Understanding Human-COVID-19 Dynamics using Geospatial Big Data: A Systematic Literature Review
arxiv(2024)
摘要
The COVID-19 pandemic has changed human life. To mitigate the pandemic's
impacts, different regions implemented various policies to contain COVID-19 and
residents showed diverse responses. These human responses in turn shaped the
uneven spatial-temporal spread of COVID-19. Consequently, the human-pandemic
interaction is complex, dynamic, and interconnected. Delineating the reciprocal
effects between human society and the pandemic is imperative for mitigating
risks from future epidemics. Geospatial big data acquired through mobile
applications and sensor networks have facilitated near-real-time tracking and
assessment of human responses to the pandemic, enabling a surge in researching
human-pandemic interactions. However, these investigations involve inconsistent
data sources, human activity indicators, relationship detection models, and
analysis methods, leading to a fragmented understanding of human-pandemic
dynamics. To assess the current state of human-pandemic interactions research,
we conducted a synthesis study based on 67 selected publications between March
2020 and January 2023. We extracted key information from each article across
six categories, e.g., research area and time, data, methodological framework,
and results and conclusions. Results reveal that regression models were
predominant in relationship detection, featured in 67.16
papers employed spatial-temporal models, notably underrepresented in the
existing literature. Studies examining the effects of policies and human
mobility on the pandemic's health impacts were the most prevalent, each
comprising 12 articles (17.91
bidirectional interactions between human responses and the COVID-19 spread.
These findings shed light on the need for future research to spatially and
temporally model the long-term, bidirectional causal relationships within
human-pandemic systems.
更多查看译文
AI 理解论文
溯源树
样例
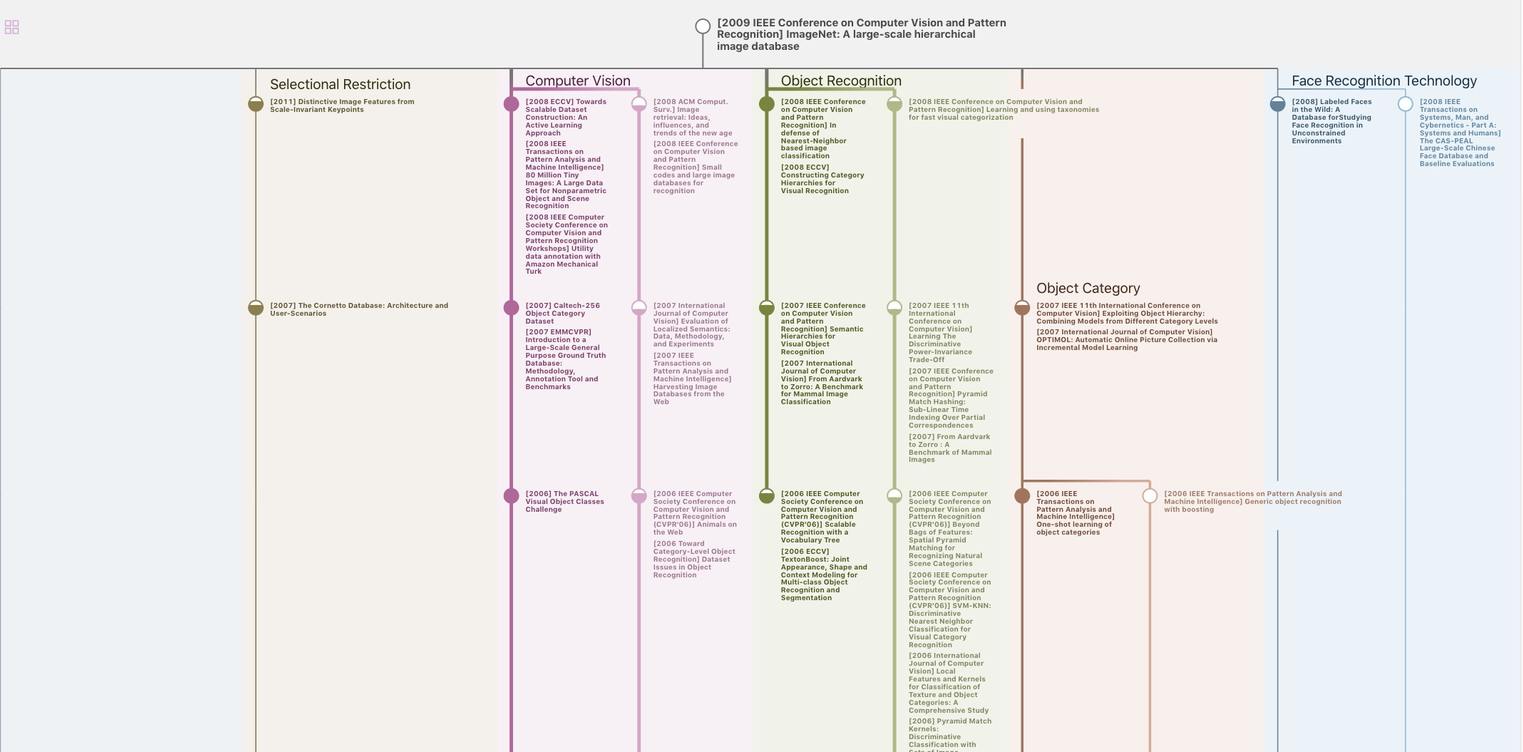
生成溯源树,研究论文发展脉络
Chat Paper
正在生成论文摘要