Aerial View 3D Human Pose Estimation Using Double Vector Quantized-Variational AutoEncoders.
IEEE/CVF Winter Conference on Applications of Computer Vision(2024)
摘要
This study introduces a novel methodology for the precise estimation of the three-dimensional (3D) pose of individuals based on images captured from aerial viewpoints, particularly from top-to-bottom viewpoints. A motion capture system utilized for surveillance purposes is frequently constrained in its ability to capture dynamic scenarios, primarily due to the limited field of view of a third-person-view camera. To address the problem at hand, various approaches employ aerial views to overcome limitations in spatial constraints. Nevertheless, when observing the unmanned aerial vehicle (UAV) from an aerial perspective, it is common for the lower body to appear diminished and obstructed by the upper body. This phenomenon results in pose estimation that is highly unreliable and inaccurate. To overcome the existing limitation, we present a novel approach that utilizes the Vector Quantized- Variational AutoEncoder (VQ-VAE) to accurately predict and optimize the 3D human pose from aerial images. Thus, we introduce a novel pipeline for pose estimation and optimization using the codebook by learning aerial image features and pose features from large human pose datasets with VQ-VAE. The proposed method with the vector quantizer of VQ-VAEs can help improve the generalization capabilities of 3D pose estimation from aerial top-to-bottom viewpoints. Through conducting comparative experiments, our method has demonstrated a substantial enhancement in performance compared to those of existing state-of-the-art methods.
更多查看译文
关键词
Pose Estimation,Human Pose Estimation,Human Pose,3D Human Pose,Aerial 3D,Prediction Accuracy,Image Features,Codebook,Upper Body,Unmanned Aerial Vehicles,Aerial Images,Motion Capture,Motion Capture System,Variational Autoencoder,3D Pose,Vector Quantization,Heatmap,Comparative Method,Convolutional Network,Convolutional Neural Network,Multi-view Images,Pose Prediction,Latent Code,2D Pose,Accurate Pose,Model Predictive Control,Ground Truth Pose,Real-world Datasets,Procrustes Analysis,Joint Position
AI 理解论文
溯源树
样例
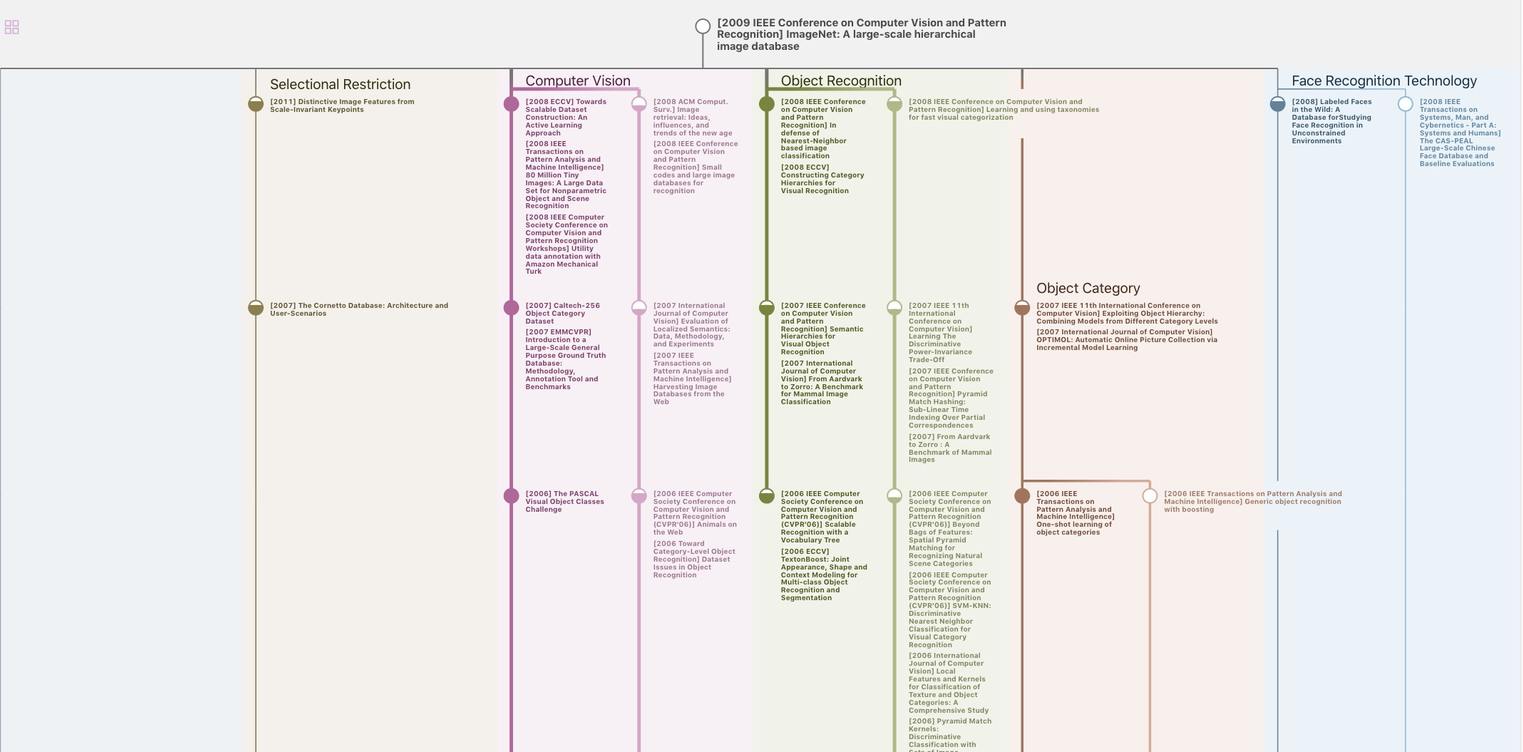
生成溯源树,研究论文发展脉络
Chat Paper
正在生成论文摘要