Instance Segmentation Method of Tunnel Facility Images Along High-Speed Rail based on Deep Learning
2023 Global Reliability and Prognostics and Health Management Conference (PHM-Hangzhou)(2023)
摘要
In order to ensure the normal operation of high-speed rail, the safety risks of tunnel facilities along the high-speed rail are the focus of safety inspections. In recent years, the remote inspection method of unmanned aerial vehicle(UAV) has gradually replaced manual inspection. With large amounts of data collected from image captures, there is a high demand for accurate identification of inspection targets, posing a major challenge for researchers. This study focuses on a deep neural network-based instance segmentation method to assist inspection personnel in eliminating complex background interference from UAV-captured images, accurately identifying facility hazards, and reducing missed detections and false alarms. Specifically, considering the dispersed locations of tunnel facilities along high-speed rail, the SOLOv2 model is employed to directly segment different facility targets using their position information. To address the low precision issue caused by imbalanced dataset distribution, a Balanced Focal Loss is proposed to balance the model parameters. To improve the segmentation boundary precision, a Self-Adaptive Boundary Refinement method is proposed and applied after SOLOv2. Experimental results demonstrate that the proposed method achieves a 2.6% improvement in average precision(AP) compared to the state-of-the-art instance segmentation method SOLOv2, reaching 61.1% AP on the tunnel entrance facility image dataset. Our method can be applied to remote inspection tasks of tunnel facilities along high-speed rail.
更多查看译文
关键词
tunnel facility images along high-speed rail,instance segmentation,sample imbalance,Focal Loss,segmentation boundary refinement
AI 理解论文
溯源树
样例
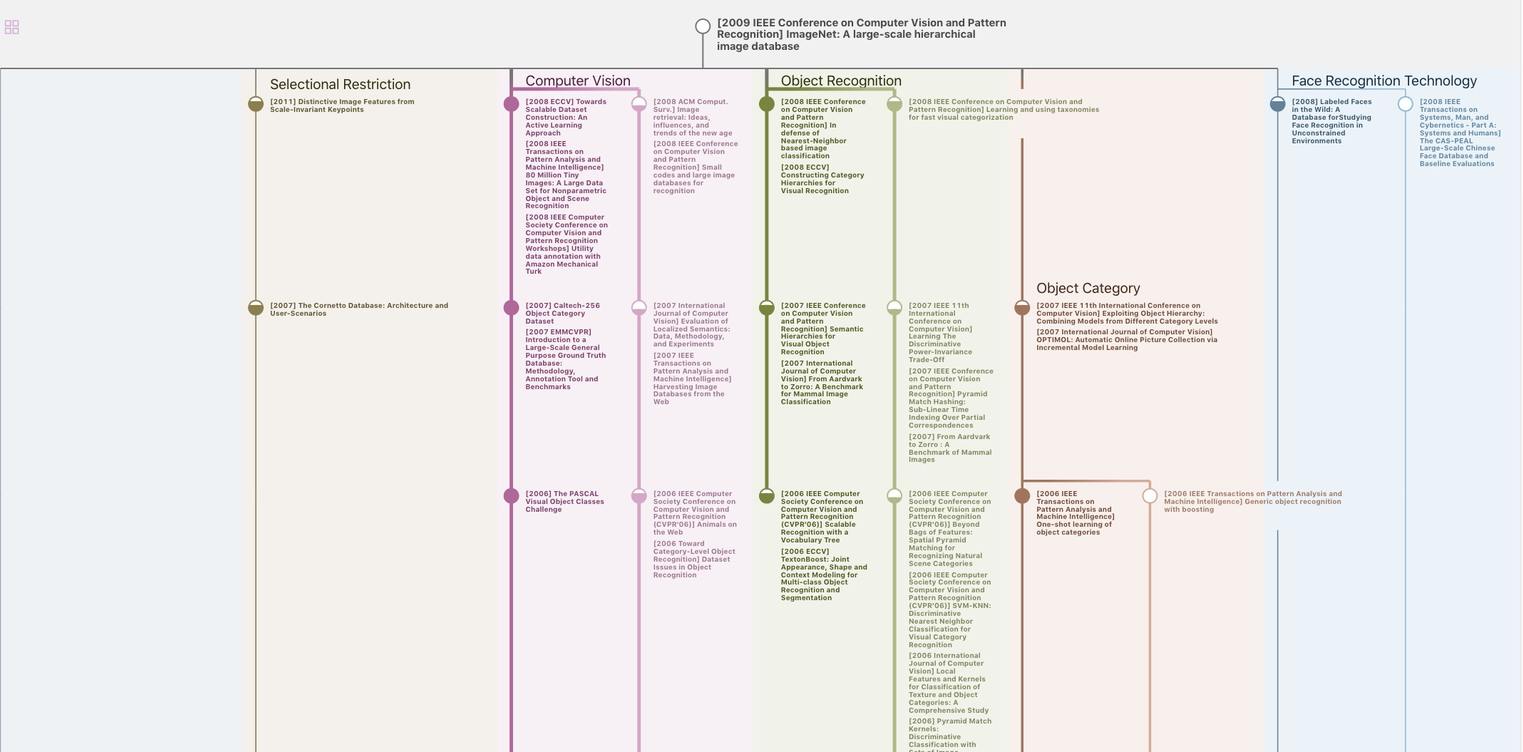
生成溯源树,研究论文发展脉络
Chat Paper
正在生成论文摘要