An Experimental Study on EUV-To-Magnetogram Image Translation Using Conditional Generative Adversarial Networks
EARTH AND SPACE SCIENCE(2024)
摘要
Deep generative models have recently become popular in heliophysics for their capacity to fill in gaps in solar observational data sets, thereby helping mitigating the data scarcity issue faced in space weather forecasting. A particular type of deep generative models, called conditional Generative Adversarial Networks (cGAN), has been used since a few years in the context of image-to-image (I2I) translation on solar observations. These algorithms have however hyperparameters whose values might influence the quality of the synthetic image. In this work, we use magnetograms produced by the Helioseismic and Magnetic Imager (HMI) on board the Solar Dynamics Observatory (SDO) and EUV images from the Atmospheric Imaging Assembly (AIA) for the problem of generating Artificial Intelligence (AI) synthetic magnetograms from multiple SDO/AIA channels using cGAN, and more precisely the Pix2PixCC algorithm. We perform a systematic study of the most important hyperparameters to investigate which hyperparameter might generate magnetograms of highest quality with respect to the Structural Similarity Index. We propose a structured way to perform training with various hyperparameter values, and provide diagnostic and visualization tools of the generated versus targeted image. Our results shows that when using a larger number of filters in the convolution blocks of the cGAN, the fine details in the generated magnetogram are better reconstructed. Adding several input channels besides the 304 angstrom channel does not improve the quality of generated magnetogram, but the hyperparameters controlling the relative importance of the different loss functions in the optimization process have an influence on the quality of the results. Performance of space weather forecasting methods relies on the availability of data to be ingested in physical models, which is scarce in space weather as compared to terrestrial weather. Since a few years, deep learning methods have produced algorithms capable of performing image-to-image-translation that are used in solar physics to mitigate this scarcity issue. In this work, we consider a deep generative model called the Pix2PixCC algorithm, which is based on conditional Generative Adversarial Networks. One needs to fix some hyperparameters in the Pix2PixCC algorithm, such as for example, the number of filters used to build the features that characterize the information contained in the data. Our aim in this paper is to make an extensive study of these hyperparameters. We propose a structured way to perform training with various hyperparameter values and provide diagnostic and visualization tools of the generated versus real image. Our results show that a higher number of filters will improve the quality of the synthetic magnetogram and that the respective contribution of each loss function in the global objective function to be minimized also has an influence on the quality of the result. We study an image to image translation algorithm that generates solar vector magnetograms from multichannel extreme ultraviolet observations We provide insight into the architecture and optimization of the Pix2PixCC algorithm, a conditional Generative Adversarial Network Our experimental design highlights relevant hyperparameters, and our interactive tool analyses the quality of the generated magnetograms
更多查看译文
AI 理解论文
溯源树
样例
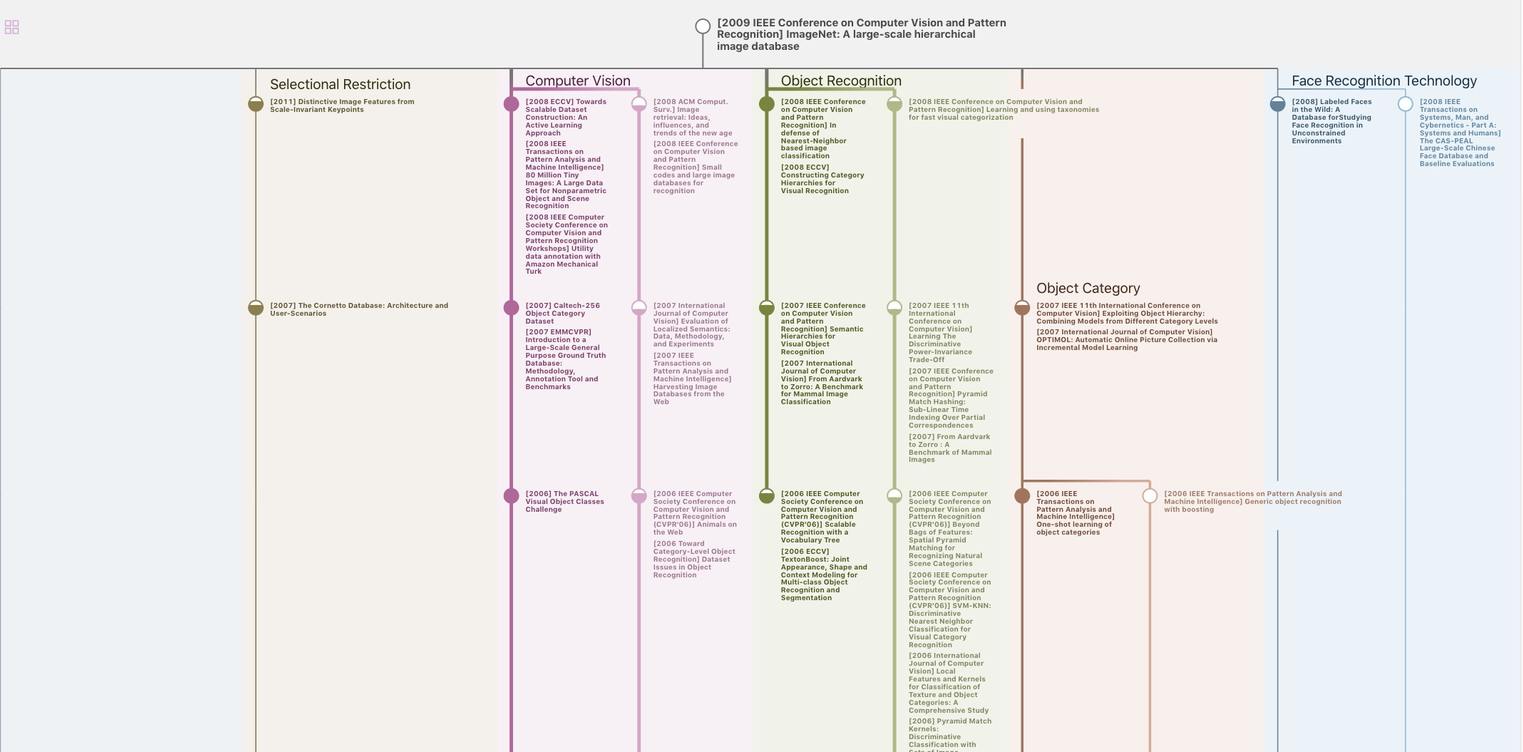
生成溯源树,研究论文发展脉络
Chat Paper
正在生成论文摘要