Adaptive weighted total variation expansion and Gaussian curvature guided low-dose CT image denoising network
Biomedical Signal Processing and Control(2024)
摘要
The denoising task of low-dose CT images is a highly complex and uncertain inverse problem. Previous studies have primarily relied on convolutional neural network to reduce noise by learning the mapping from LDCT images to normal dose CT images. However, simply increasing the network depth alone is not an optimal choice due to the limited performance improvement and significant computational cost. In contrast, integrating prior knowledge of images with a model to assist in image reconstruction is a more efficient approach. This study proposes a new framework for denoising LDCT images, named Noise-Optimized Edge Feature Guided Network (NEFGN). The task of NEFGN is to integrate the noise optimization model of adaptive weighted total variation expansion, the edge detection model guided by Gaussian curvature, and image reconstruction into an end-to-end CNN framework. In order to achieve this goal, the noise optimization model is first constructed by learning the parameters in the adaptive weighted total variation regularization model to approximate the noise level in the NDCT image. The edge detection network is constructed using Gaussian curvature, predicting clear edges directly from the noise image. Finally, under the guidance of the noise optimization model and the edge detail model, NEFGN is more capable of suppressing artifact noise, demonstrating good accuracy and robustness, and can restore finer details. Numerous experimental studies demonstrate that the NEFGN denoising framework effectively restores the structure of LDCT images with limited image details and outperforms other methods in terms of performance.
更多查看译文
关键词
LDCT,AWTV,Image denoising,Gaussian curvature,CNN
AI 理解论文
溯源树
样例
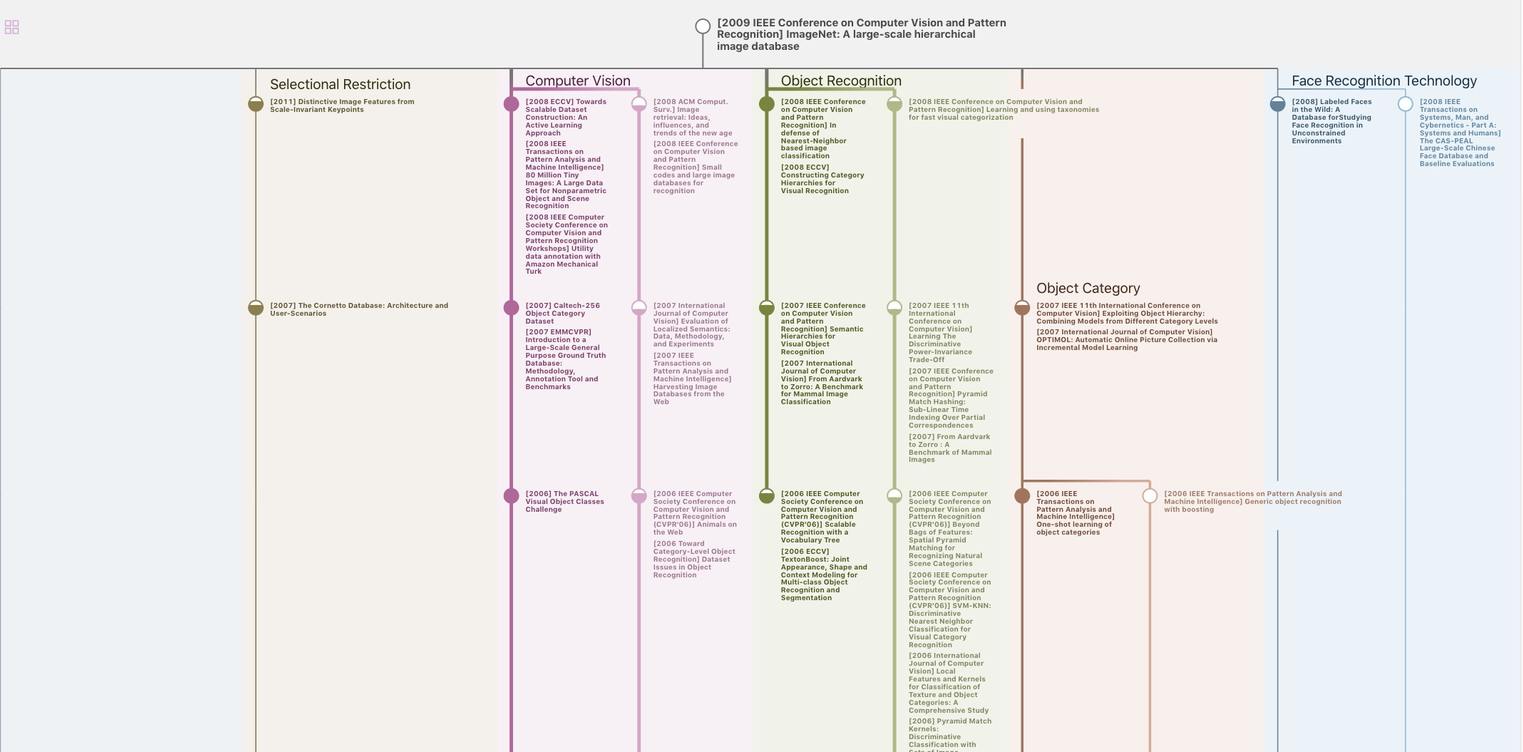
生成溯源树,研究论文发展脉络
Chat Paper
正在生成论文摘要