Weather and Meteorological Optical Range Classification for Autonomous Driving
IEEE Transactions on Intelligent Vehicles(2024)
摘要
Weather and meteorological optical range (MOR) perception is crucial for smooth and safe autonomous driving (AD). This article introduces two deep learning-based architectures, employing early and intermediate sensor fusion and multi-task strategies, designed for concurrent weather and MOR classification in AD. Extensive experiments employing the publicly available FogChamber dataset demonstrate that the proposed early fusion architecture, characterized by its lightweight design and simplicity, achieves an accuracy of 98.88% in weather classification and 89.77% in MOR classification, with a competitive memory allocation of 5.33 megabytes (MB) and an inference time of 2.50 milliseconds (ms). In contrast, the proposed intermediate fusion architecture prioritizes performance, achieving higher accuracies of 99.38% in weather classification and 91.88% in MOR classification. However, it requires a more substantial memory allocation of 54.06 MB and exhibits a longer inference time of 15.55 ms. Compared to other state-of-the-art architectures, the proposed methods present a competitive balance between accuracy performance, inference time, and memory allocation, which are crucial parameters for enabling autonomous driving. The code presented in this research is available at
https://github.com/CelsoPereira1/AutoWeatherPerception
.
更多查看译文
关键词
Weather classification,Meteorological optical range classification,Deep learning,Multi-task learning,Single-task learning,Multi-modal,RGB Camera,LiDAR,Image entropy
AI 理解论文
溯源树
样例
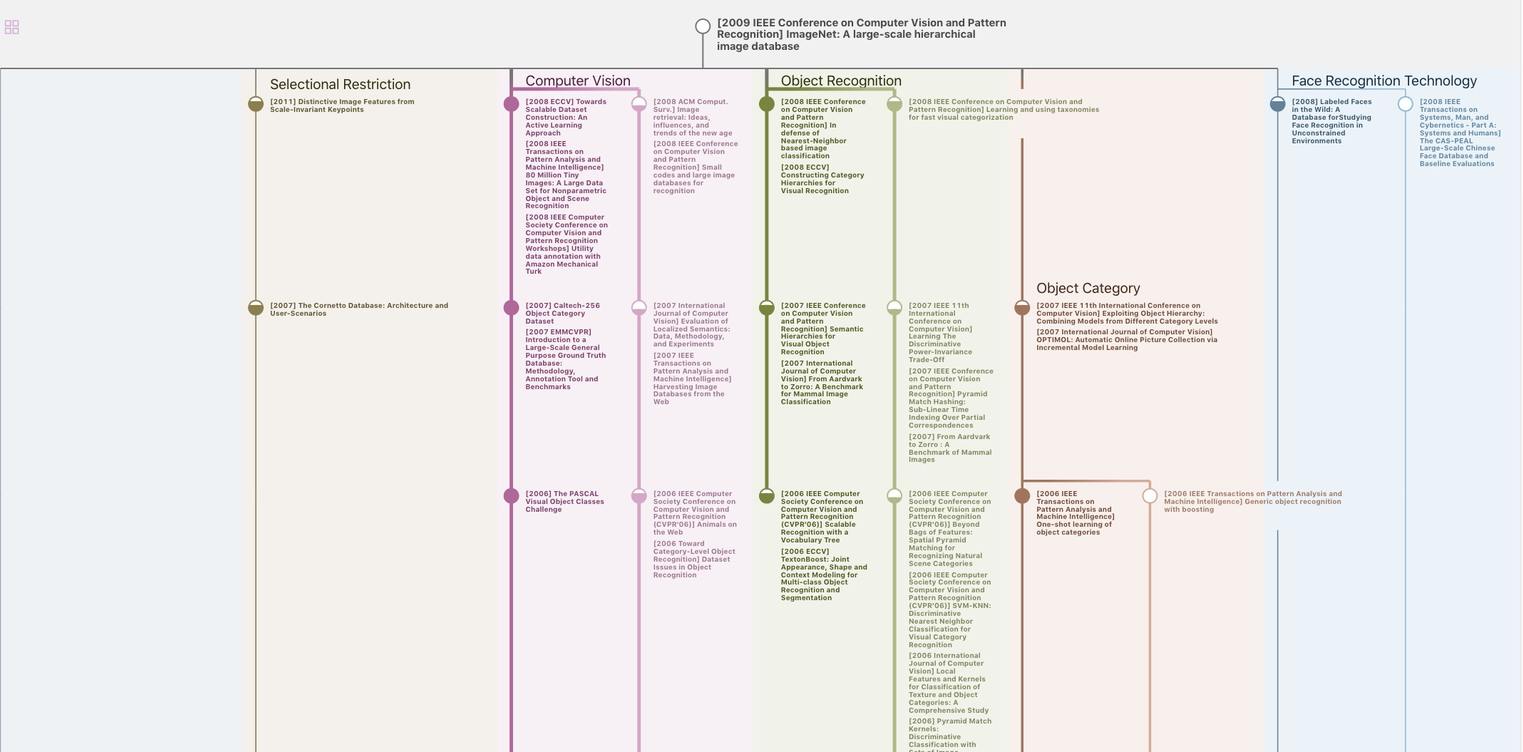
生成溯源树,研究论文发展脉络
Chat Paper
正在生成论文摘要