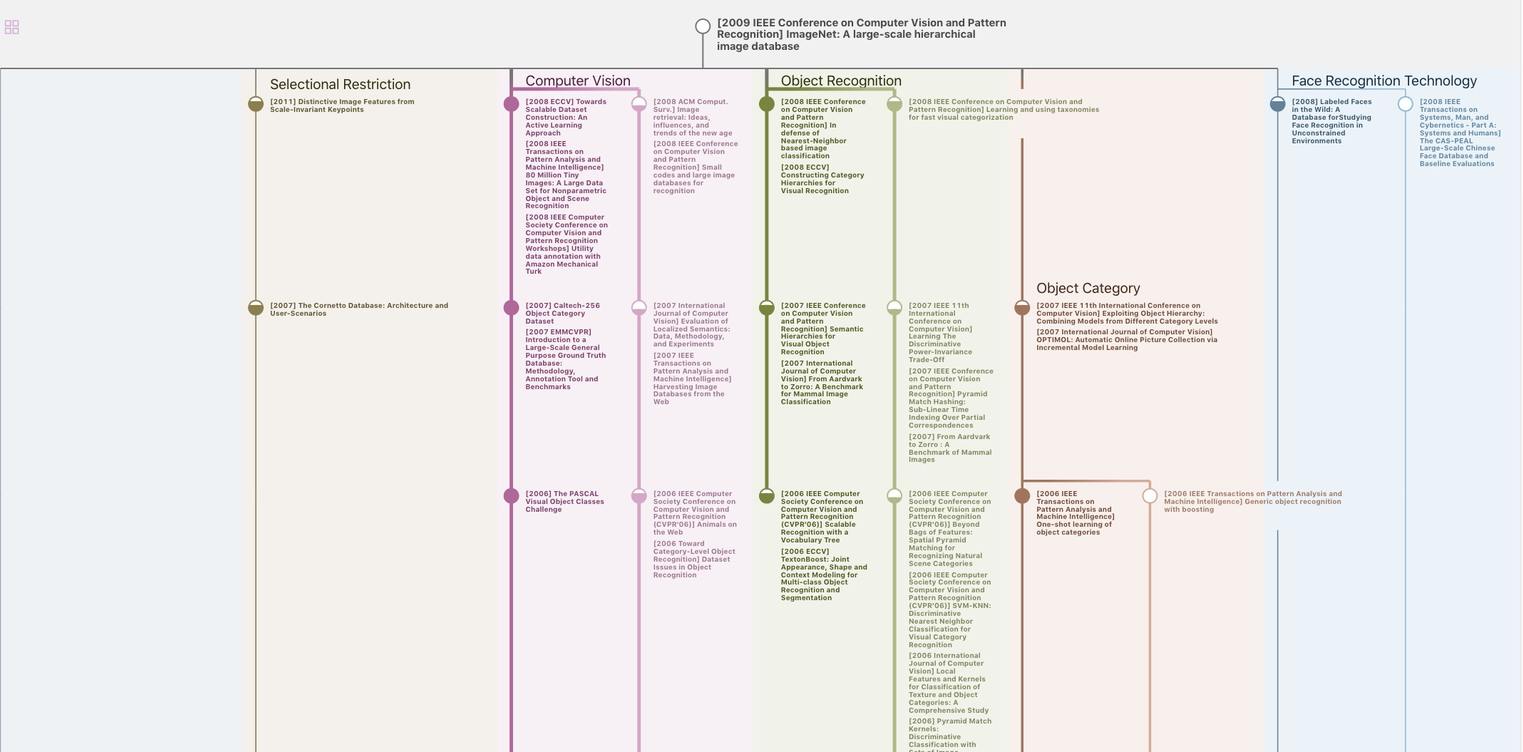
生成溯源树,研究论文发展脉络
Chat Paper
正在生成论文摘要