Anatomy of Industrial Scale Multilingual ASR
arxiv(2024)
摘要
This paper describes AssemblyAI's industrial-scale automatic speech
recognition (ASR) system, designed to meet the requirements of large-scale,
multilingual ASR serving various application needs. Our system leverages a
diverse training dataset comprising unsupervised (12.5M hours), supervised
(188k hours), and pseudo-labeled (1.6M hours) data across four languages. We
provide a detailed description of our model architecture, consisting of a
full-context 600M-parameter Conformer encoder pre-trained with BEST-RQ and an
RNN-T decoder fine-tuned jointly with the encoder. Our extensive evaluation
demonstrates competitive word error rates (WERs) against larger and more
computationally expensive models, such as Whisper large and Canary-1B.
Furthermore, our architectural choices yield several key advantages, including
an improved code-switching capability, a 5x inference speedup compared to an
optimized Whisper baseline, a 30
data, and a 90
significantly improved time-stamp accuracy. Throughout this work, we adopt a
system-centric approach to analyzing various aspects of fully-fledged ASR
models to gain practically relevant insights useful for real-world services
operating at scale.
更多查看译文
AI 理解论文
溯源树
样例
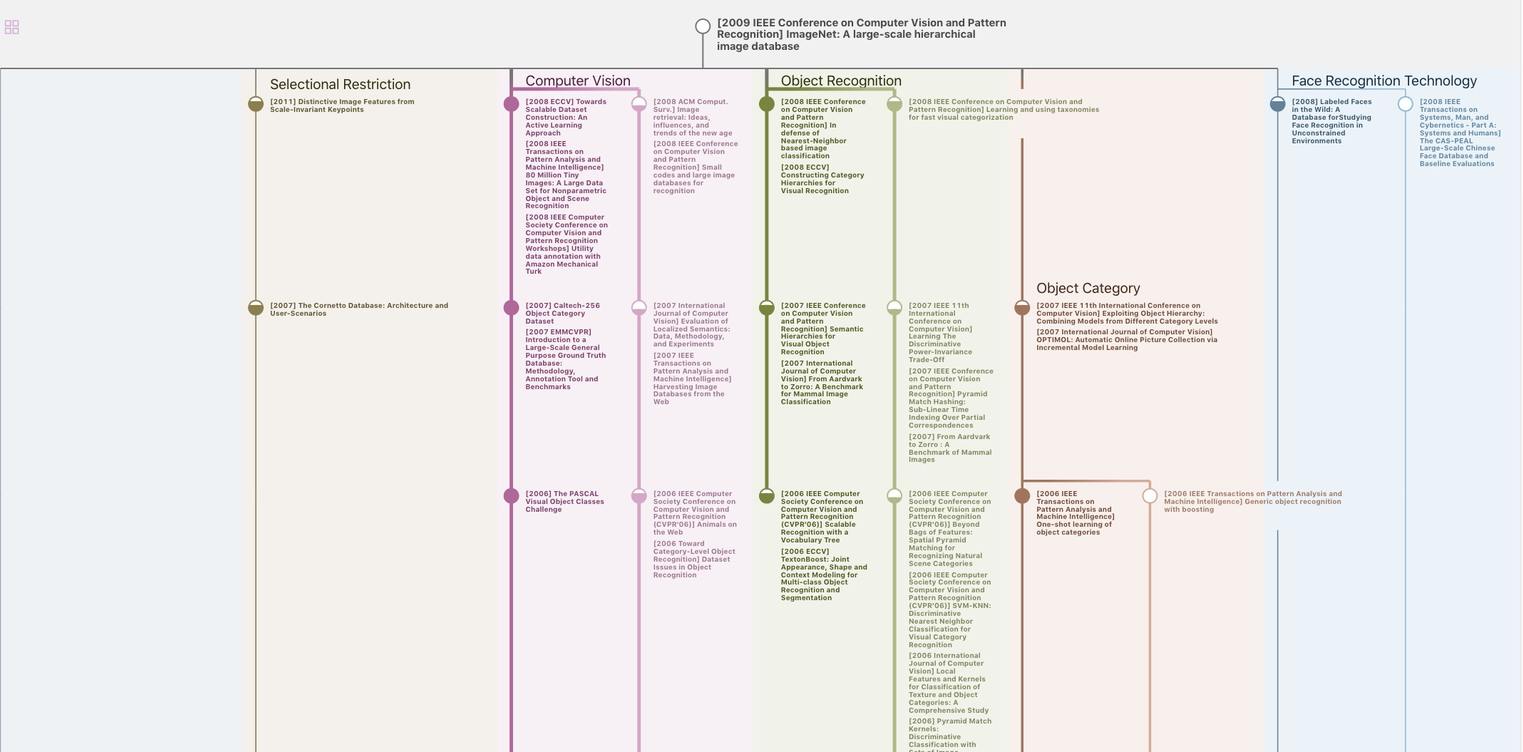
生成溯源树,研究论文发展脉络
Chat Paper
正在生成论文摘要