A Novel Deep Multi-Source Domain Adaptation Framework for Remaining Useful Life Prediction Across Operating Condition
2023 Global Reliability and Prognostics and Health Management Conference (PHM-Hangzhou)(2023)
摘要
In recent years, there has been a rapid development of data-driven domain adaptive remaining useful life prediction methods. It can more effectively predict training (source) and testing (target) condition monitoring data with different distributions. In practice, the target domain is not correlated with only one source domain, and label data can be obtained from multiple diverse domains, which leads to incomplete information from the Sigle-source Unsupervised Domain Adaptation (SUDA) method, thus limiting the prediction accuracy. This paper is the first to propose a multi-source Unsupervised Domain Adaptation (MUDA) method for remaining useful life prediction. A challenging practical situation was investigated where the source domain data came from multiple datasets with different operating conditions. A prediction framework MFDAN (Multiple Feature Domain Align Network) is proposed, consisting of a feature extraction phase and a regression prediction phase. Extensive validations using an aero-engine dataset, with different domain adaptation methods and metrics, show that the proposed multi-source prediction method offers a new and promising approach.
更多查看译文
关键词
RUL prediction,Transfer learning,Unsupervised domain adaptation,Multi-source domain,Unlabeled target domain
AI 理解论文
溯源树
样例
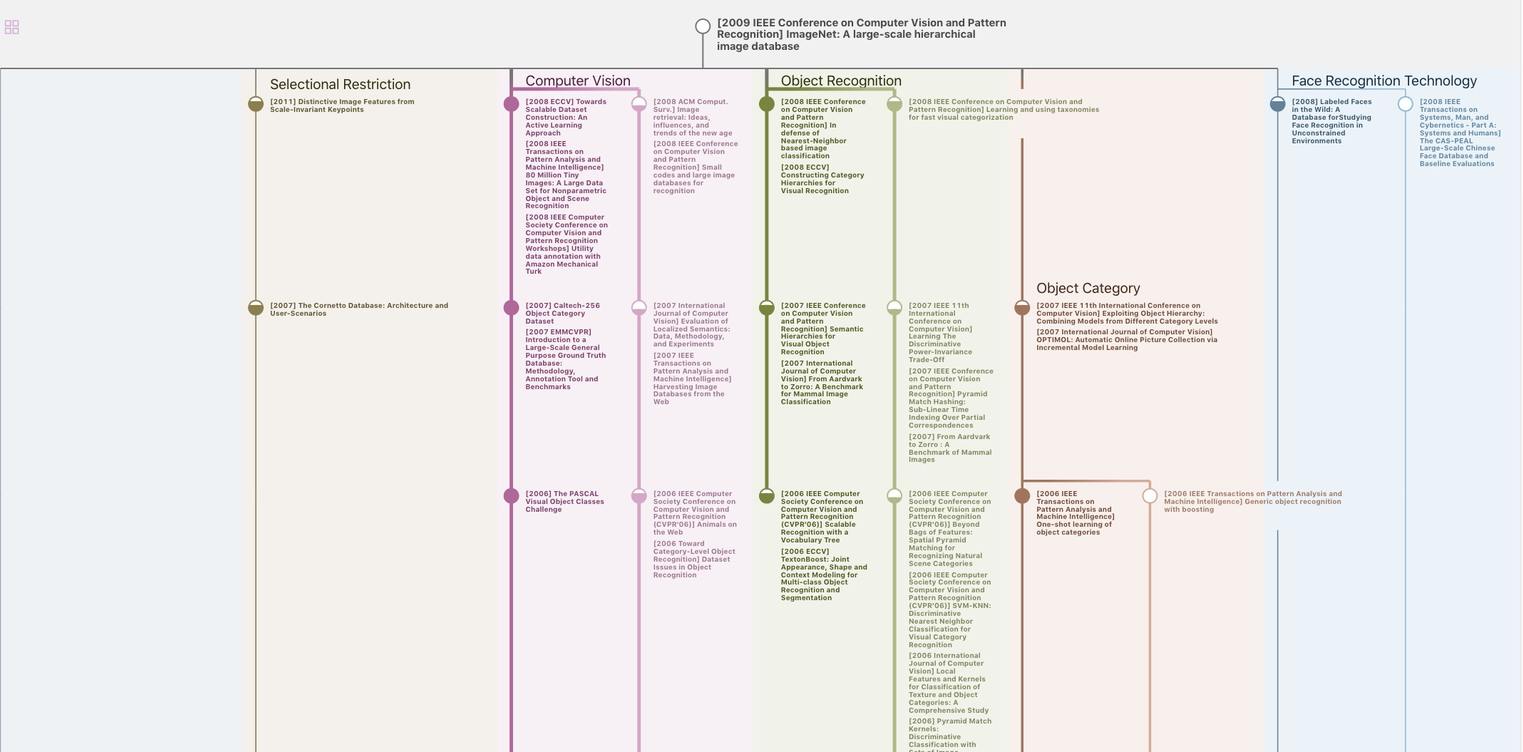
生成溯源树,研究论文发展脉络
Chat Paper
正在生成论文摘要