Adapting Neural Networks at Runtime: Current Trends in At-Runtime Optimizations for Deep Learning
ACM Computing Surveys(2024)
摘要
Adaptive optimization methods for deep learning adjust the inference task to the current circumstances at runtime to improve the resource footprint while maintaining the model’s performance. These methods are essential for the widespread adoption of deep learning, as they offer a way to reduce the resource footprint of the inference task while also having access to additional information about the current environment. This survey covers the state-of-the-art at-runtime optimization methods, provides guidance for readers to choose the best method for their specific use-case, and also highlights current research gaps in this field.
更多查看译文
AI 理解论文
溯源树
样例
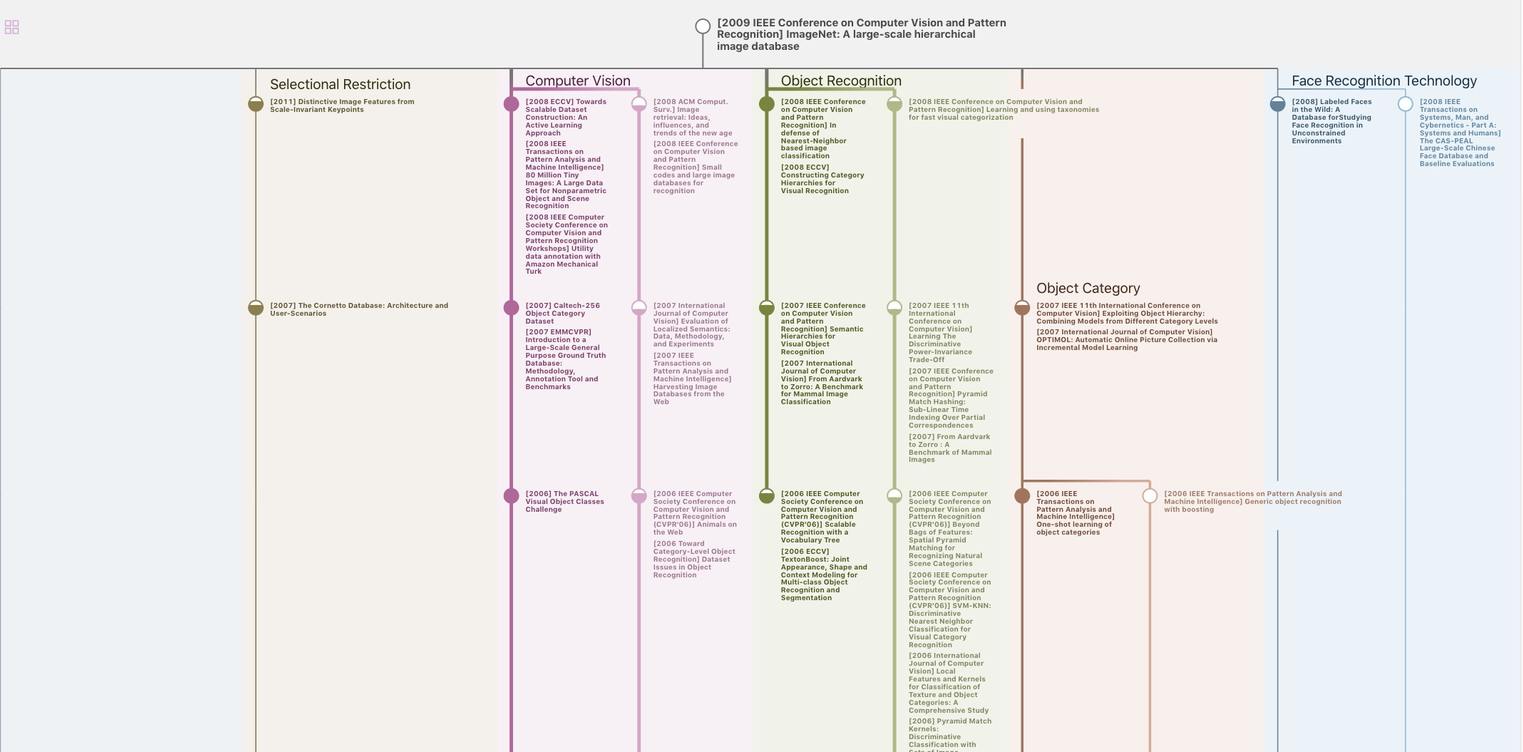
生成溯源树,研究论文发展脉络
Chat Paper
正在生成论文摘要