Machine learning guided design of mechanically efficient metamaterials with auxeticity
Materials Today Communications(2024)
摘要
Chiral structural materials with excellent mechanical properties and tunability offer potential solutions for deformable robots, multifunctional structures and smart sensor innovations. Traditional design and empirical optimization methods are still challenging to achieve efficient structural design and optimization that meets the desired goals. This study explores reentrant anti-tetrachiral (RATC) structures with various design parameters and reveals their extensive mechanical response space by increasing and randomly varying their parameter combinations(the ranges of the parameters for an individual cell are: r [0, 6], αx [0, 12], αy [0, 12], β [0, 1], γ [0, 1], θ [0, 90], E0 [100, 10000], and P0 [0, 0.5]). Then, the relationship between the multidimensional parameters and the mechanical performance of the structure is established by utilizing the fast neural network inference capability of deep learning. Finally, the design and optimization of the RATC structure was successfully achieved by combining the deep learning model with genetic algorithm. The results show that the optimized structure shows significant improvements in terms of stiffness (+1324%), Poisson's ratio (+187.8%), and lightweight performance (+70.8%) compared to the reference structure in the literature. In addition, the optimized results exceeded the scope of the dataset in terms of the targeted properties. The optimized structures were successfully prepared by additive manufacturing techniques and subsequently subjected to experimental and simulation analyses to validate their performance. The results of the study showed a high degree of consistency between the data from the experimental and simulation analyses.
更多查看译文
关键词
Metamaterials,RATC,machine learning,inverse design
AI 理解论文
溯源树
样例
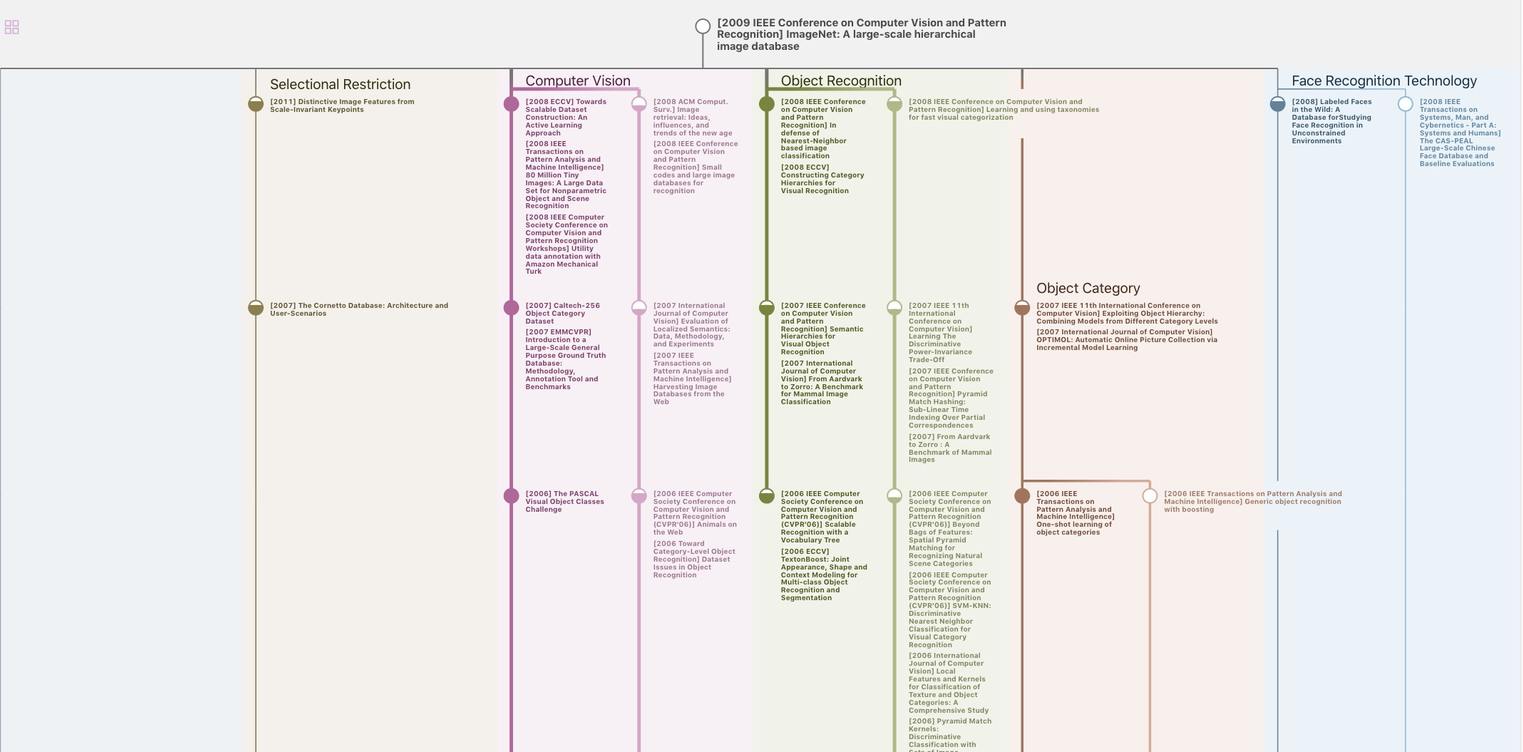
生成溯源树,研究论文发展脉络
Chat Paper
正在生成论文摘要