BayGO: Decentralized Bayesian Learning and Information-Aware Graph Optimization Framework
IEEE Transactions on Signal Processing(2024)
摘要
Multi-agent Decentralized Learning (MADL) is a scalable approach that enables agents to learn based on their local datasets. However, it presents significant challenges related to the impact of dataset heterogeneity and the communication graph structure on learning speed, as well as the lack of a robust method for quantifying prediction uncertainty. To address these challenges, we propose BayGO, a novel fully-decentralized multi-agent local
Bay
esian learning with local averaging, usually referred to as non-Bayesian social learning, together with
g
raph
o
ptimization framework. Within BayGO, agents locally learn a posterior distribution over the model parameters, updating it locally using their datasets and sharing this information with their neighbors. We derive an aggregation rule for combining received posterior distributions to achieve optimality and consensus. Moreover, we theoretically derive the convergence rate of agents’ posterior distributions. This convergence rate accounts for both network structure and information heterogeneity among agents. To expedite learning, agents employ the derived convergence rate as an objective, optimizing it with respect to the network structure alternately with their posterior distributions. As a consequence, agents can successfully fine-tune their network connections according to the information content of their neighbors. This leads to a sparse graph configuration, where each agent communicates exclusively with the neighbor that offers the highest information gain, enhancing communication efficiency. Our simulations corroborate that the BayGO framework accelerates learning compared to fully-connected and star topologies owing to its capacity for selecting neighbors based on information gain.
更多查看译文
关键词
Bayesian Learning,Distributed Learning,Social Learning,Graph Optimization,Information Heterogeneity,KL Divergence,Multi-agent systems
AI 理解论文
溯源树
样例
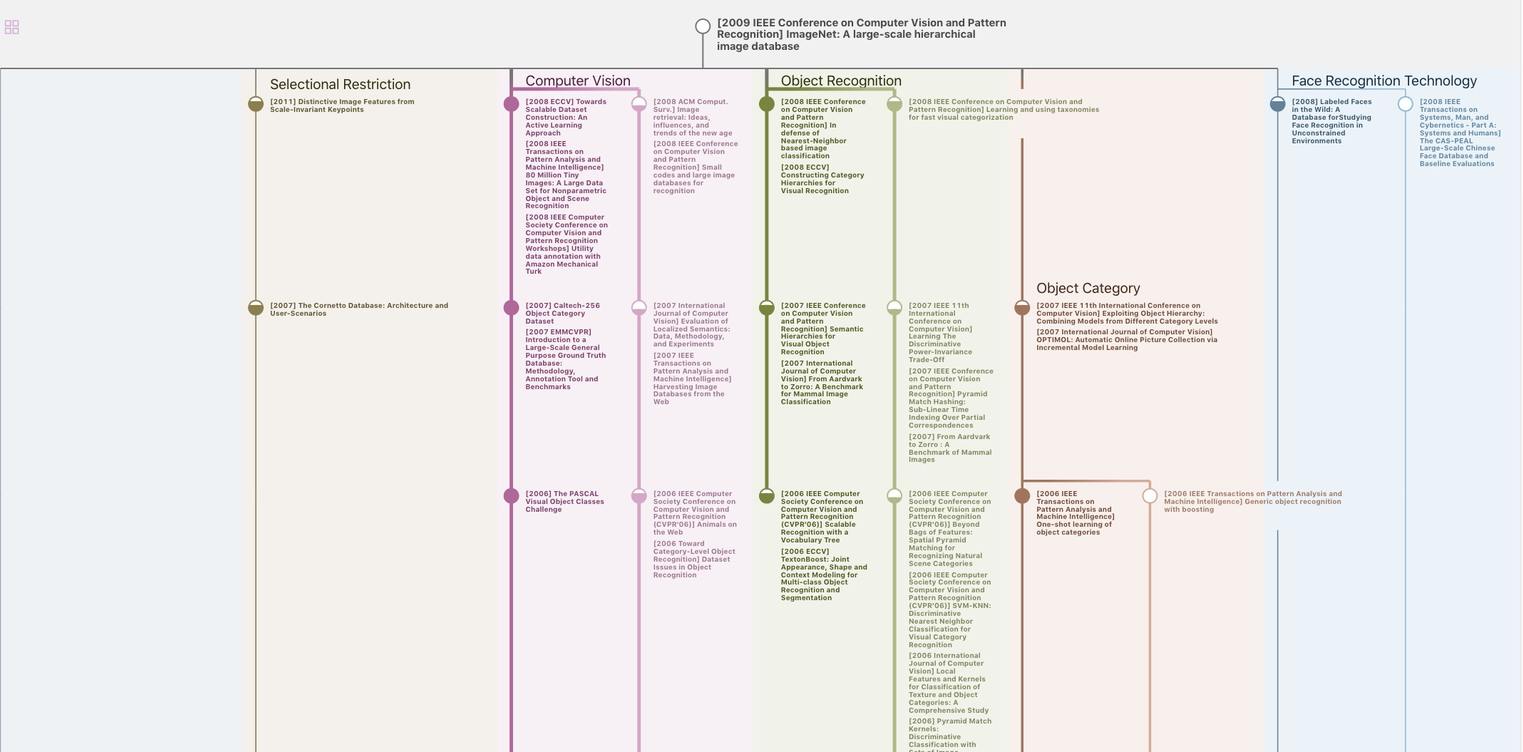
生成溯源树,研究论文发展脉络
Chat Paper
正在生成论文摘要