Prediction of Specific Surface Area of Metal-Organic Frameworks by Graph Kernels
2023 Congress in Computer Science, Computer Engineering, & Applied Computing (CSCE)(2023)
摘要
Metal-organic frameworks (MOFs) are coordination networks of metal ions or clusters bonded by organic ligands. They are expected to have a wide range of applications due to their tunable micro-porosity and unprecedented surface area. However, the diverse combinations of metals and organic ligands make it difficult to identify optimal combinations. This research aims to propose a machine learning-based method for searching candidates of optimal combinations without physically synthesizing MOFs, thus improving the effectiveness and efficiency of MOF development. To incorporate the structural features of organic ligands into the investigation, this paper utilizes graph kernels. Graphs of atoms are used to faithfully represent the structural features, and graph kernels evaluate the similarity between these graphs. Additionally, a unified kernel is defined to assess the similarity between MOFs. This unified kernel consists of a weighted sum of an RBF kernel to evaluate the similarity of metals and a normalized graph kernel to evaluate the similarity of organic ligands. Using this unified kernel and the support vector machine classification algorithm, a model is developed to predict whether a given combination of a metal and an organic ligand can result in an MOF with a high specific surface area. Through experiments using MOF data from the CoRE MOF dataset, it has been verified that the models constructed with six out of the seven tested graph kernels demonstrate good prediction performance in terms of the AUC-ROC score.
更多查看译文
关键词
graph kernel,metal-organic framework
AI 理解论文
溯源树
样例
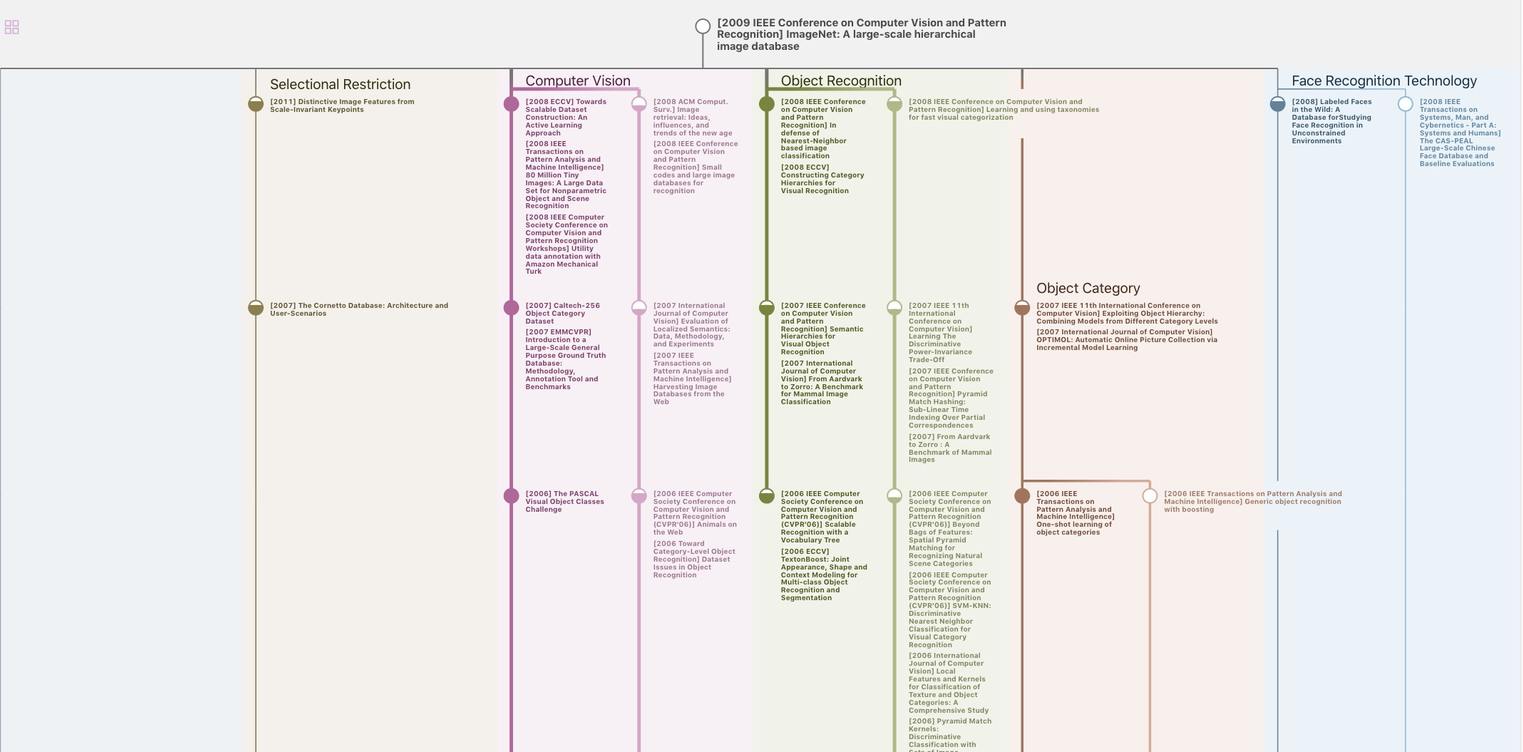
生成溯源树,研究论文发展脉络
Chat Paper
正在生成论文摘要