MMI-ML: Maximize Mutual Information between Different Views for Few-Shot Remote Sensing Image Classification
IEEE Geoscience and Remote Sensing Letters(2024)
摘要
Few-shot learning is widely applied in the current stage for remote sensing image classification to use prior knowledge to identify new classes faster. However, since existing few-shot remote sensing image classification methods only process the feature vectors extracted from the complete image, ignoring the localized knowledge in the input incorporated into the target can better utilize the contextual information to capture more local details. To address these problems, we propose a metric learning framework based on maximizing mutual information between different views (MMI-ML). Specifically, we introduce a self-supervised model to train an embedding network with enhanced feature representation by maximizing mutual information of global features and local features at different scales. In addition, we design a new embedding network to make it more appropriate for the self-supervised model. Finally, we devise a new loss function in the training stage, which can effectively speed up the convergence of the model. We conduct comparative experiments on three public remote sensing datasets, and the experimental results show that the classification accuracy of the MMI-ML framework is improved by up to 3.22%.
更多查看译文
关键词
Maximize mutual information,few-shot learning,metric learning,remote sensing image classification
AI 理解论文
溯源树
样例
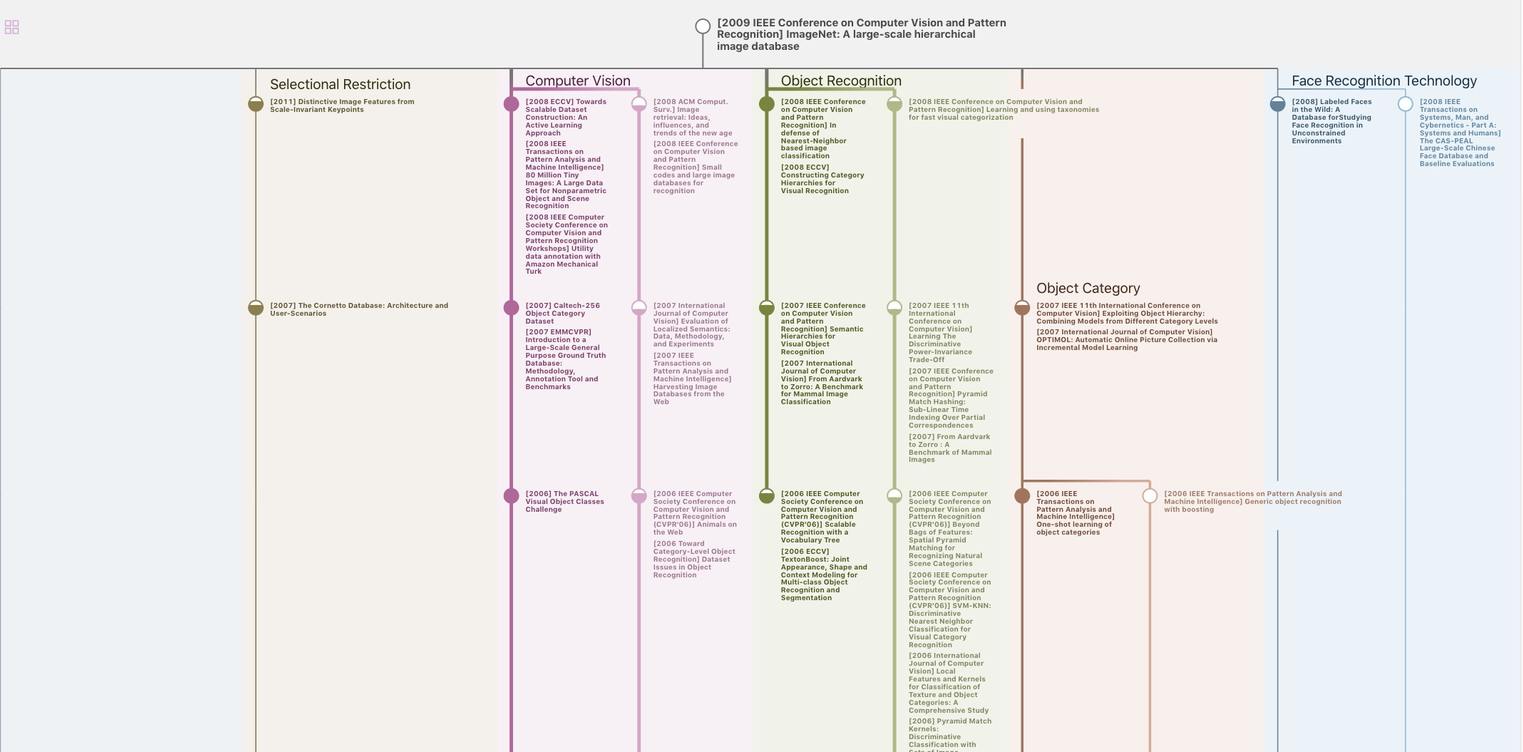
生成溯源树,研究论文发展脉络
Chat Paper
正在生成论文摘要