A Prognosis Swin Transformer Network for Rolling Bearing Remaining Useful Life Prediction
2023 International Conference on Sensing, Measurement & Data Analytics in the era of Artificial Intelligence (ICSMD)(2023)
摘要
The research on the remaining useful life (RUL) of rolling bearings based on deep learning plays an important role in the safe and economic operation of rotating machinery. In order to improve the matching degree of network structure and vibration signal data, a novel method named Prognosis Swin Transformer (ProgSwT) is developed for bearing RUL prediction. In the ProgSwT network, the hierarchical structure and sliding window feature extraction are retained, and the number of stages in the Swin Transformer and the number of network layers per level are optimized. Specifically, the input signal first obtains features through the Patch Partition, then transmits into three consecutive stages composed of Linear Embedding and Swin Transformer Block, and finally enters the overall average pooling layer to output the RUL result. As a result, the flexibility and portability of the network structure can be heightened while the computational complexity of the network can be decreased when extracting the characteristics of vibration signals. The experimental results show that the ProgSwT network has good prediction accuracy in the RUL prediction.
更多查看译文
关键词
Prediction,Prognosis Swin Transformer (ProgSwT),Rolling bearing,Remaining Useful Life (RUL)
AI 理解论文
溯源树
样例
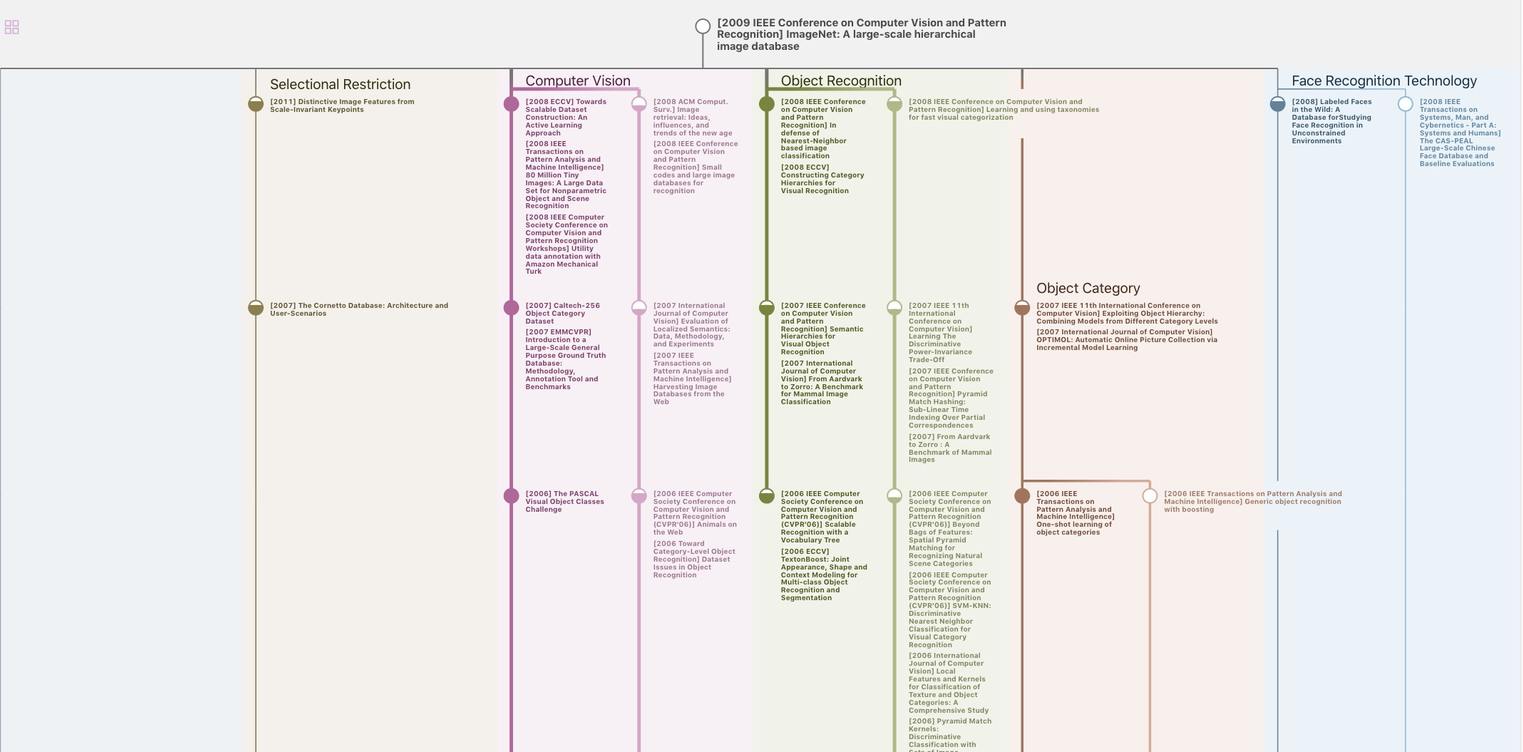
生成溯源树,研究论文发展脉络
Chat Paper
正在生成论文摘要