Revisiting Latent Space of GAN Inversion for Robust Real Image Editing.
IEEE/CVF Winter Conference on Applications of Computer Vision(2024)
摘要
We present a generative adversarial network (GAN) inversion with high reconstruction and editing quality. GAN inversion algorithms with expressive latent spaces produce near-perfect inversion but are not robust to editing operations in a latent space, leading to undesirable edited images, a phenomenon known as the trade-off between reconstruction and editing quality. To cope with the trade-off, we revisit the hyperspherical prior of StyleGANs $\mathcal{Z}$ and propose to combine an extended space of $\mathcal{Z}$ with highly capable inversion algorithms. Our approach maintains the reconstruction quality of seminal GAN inversion methods while improving their editing quality owing to the constrained nature of $\mathcal{Z}$. Through comprehensive experiments with several GAN inversion algorithms, we demonstrate that our approach enhances the image editing quality in 2D/3D GANs.
1
更多查看译文
关键词
Algorithms,Generative models for image,video,3D,etc.,Algorithms,Biometrics,face,gesture,body pose,Applications,Arts / games / social media
AI 理解论文
溯源树
样例
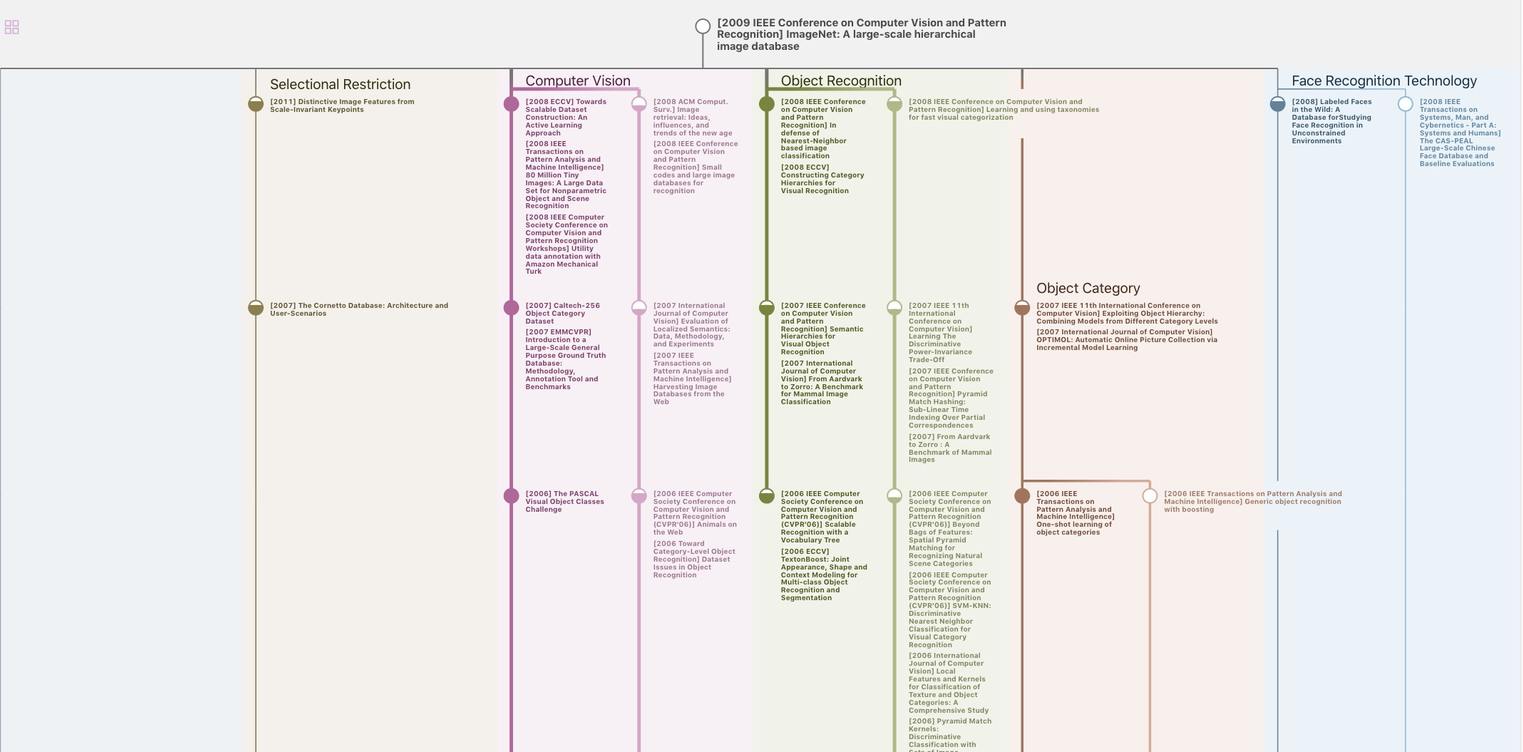
生成溯源树,研究论文发展脉络
Chat Paper
正在生成论文摘要