Unsupervised Domain Adaptation Enhanced by Fuzzy Prompt Learning
IEEE Transactions on Fuzzy Systems(2024)
摘要
Unsupervised Domain Adaptation (UDA) addresses the challenge of distribution shift between a labeled source domain and an unlabeled target domain by utilizing knowledge from the source. Traditional UDA methods mainly focus on single-modal scenarios, either vision or language, thus not fully exploring the advantages of multi-modal representations. Vision-Language models utilize multi-modal information, applying prompt learning techniques for addressing target domain tasks. Motivated by the recent advancements in pre-trained Vision-Language models, this paper expands the UDA framework to incorporate multi-modal approaches using fuzzy techniques. The adoption of fuzzy techniques, preferred over conventional domain adaptation methods, is based on two key aspects: the nature of prompt learning is intrinsically linked to fuzzy logic, and the superior capability of fuzzy techniques in processing soft information and effectively utilizing inherent relationships both within and across domains. To this end, we propose UDA enhanced by
Fuzz
y Prompt
Le
arning (FUZZLE), a simple and effective method for aligning the source and target domains via domain-specific prompt learning. Specifically, we introduce a novel technique to enhance prompt learning in the target domain. This method integrates fuzzy C-means clustering and a novel instance-level fuzzy vector into the prompt learning loss function, minimizing the distance between prompt cluster centers and instance prompts, thereby enhancing the prompt learning process. In addition, we propose a Kullback-Leibler (KL) divergence-based loss function with a fuzzification factor. This function is designed to minimize the distribution discrepancy in the classification of similar cross-domain data, aligning domain-specific prompts during the training process. We contribute an in-depth analysis to understand the effectiveness of Extensive experiments demonstrate that our method achieves superior performance on standard UDA benchmarks.
更多查看译文
关键词
Fuzzy clustering,prompt learning,domain adaptation,transfer learning
AI 理解论文
溯源树
样例
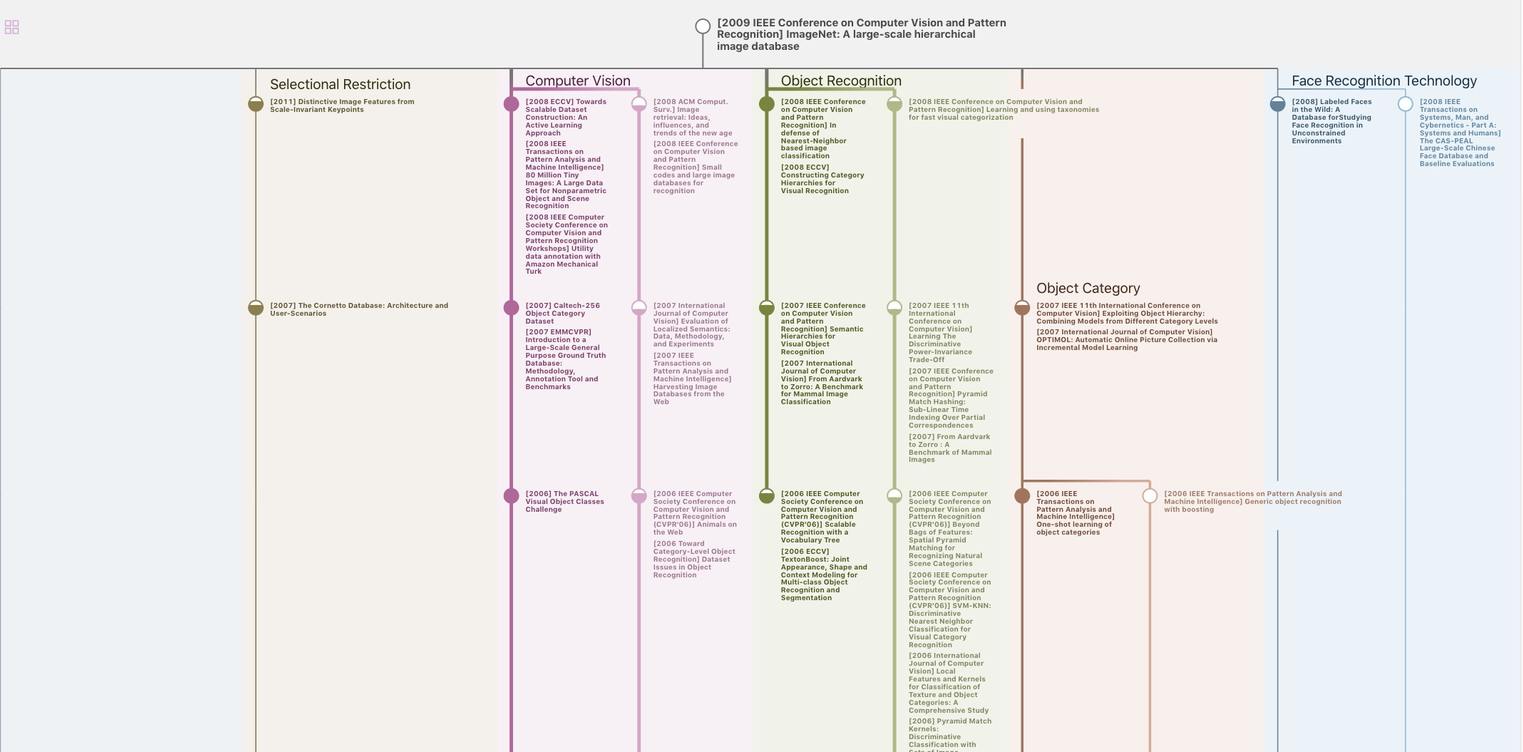
生成溯源树,研究论文发展脉络
Chat Paper
正在生成论文摘要