Radar Waveform Design based on Target Pattern Separability via Fractional Programming
IEEE Transactions on Signal Processing(2024)
摘要
Separability is an important criterion for measuring the degree of overlap between different features in pattern recognition. Stronger separability indicates clearer feature margins, facilitating classification of samples into their respective classes. Therefore, if the separability of radar target echoes within the feature space increases, the likelihood of correct classification increases. A waveform with enhanced separability offers more reliable discrimination for specific targets and highlights their unique aspects. Accordingly, this paper proposes radar waveform design methods driven by target echo pattern separability, i.e., the convolution of the target high-resolution range profiles (HRRP)/target impulse response (TIR) and transmit waveform. First, from the views of local HRRP/TIR manifold structure preservation and inter-class distance enlargement, we construct a minmax based fractional optimization model with non-convex and non-linear orthonormality and constant modulus (CM) constraints. Then, the derived waveform design solution is computed iteratively via subproblem division, fraction simplification, and high-order polynomial optimization. Furthermore, we extend our study to a linear discriminant analysis-based waveform design model, which incorporates minmax intra-class distance and inter-class distance metrics to maximize separability of all classes from a worst-case perspective. We utilize simulated datasets based on the scattering point model as well as electromagnetic simulation and MSTAR CSV datasets to evaluate the performance of our waveform design approaches.
更多查看译文
关键词
Radar waveform design,Fractional programming,Pattern separability
AI 理解论文
溯源树
样例
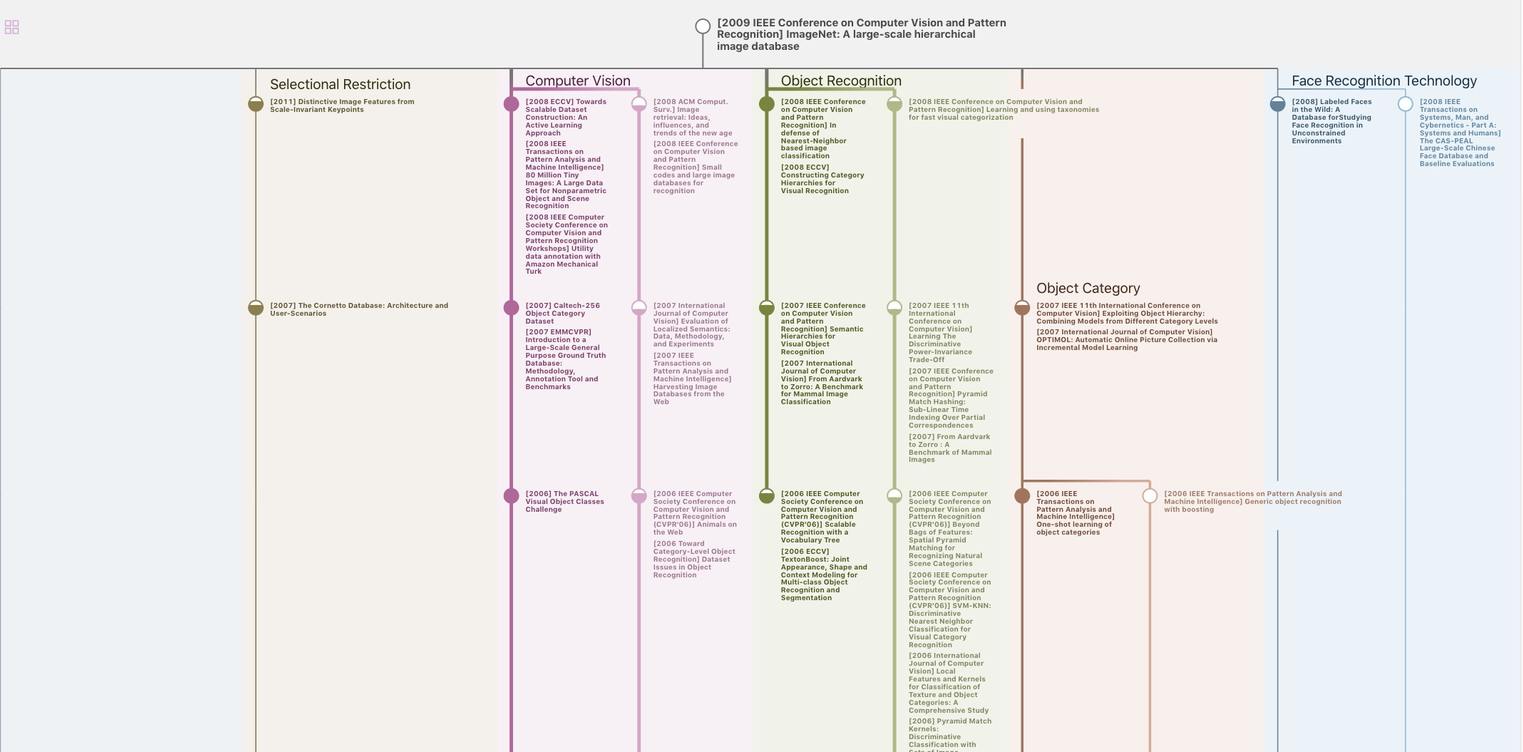
生成溯源树,研究论文发展脉络
Chat Paper
正在生成论文摘要