Rank-based Hashing for Effective and Efficient Nearest Neighbor Search for Image Retrieval
ACM Transactions on Multimedia Computing, Communications, and Applications(2024)
摘要
The large and growing amount of digital data creates a pressing need for approaches capable of indexing and retrieving multimedia content. A traditional and fundamental challenge consists of effectively and efficiently performing nearest-neighbor searches. After decades of research, several different methods are available, including trees, hashing, and graph-based approaches. Most of the current methods exploit learning to hash approaches based on deep learning. In spite of effective results and compact codes obtained, such methods often require a significant amount of labeled data for training. Unsupervised approaches also rely on expensive training procedures usually based on a huge amount of data. In this work, we propose an unsupervised data-independent approach for nearest neighbor searches, which can be used with different features, including deep features trained by transfer learning. The method uses a rank-based formulation and exploits a hashing approach for efficient ranked list computation at query time. A comprehensive experimental evaluation was conducted on 7 public datasets, considering deep features based on CNNs and Transformers. Both effectiveness and efficiency aspects were evaluated. The proposed approach achieves remarkable results in comparison to traditional and state-of-the-art methods. Hence, it is an attractive and innovative solution, especially when costly training procedures need to be avoided.
更多查看译文
AI 理解论文
溯源树
样例
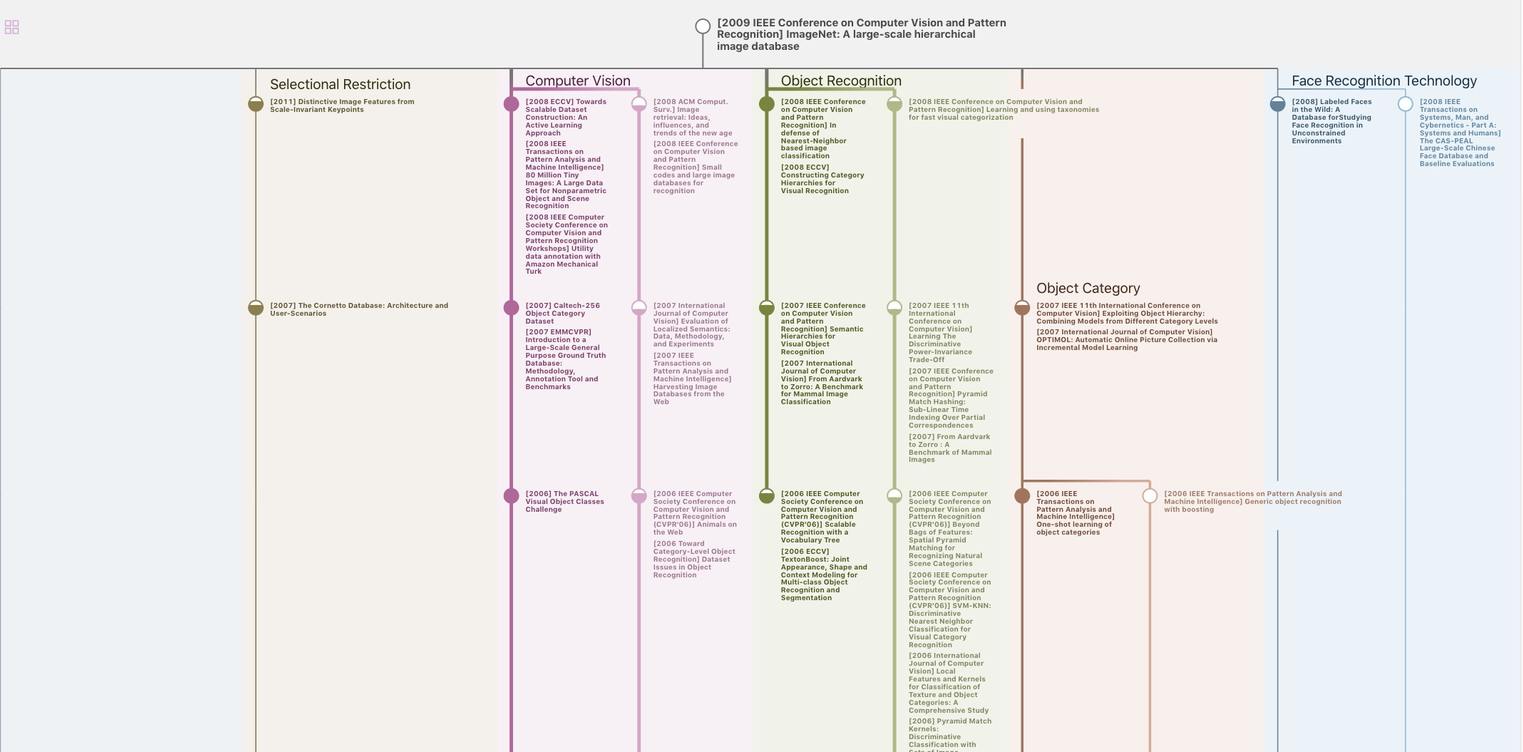
生成溯源树,研究论文发展脉络
Chat Paper
正在生成论文摘要