Semantic Approach to Quantifying the Consistency of Diffusion Model Image Generation
arxiv(2024)
摘要
In this study, we identify the need for an interpretable, quantitative score
of the repeatability, or consistency, of image generation in diffusion models.
We propose a semantic approach, using a pairwise mean CLIP (Contrastive
Language-Image Pretraining) score as our semantic consistency score. We applied
this metric to compare two state-of-the-art open-source image generation
diffusion models, Stable Diffusion XL and PixArt-α, and we found
statistically significant differences between the semantic consistency scores
for the models. Agreement between the Semantic Consistency Score selected model
and aggregated human annotations was 94
SDXL and a LoRA-fine-tuned version of SDXL and found that the fine-tuned model
had significantly higher semantic consistency in generated images. The Semantic
Consistency Score proposed here offers a measure of image generation alignment,
facilitating the evaluation of model architectures for specific tasks and
aiding in informed decision-making regarding model selection.
更多查看译文
AI 理解论文
溯源树
样例
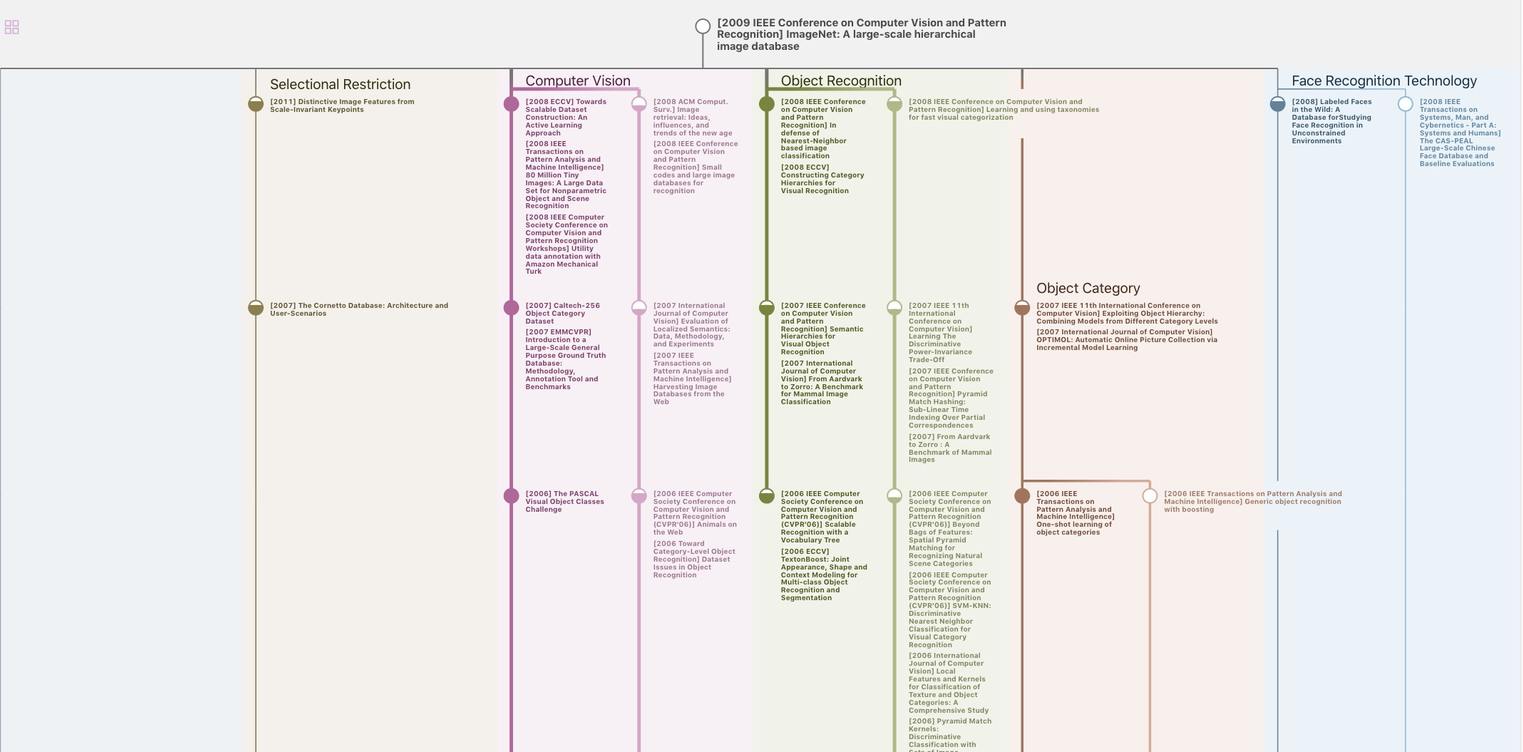
生成溯源树,研究论文发展脉络
Chat Paper
正在生成论文摘要