Controllable Text-to-Image Synthesis for Multi-Modality MR Images.
IEEE/CVF Winter Conference on Applications of Computer Vision(2024)
摘要
Generative modeling has seen significant advancements in recent years, especially in the realm of text-to-image synthesis. Despite this progress, the medical field has yet to fully leverage the capabilities of large-scale foundational models for synthetic data generation. This paper introduces a framework for text-conditional magnetic resonance (MR) imaging generation, addressing the complexities associated with multi-modality considerations. The framework comprises a pre-trained large language model, a diffusion-based prompt-conditional image generation architecture, and an additional denoising network for input structural binary masks. Experimental results demonstrate that the proposed framework is capable of generating realistic, high-resolution, and high-fidelity multi-modal MR images that align with medical language text prompts. Further, the study interprets the cross-attention maps of the generated results based on text-conditional statements. The contributions of this research lay a robust foundation for future studies in text-conditional medical image generation and hold significant promise for accelerating advancements in medical imaging research.
更多查看译文
关键词
Applications,Biomedical / healthcare / medicine,Algorithms,Vision + language and/or other modalities
AI 理解论文
溯源树
样例
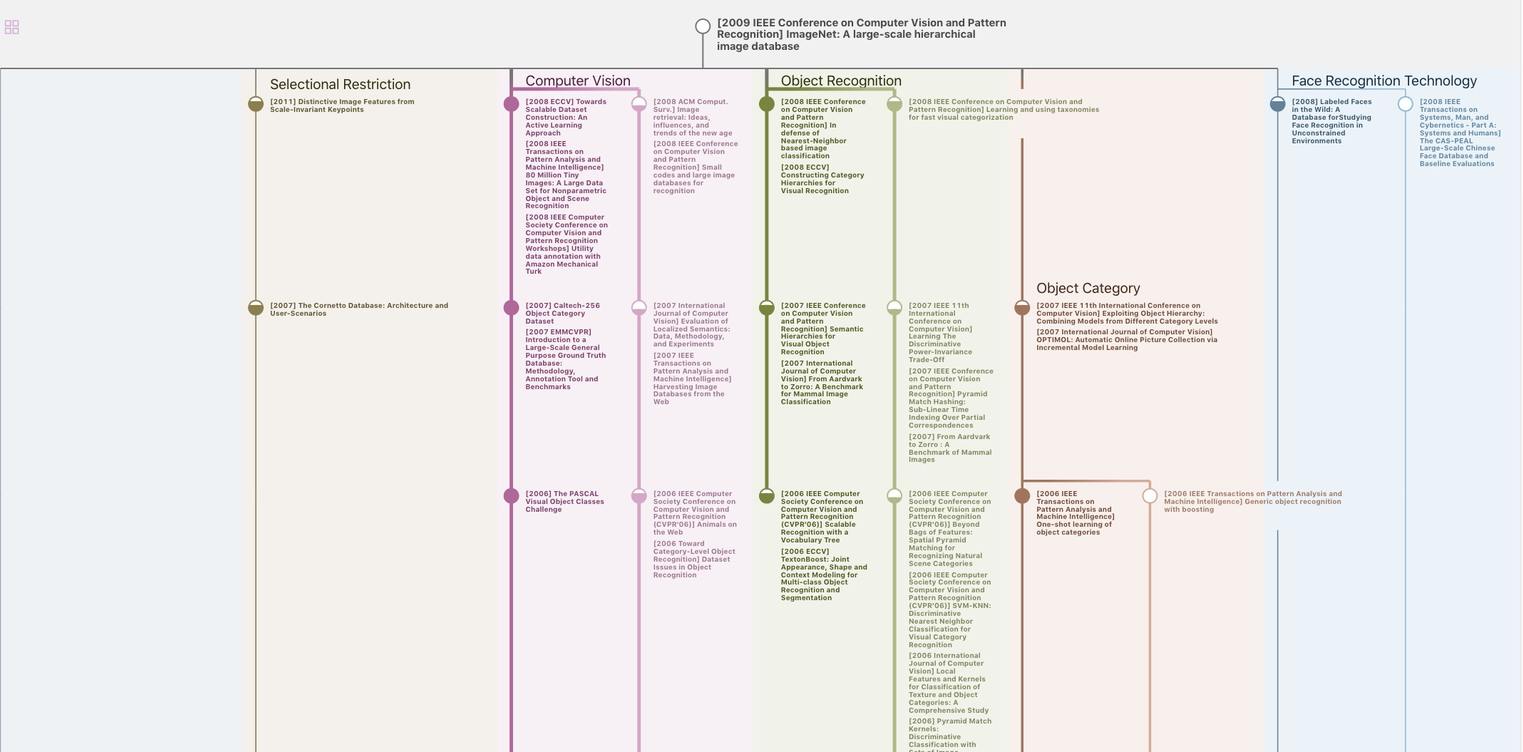
生成溯源树,研究论文发展脉络
Chat Paper
正在生成论文摘要