Manufacturing process selection based on similarity search: incorporating non-shape information in shape descriptor comparison
Journal of Intelligent Manufacturing(2024)
摘要
Given a part design, the task of manufacturing process selection chooses an appropriate manufacturing process to fabricate it. Prior research has traditionally determined manufacturing processes through direct classification. However, an alternative approach to select a manufacturing process for a new design involves identifying previously produced parts with comparable shapes and materials and learning from them. Finding similar designs from a large dataset of previously manufactured parts is a challenging problem. To solve this problem, researchers have proposed different spatial and spectral shape descriptors to extract shape features including the D2 distribution, spherical harmonics (SH), and the Fast Fourier Transform (FFT), as well as the application of different machine learning methods on various representations of 3D part models like multi-view images, voxel, triangle mesh, and point cloud. However, there has not been a comprehensive analysis of these different shape descriptors, especially for part similarity search aimed at manufacturing process selection. To remedy this gap, this paper presents an in-depth comparative study of these shape descriptors for part similarity search. While we acknowledge the importance of factors like part size, tolerance, and cost in manufacturing process selection, this paper focuses on part shape and material properties only. Our findings show that SH performs the best among non-machine learning methods for manufacturing process selection, yielding 97.96
更多查看译文
关键词
Manufacturing process selection,Shape descriptors,Deep learning,Similarity search
AI 理解论文
溯源树
样例
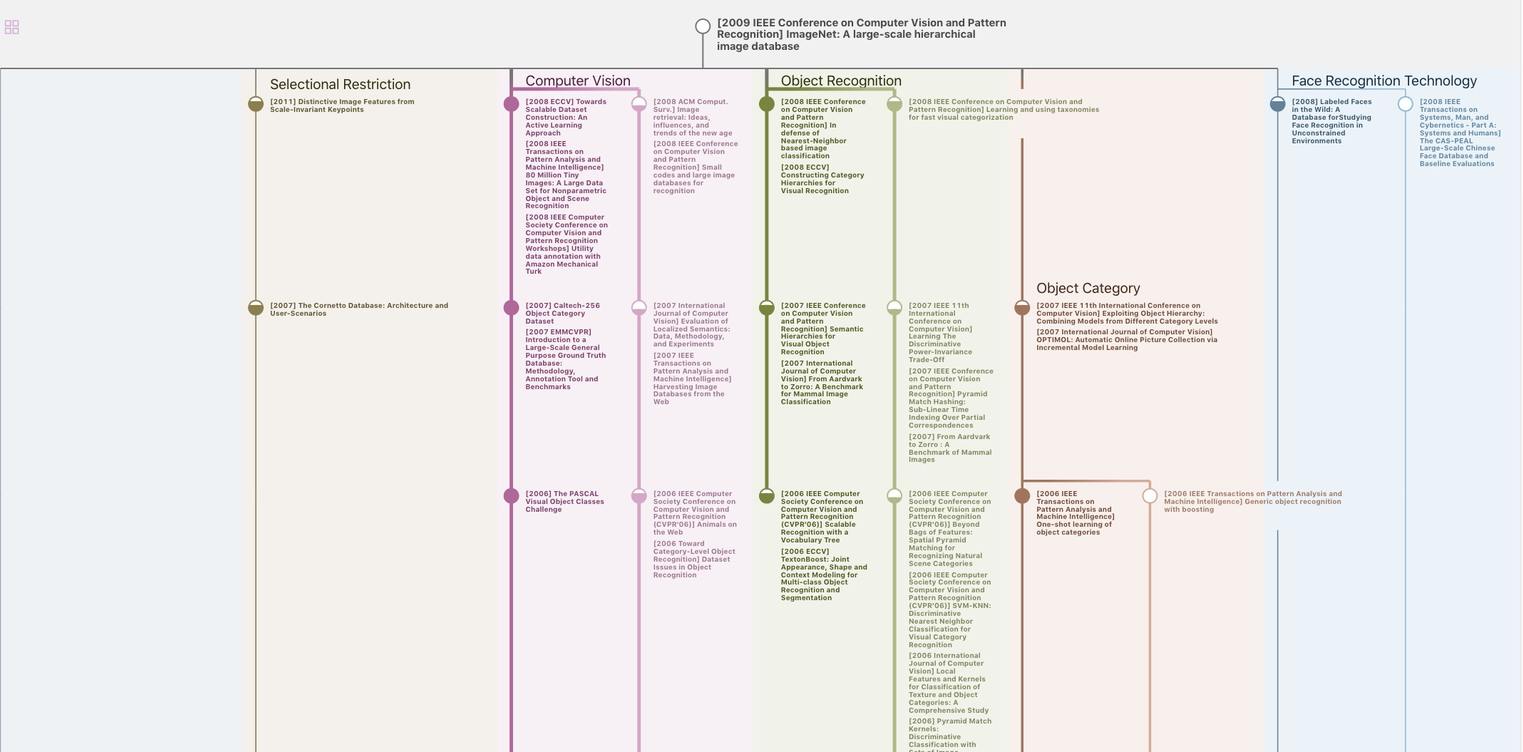
生成溯源树,研究论文发展脉络
Chat Paper
正在生成论文摘要