Routing and Charging Scheduling for EV Battery Swapping Systems: Hypergraph-Based Heterogeneous Multiagent Deep Reinforcement Learning
IEEE Transactions on Smart Grid(2024)
摘要
This work studies the joint electric vehicle (EV) routing and battery charging scheduling problem in a transportation network with multiple battery swapping stations (BSSs) under random EV swapping demands, renewable generation, and electricity prices. The joint scheduling problem is formulated as a decentralized partially observable Markov decision process (Dec-POMDP) with an objective to minimize the expected sum of the battery charging cost and the travel/waiting cost of EV owners. The formulated Dec-POMDP is hard to solve, due the curse of dimensionality and the unknown system dynamics. To tackle the challenges, we propose a new heterogeneous multiagent hypergraph attention actor-critic (HMA-HGAAC) framework, which integrates hypergraph attention (HGAT) networks to multiagent deep reinforcement learning (MADRL) to enhance the learning efficiency with a hypergraph where multiple nodes can be connected by a single hyperedge. Numerical experiments based on real-world data and a 180-node transportation network show that the proposed approach can save the system cost achieved by state-of-the-art benchmarks, independent proximal policy optimization (IPPO), multiagent proximal policy optimization (MAPPO), and heterogeneous multiagent graph attention proximal policy optimization (HMA-GAPPO), by 23.5%, 18.9%, and 13.3%, respectively.
更多查看译文
关键词
Electric vehicles,battery swapping stations,decentralized partially observable Markov decision process,multiagent deep reinforcement learning,hypergraph neural networks
AI 理解论文
溯源树
样例
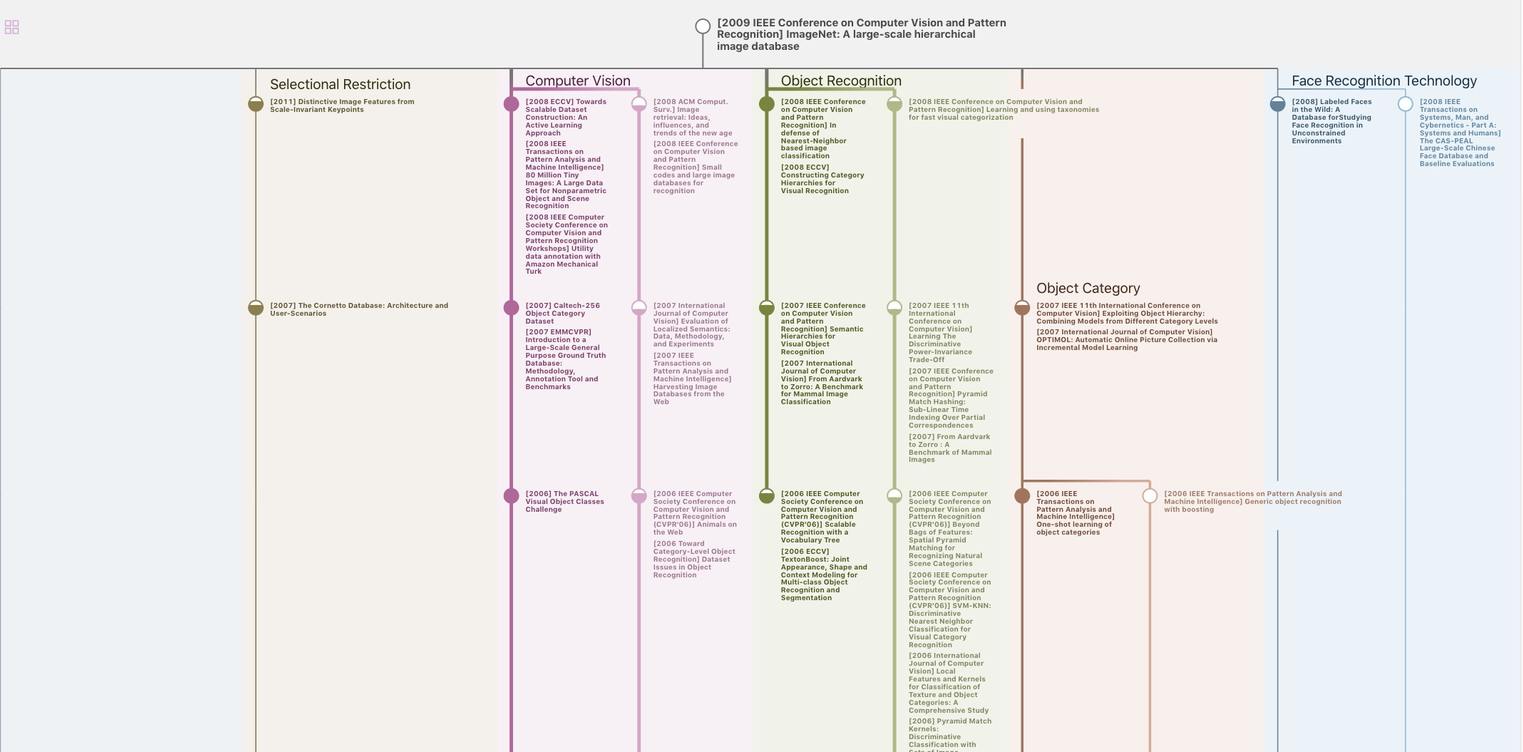
生成溯源树,研究论文发展脉络
Chat Paper
正在生成论文摘要