An SGX and Blockchain-Based System for Federated Learning in Classifying COVID-19 Images.
Parallel and Distributed Processing with Applications(2023)
摘要
Federated learning, an emerging distributed learning framework, has gained prominence recently. However, two challenges arise when applying federated learning to medical data. The first challenge stems from the inability of model-generating nodes to ensure computational fairness. The second challenge involves the potential leakage of private information through gradient differences. This paper introduces a federated learning system that leverages Software Guard Extensions (SGX) and blockchain technology to enhance the fairness and security of federated learning for analyzing chest imaging data. The modeling of imaging factors for chest radiography data can effectively regulate the data quality of semi-trusted nodes. A blockchain smart contract is employed for data quality-aware proof, and the quality-aware block undergoes a two-stage rewriting process across distinct nodes. A notary membership model achieves notarized cluster consensus for imaging informatics data. The multi-channel layer model quantifies the distribution of computational workload across participating nodes within the medical consortium. Upon successful verification of the enclave command in the aggregation cycle, traceable and packaged signatures are generated to support computational fairness. To ensure robust information security, SGX remote authentication is used between downstream and upstream nodes. The federated learning system, built upon SGX and blockchain, has been implemented using the Fabric federated chain platform. The ResNet model underwent training through cross-sectional federated learning to effectively classify novel coronavirus lung medical image data. Following this, multiple experimental control and security analyses were conducted, revealing that the time loss incurred due to crossing channels is approximately 21.31%, while the time overhead of SGX remains within 5.2%, as allowed by the number of nodes.
更多查看译文
关键词
Medical Imaging,SGX,Blockchain,Federated Learning,Affiliate Chain
AI 理解论文
溯源树
样例
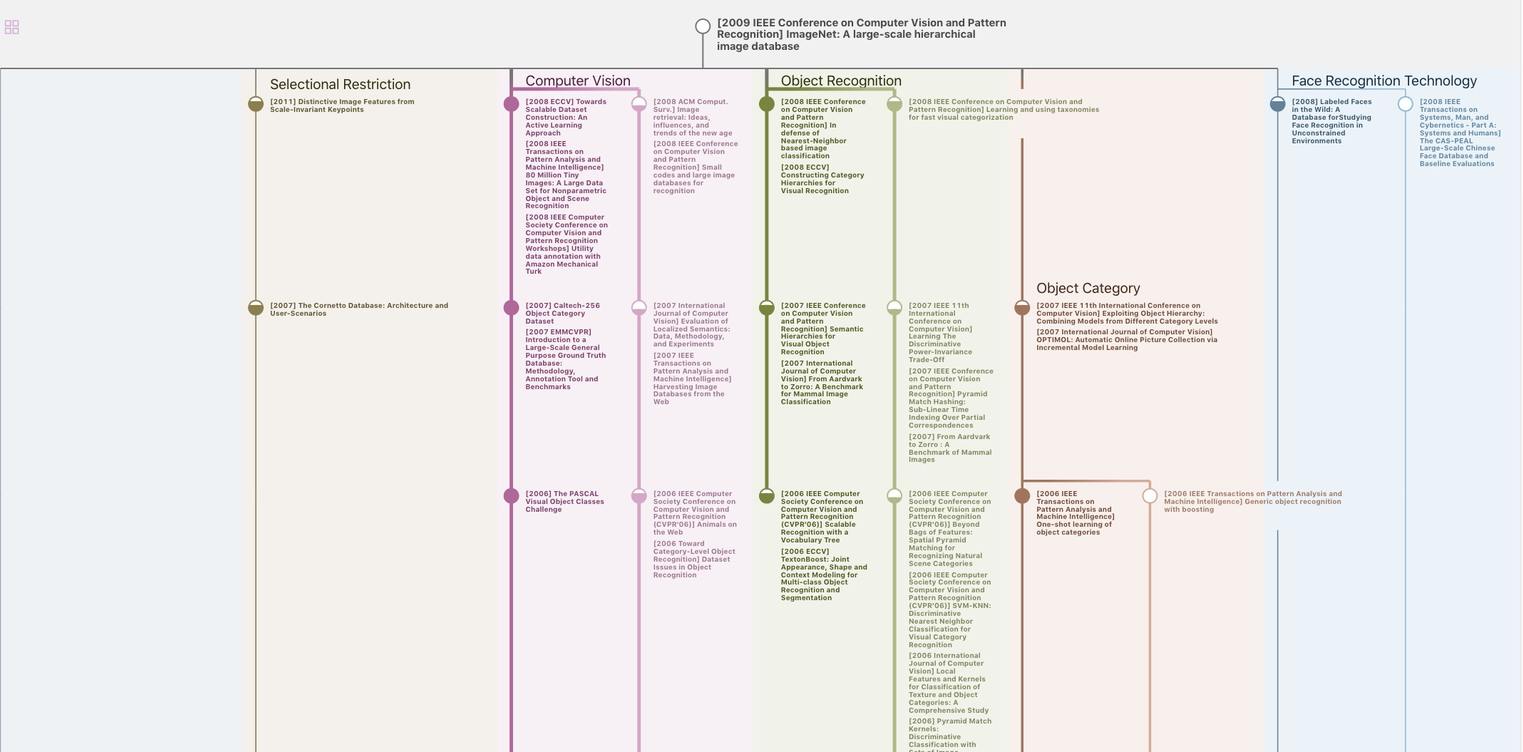
生成溯源树,研究论文发展脉络
Chat Paper
正在生成论文摘要