Multi-task Dual-Graph Network framework for Aspect Sentiment Triplet Extraction
crossref(2024)
摘要
Abstract Aspect Sentiment Triplet Extraction is a task that extracts aspects, opinion words corresponding to aspects and sentiment polarity from review sentences. Although aspect emotion triple extraction technology has achieved remarkable results in recent years, it still has a few drawbacks. Many existing research methods ignore important issues, such as the dependency relationships between emotional triad elements and the detection of aspect and viewpoint boundary terms at the phrase-level. Due to the above problems, this paper proposes a multi-task joint learning architecture based on dual graph networks (DGNMT). Firstly, a shared sentence encoder module is proposed in the model. The middle-layer and top-level feature representations of the BERT encoder are applied to two different subtasks in this module. Secondly, Dual-Graph Network Learning module has been designed. As part of the dual-graph network learning layer, this module introduces a graph attention network based on phrase structure trees in the joint extraction task branch. For the triple extraction task branch, a graph convolution network is used based on the modified syntactic dependency graph in order to determine the syntactic relationships between triple elements. Additionally , the Multi-Task Tagging module is proposed to extract labels for tasks as well as triplet extraction tasks. And then, predictions are made based on 1D sequence tags and 2D word pair matrices, and the tag information of the two tasks is transformed into each other through a conversion strategy. According to the final experimental results, the model outperforms a state-of-the-art baseline model on four commonly used evaluation indicators.
更多查看译文
AI 理解论文
溯源树
样例
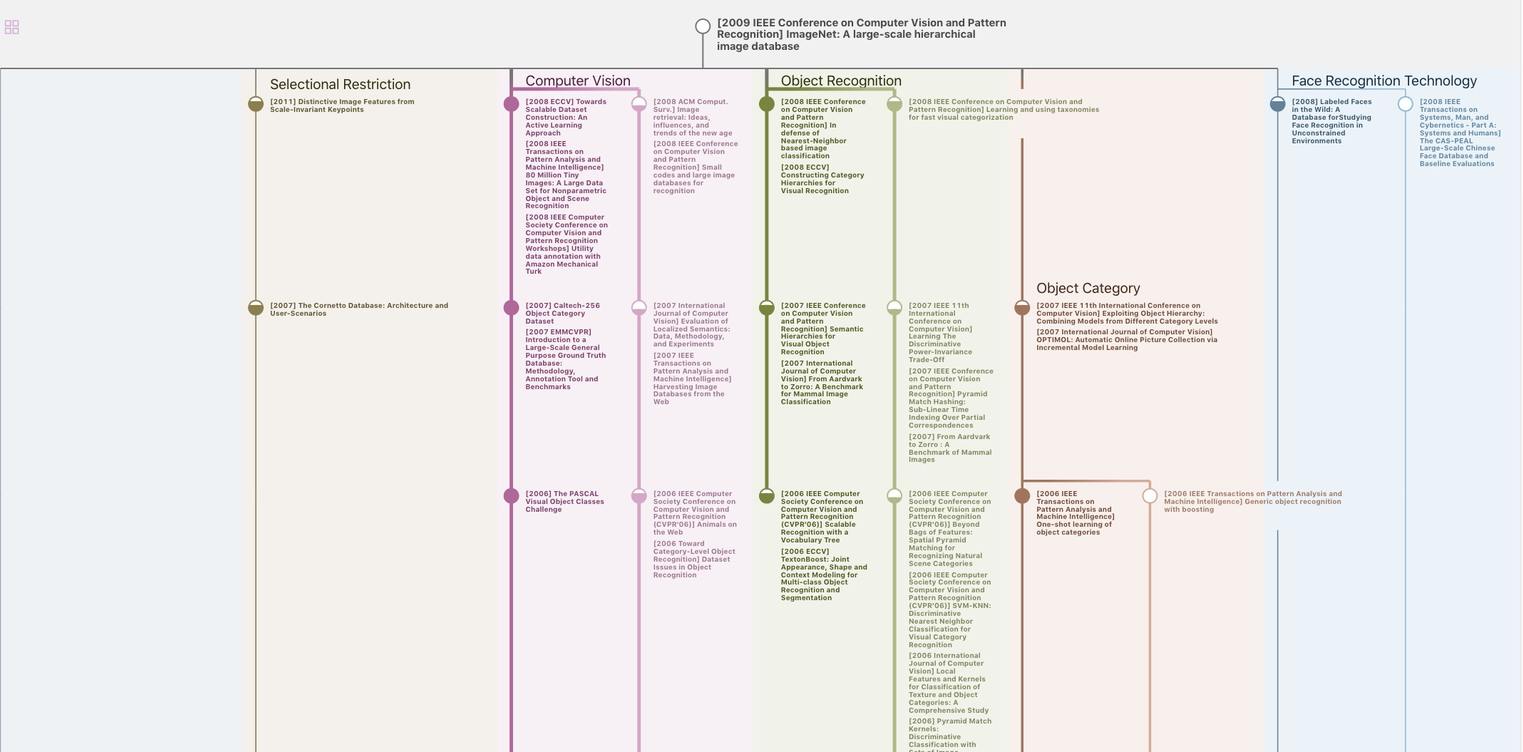
生成溯源树,研究论文发展脉络
Chat Paper
正在生成论文摘要