Multimedia Data and Security.
IEEE Multim.(2024)
摘要
The detection of manipulation of multimedia data can be categorized as active and passive approaches.1 The active approaches use source information, such as watermarks and digital signatures. For instance, Bahirat et al.2 proposed a watermarking-based framework for authentication and localization of tampering in red, green, blue (RGB) and the 3-D point cloud. The watermarking methods, which are computationally expensive, cannot be applied on raw unprocessed data. Passive approaches, or blind forensics,3 are intended for testing multimedia data where the original or source information is not available. Deep-learning-based tampering detection avoids the need to perform various forensic tests to detect whether multimedia data have been manipulated or not.4 However, deep-learning-based methodologies need to balance false positives and negatives, as pointed out by Bayar and Stamm.4 Apart from the need for detecting manipulations in multimedia data, there is also a need for localizing (i.e., identifying the region) where the manipulation occurred.
更多查看译文
关键词
Multimedia Data,Source Of Information,Convolutional Neural Network,Deep Network,Forensic,Feature Learning,Transfer Learning,Point Cloud,Conditional Random Field,Passive Approach,Red-green-blue,Digital Signature,JPEG Images
AI 理解论文
溯源树
样例
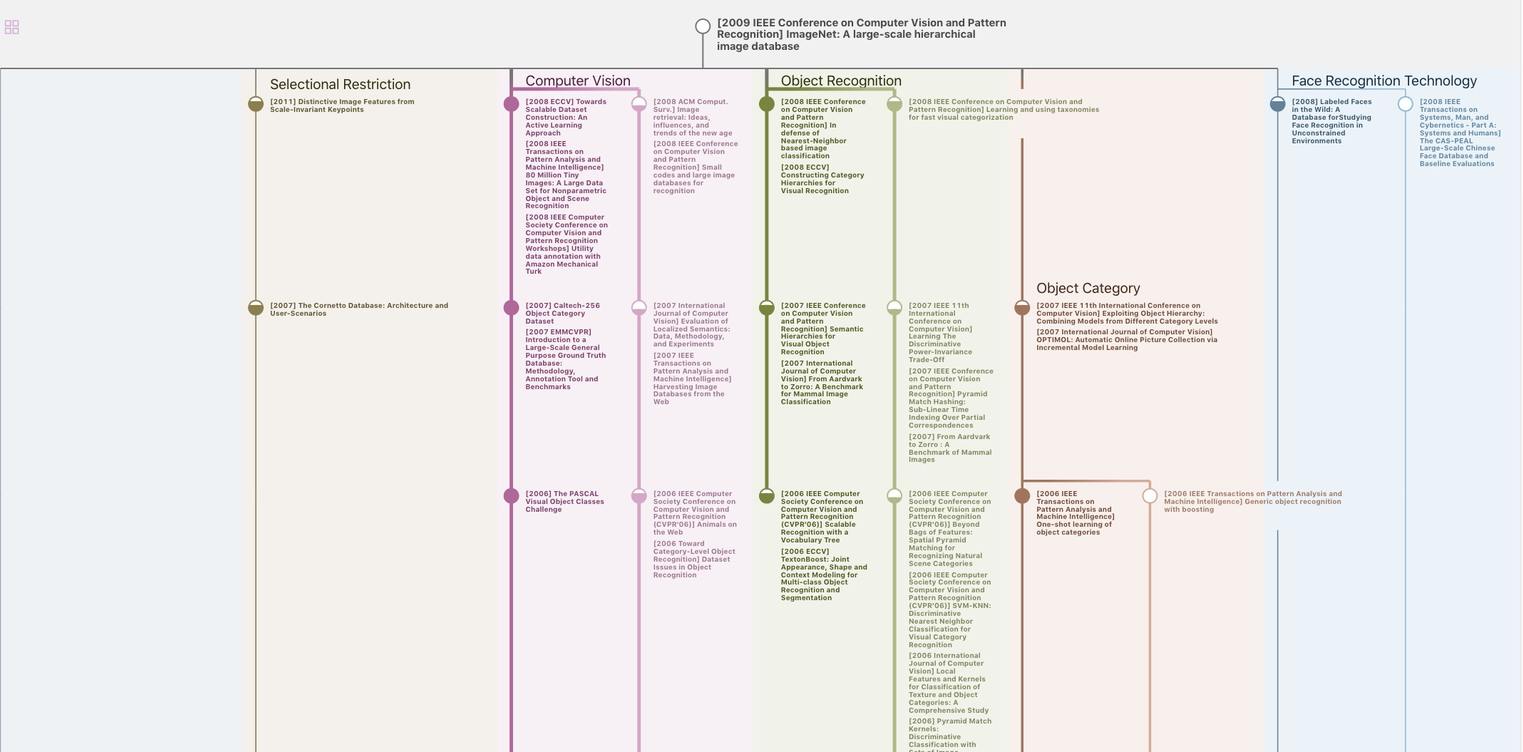
生成溯源树,研究论文发展脉络
Chat Paper
正在生成论文摘要