A Fast Total-Variation Driven Network for Sparse Aperture ISAR Imaging
Digital Signal Processing(2024)
摘要
Conventional Fourier transform-based Inverse Synthetic Aperture Radar (ISAR) imaging cannot deal with the cases of incomplete radar echoes, in which the data needs to be specially processed by Sparse Aperture (SA) imaging methods. Most current SA-ISAR imaging employs sparsity-driven optimization methods. However, the assumption that the target reflectivity is sparse in the spatial domain is insufficient to capture the surface and edge features of the target if no sparse representations are found for the target and well incorporated in the imaging process. In view of the edge-preserving capability of Total Variation (TV) and the strong learning ability of the Deep Neural Network (DNN), we propose a TV-driven network to improve the SA ISAR imaging performance. We first develop a fast TV regularization method to perform the imaging where the Gradient Descent (GD) along with the Momentum Acceleration (MA) are incorporated to increase the computational efficiency. Then, we unwrap the iterative fast TV regularization into a cascaded neural network to make the key imaging parameters learnable, leading to improved imaging performance, which we refer to as FGDTV-Net. Experiments under a variety of scenarios show that the proposed FGDTV-Net for SA-ISAR imaging is superior to existing SA imaging algorithms in preserving surface and edge features and is more robust in low signal-to-noise scenarios.
更多查看译文
关键词
Sparse Aperture,Total Variation,Deep Neural network,ISAR imaging,Gradient Descent
AI 理解论文
溯源树
样例
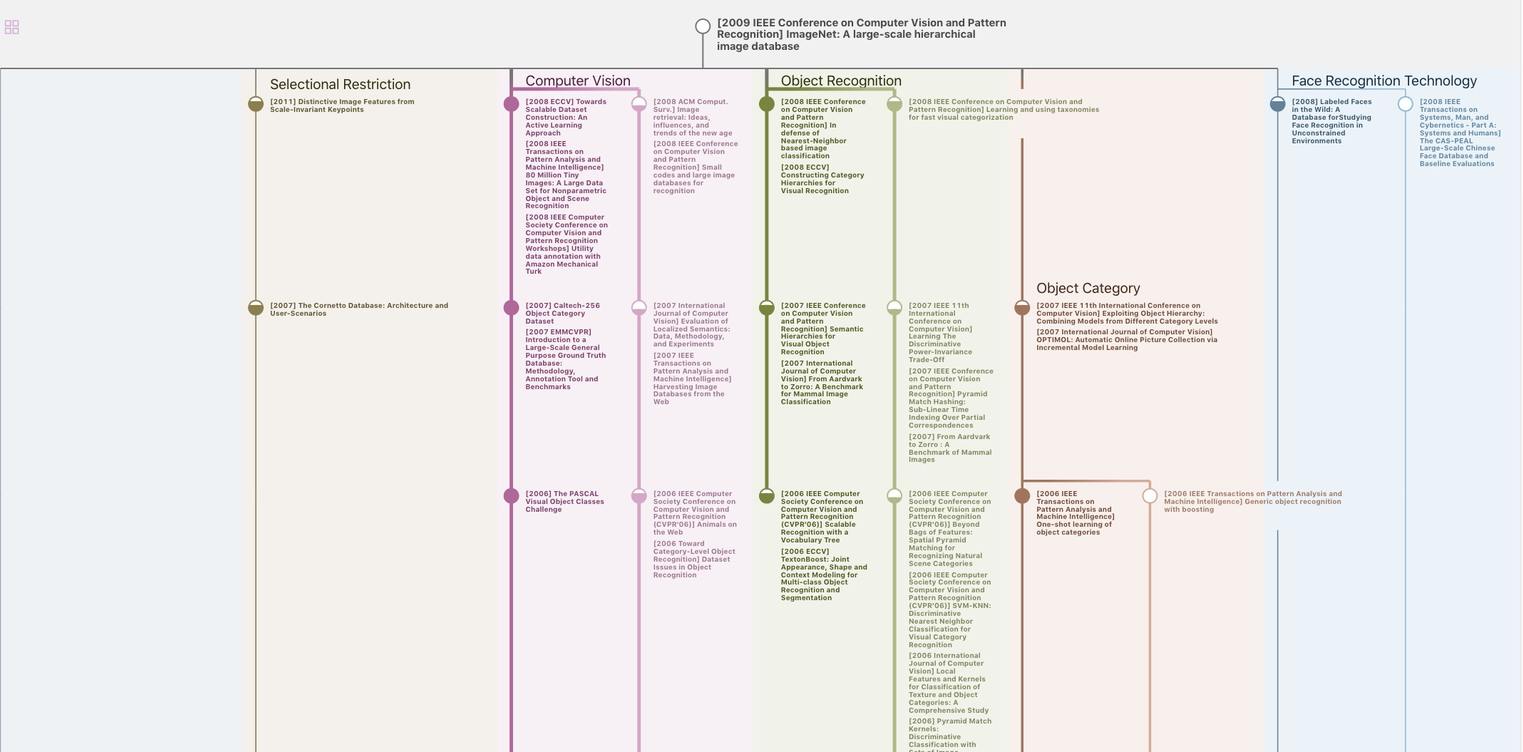
生成溯源树,研究论文发展脉络
Chat Paper
正在生成论文摘要