A Circumstance-aware Neural Framework for Explainable Legal Judgment Prediction
IEEE Transactions on Knowledge and Data Engineering(2024)
摘要
Massive legal documents have promoted the application of legal intelligence. Among them, Legal Judgment Prediction (LJP) has emerged as a critical task, garnering significant attention. LJP aims to predict judgment results for multiple subtasks, including charges, law articles, and terms of penalty. Existing studies primarily focus on utilizing the entire factual description to produce judgment results, overlooking the practical judicial scenario where judges consider various crime circumstances to decide verdicts and sentencing. To this end, in this paper, we propose a circumstance-aware LJP framework (i.e., NeurJudge) by exploring the circumstances of crime. Specifically, NeurJudge first separates the factual description into different circumstances with the predicted results of intermediate subtasks and then employs them to yield results of other subtasks. Besides, as confusing verdicts may degrade the performance of LJP, we further develop a variant of NeurJudge (NeurJudge+) that incorporates the semantics of labels (charges and law articles) into facts to yield more expressive and distinguishable fact representations. Finally, to provide explanations for LJP, we extend NeurJudge to an explainable LJP framework E-NeurJudge with a cooperative teacher-student system. The teacher system is NeurJudge which exploits legal particularities well but lacks explanation capability. The student system is a rationalization method that provides explainability but fails to utilize legal particularities. To combine the advantages of the above methods, we use a transferring function to transfer legal particularities from the teacher to the student, making a trade-off between yielding LJP results and rendering them explainable. Extensive experimental results on real-world datasets validate the effectiveness of our proposed frameworks.
更多查看译文
关键词
Explainable legal document mining,text classification,label embedding,fact separation,rationalization
AI 理解论文
溯源树
样例
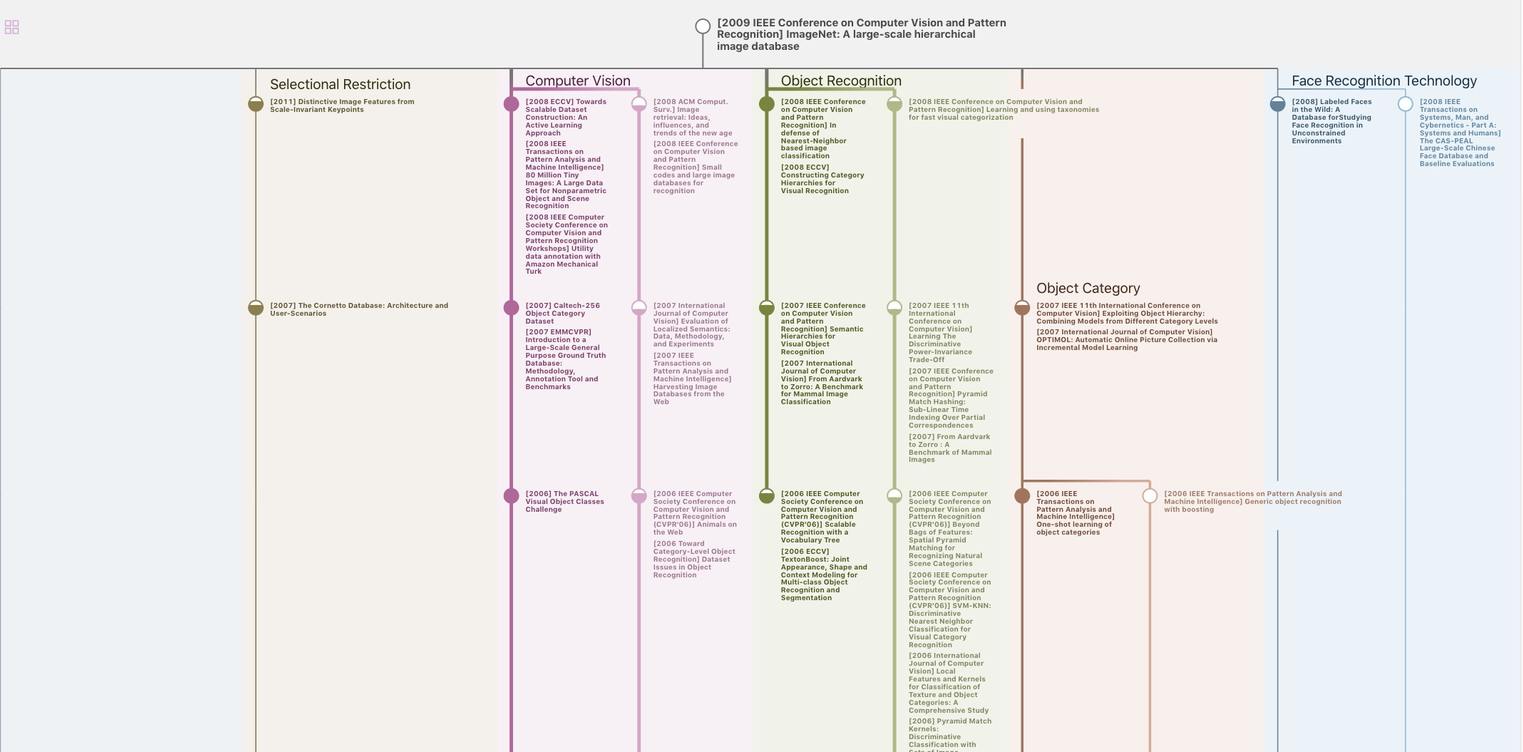
生成溯源树,研究论文发展脉络
Chat Paper
正在生成论文摘要