Deep Learning Missing Value Imputation on Traffic Data Using Self-Attention and GAN-based Methods
2024 Panhellenic Conference on Electronics & Telecommunications (PACET)(2024)
摘要
Open traffic data are sensor generated data with real-time information about the movement of vehicles on roads and other transportation networks and are valuable for decisionmaking such as better traffic management. One of the major challenge with these datasets is the imputation of missing values, which can be addressed using methods that range from statistical methods to machine learning and deep learning. This work investigates the effectiveness of three deep learning imputation methods namely, the self-attention SAITS, the GAN-based USGAN, and Transformer. Using open traffic data collected using an Application Programming Interface from the Swiss Open Data Portal, with introduced 20% artificial missing values added with the Missing Completely at Random mechanism, the deep learning methods were compared against one machine learning model (K-NN) and two traditional statistical methods (Median and LOCF). Results reveal that deep learning-based imputation methods outperform the other counterparts.
更多查看译文
关键词
open traffic data,missing data imputation,deep learning,self-attention,generative networks,transformers
AI 理解论文
溯源树
样例
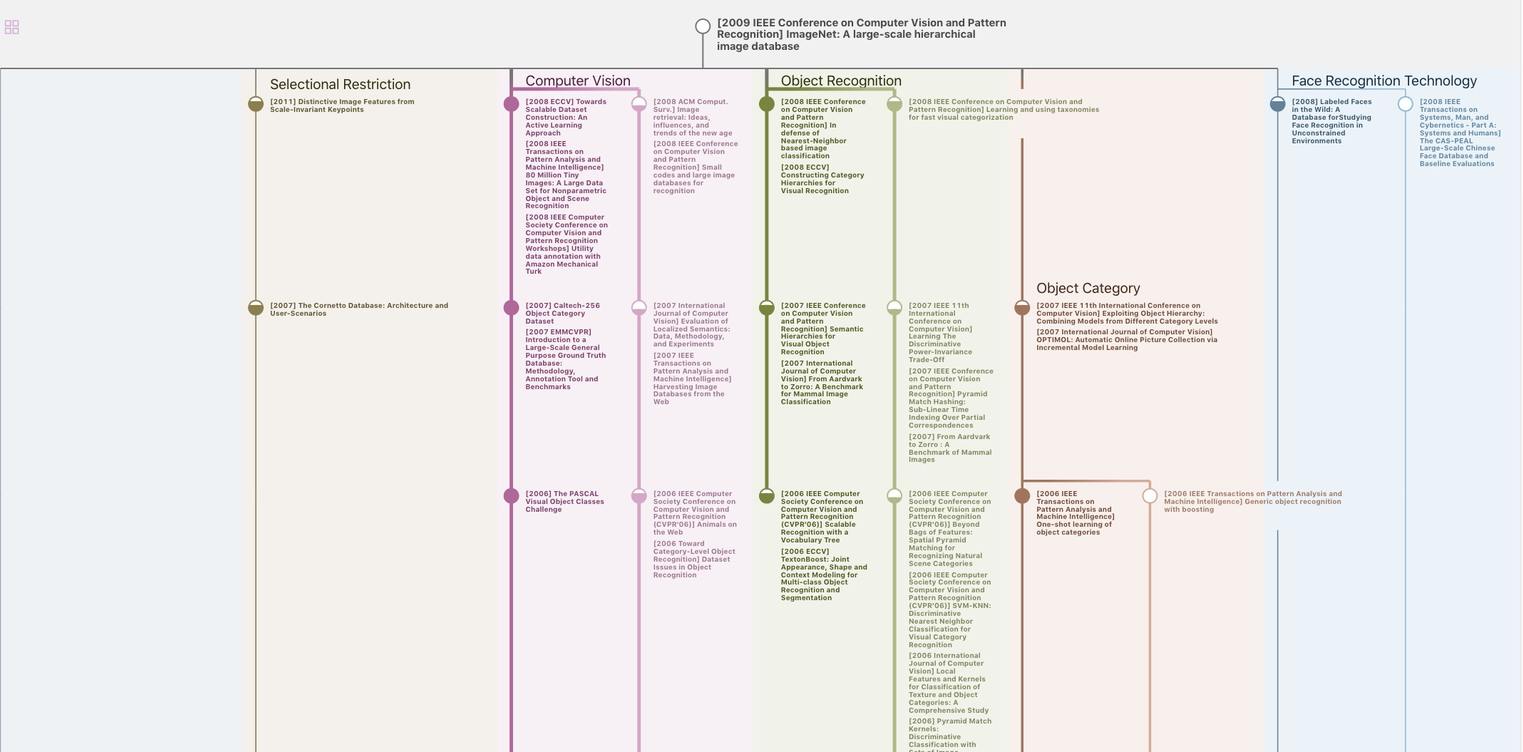
生成溯源树,研究论文发展脉络
Chat Paper
正在生成论文摘要