Kernel support vector machine classifiers with ℓ0-norm hinge loss
Neurocomputing(2024)
摘要
Support vector machines (SVMs) are some of the most successful machine learning models for binary classification problems. Their key idea is maximizing the margin from the data to the hyperplane subject to correct classification on training samples. In the SVM training model, hinge loss is sensitive to label noise and unstable for resampling. Moreover, binary loss is the most natural choice for modeling classification errors. Motivated by this, we focus on the kernel SVM with the ℓ0-norm hinge loss (referred to as ℓ0-KSVM); this is a composite function of the hinge loss and ℓ0-norm, which has the potential to address the aforementioned challenges. In consideration of the non-convexity and non-smoothness of the ℓ0-norm hinge loss, we first characterize the limiting subdifferential of the ℓ0-norm hinge loss and then derive the equivalent relationship between the proximal stationary point, the Karush–Kuhn–Tucker point, and the local optimal solution of ℓ0-KSVM. Second, we develop an alternating direction method of multipliers for ℓ0-KSVM and find that any limit point of the sequence generated by the proposed algorithm is a locally optimal solution. Lastly, experiments on synthetic and real datasets demonstrate that ℓ0-KSVM can achieve comparable accuracy compared to the standard kernel SVMs and that the former generally results in fewer support vectors.
更多查看译文
关键词
Kernel support vector machines,ℓ0-norm hinge loss,ℓ0-proximal operator,Proximal stationary points,Karush–Kuhn–Tucker points,Alternating direction method of multipliers
AI 理解论文
溯源树
样例
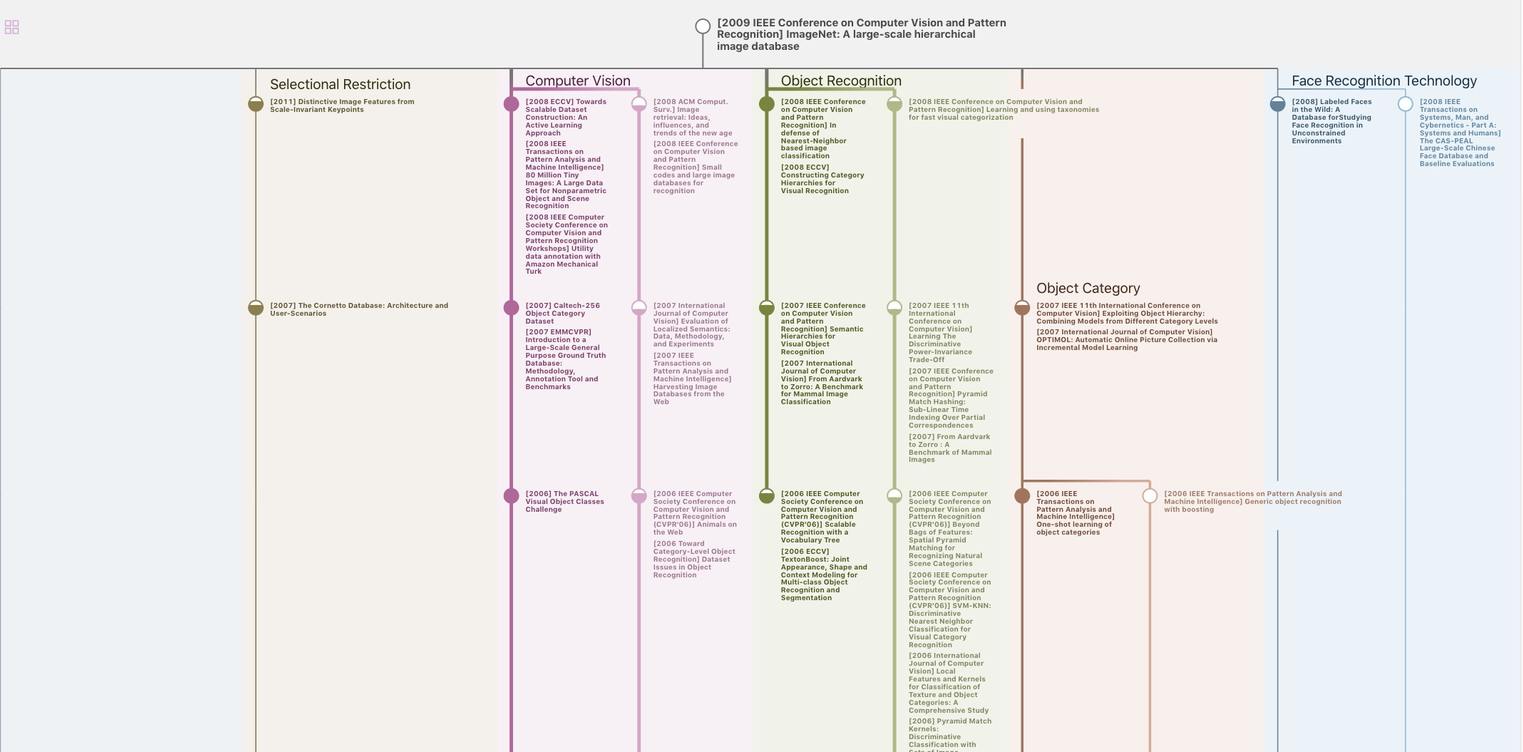
生成溯源树,研究论文发展脉络
Chat Paper
正在生成论文摘要