Incomplete Graph Learning via Partial Graph Convolutional Network
IEEE Transactions on Artificial Intelligence(2024)
摘要
Graph Convolutional Networks (GCNs) gain increasing attention on graph data learning tasks in recent years. However, in many applications, graph may come with an incomplete form where attributes of graph nodes are partially unknown/missing. Existing Graph Convolutions (GCs) are generally designed on complete graphs which can not deal with attribute-incomplete graph data directly. To address this problem, in this paper, we extend standard GC and develop an explicit Partial Graph Convolution (PaGC) for attribute-incomplete graph data. Our PaGC is derived based on the observation that the core neighborhood aggregator in GC operation can be equivalently viewed as an energy minimization model. Based on it, we can define a novel
partial aggregation function
and derive PaGC for incomplete graph data. Experiments demonstrate the effectiveness and efficiency of the proposed PaGCN.
更多查看译文
关键词
Graph convolutional network,Partial aggregation,Attribute-incomplete graph,Dropout,Semi-supervised learning
AI 理解论文
溯源树
样例
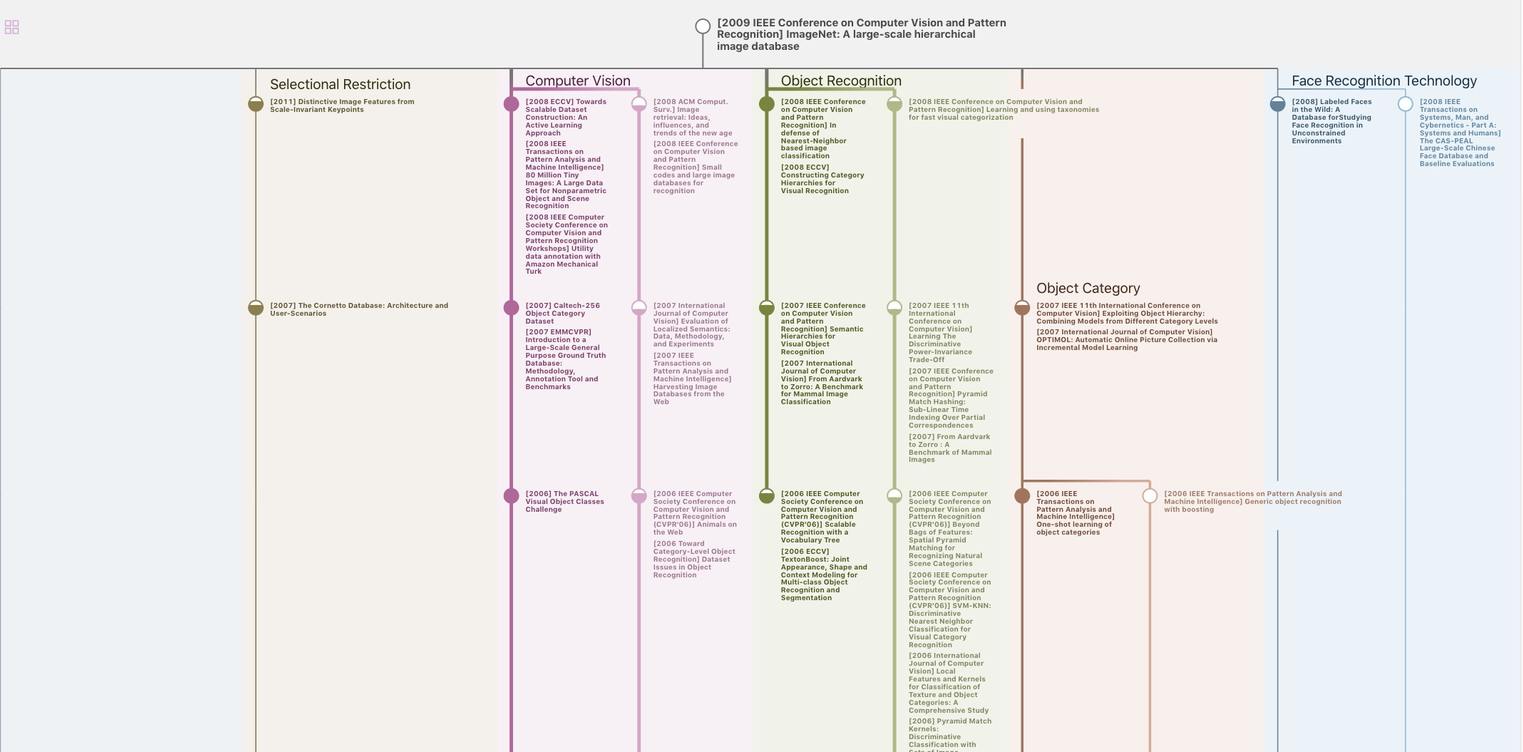
生成溯源树,研究论文发展脉络
Chat Paper
正在生成论文摘要