Energy-saving operation in urban rail transit: A deep reinforcement learning approach with speed optimization
Travel Behaviour and Society(2024)
摘要
The energy consumption of urban rail transit plays a significant role in the operating costs of trains. It is particularly crucial to decrease the energy consumption of the traction power supply in subway systems, as it accounts for approximately half of the total energy consumption of the subway operating organization. To overcome the limitations of traditional real-time speed profile generation methods and the limited exploration capabilities of popular reinforcement learning algorithms in the speed domain, this paper presents the Energy-Saving Maximum Entropy Deep Reinforcement Learning (ES-MEDRL) algorithm. The ES-MEDRL algorithm incorporates Lagrange multipliers and maximum policy entropy as penalties to formulate a novel objective function. This function aims to intensify exploration in the speed domain, minimize train traction energy consumption, and ensure a balance between ride comfort, punctuality, and safety within the subway system. This leads to the optimization of speed profile strategies. To further reduce energy consumption, this paper proposes a secondary optimization strategy for the energy-saving speed profile. This approach involves trading acceptable travel time for improved energy efficiency. To validate the performance of the proposed model and algorithm, numerical experiments are conducted using the Yizhuang Line of the Beijing Metro. The findings demonstrate a minimum 20 % increase in energy efficiency with the ES-MEDRL algorithm compared to manual driving. This algorithm can guide energy-efficient train operations at the planning level.
更多查看译文
关键词
Urban rail transit,Reinforcement learning,Train energy saving,Actor-Critic,Train Speed Profile Optimization
AI 理解论文
溯源树
样例
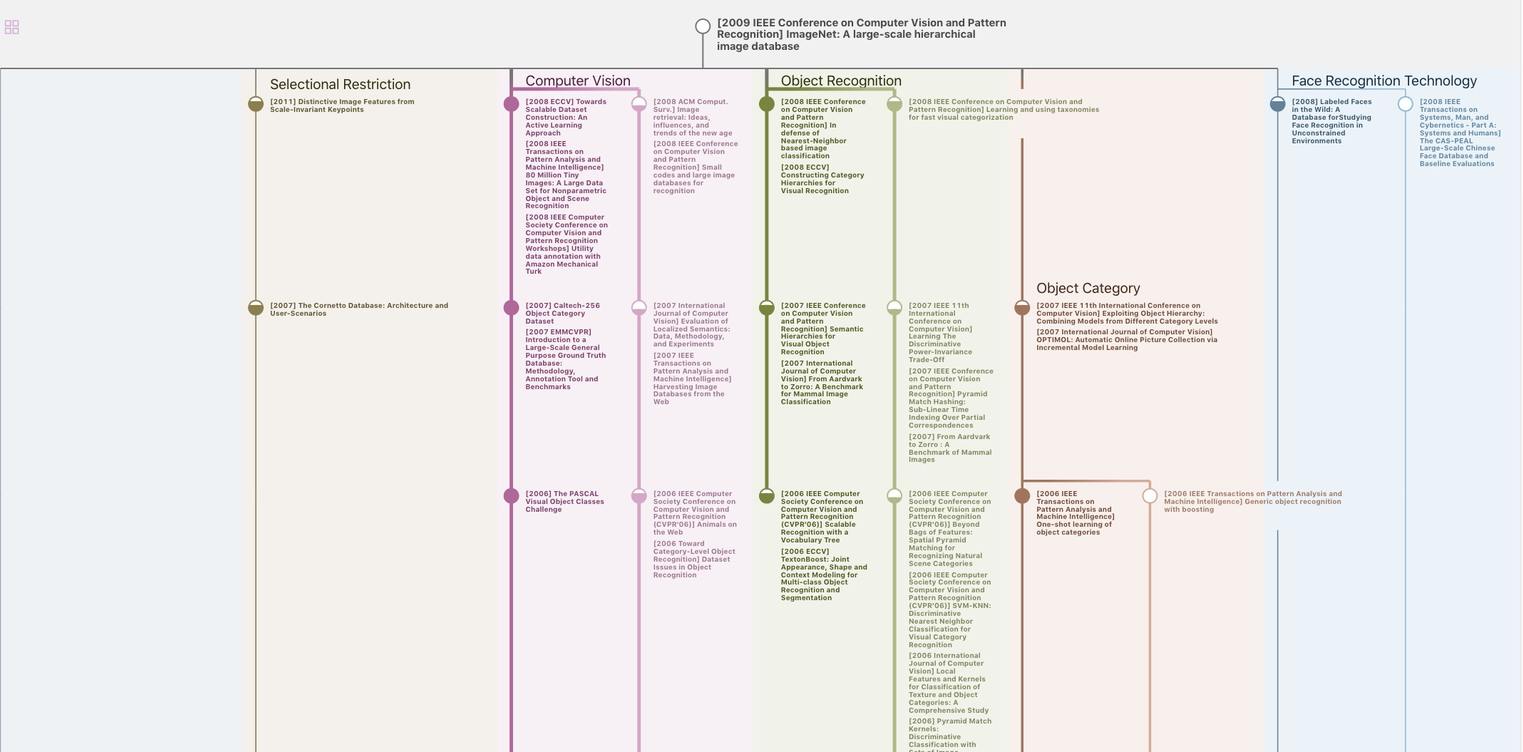
生成溯源树,研究论文发展脉络
Chat Paper
正在生成论文摘要